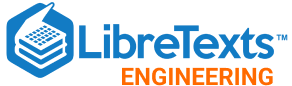
- school Campus Bookshelves
- menu_book Bookshelves
- perm_media Learning Objects
- login Login
- how_to_reg Request Instructor Account
- hub Instructor Commons
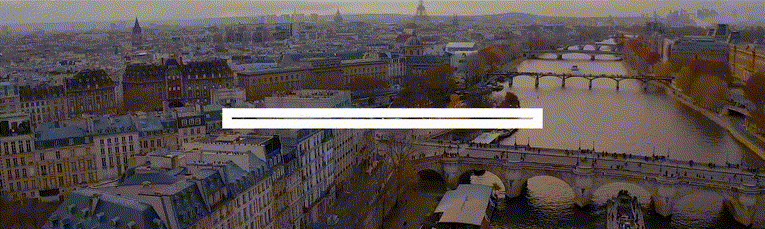
Margin Size
- Download Page (PDF)
- Download Full Book (PDF)
- Periodic Table
- Physics Constants
- Scientific Calculator
- Reference & Cite
- Tools expand_more
- Readability
selected template will load here
This action is not available.
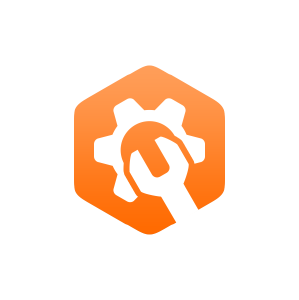
3.4: Trip Generation
- Last updated
- Save as PDF
- Page ID 47326
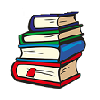
- David Levinson et al.
- Associate Professor (Engineering) via Wikipedia
\( \newcommand{\vecs}[1]{\overset { \scriptstyle \rightharpoonup} {\mathbf{#1}} } \)
\( \newcommand{\vecd}[1]{\overset{-\!-\!\rightharpoonup}{\vphantom{a}\smash {#1}}} \)
\( \newcommand{\id}{\mathrm{id}}\) \( \newcommand{\Span}{\mathrm{span}}\)
( \newcommand{\kernel}{\mathrm{null}\,}\) \( \newcommand{\range}{\mathrm{range}\,}\)
\( \newcommand{\RealPart}{\mathrm{Re}}\) \( \newcommand{\ImaginaryPart}{\mathrm{Im}}\)
\( \newcommand{\Argument}{\mathrm{Arg}}\) \( \newcommand{\norm}[1]{\| #1 \|}\)
\( \newcommand{\inner}[2]{\langle #1, #2 \rangle}\)
\( \newcommand{\Span}{\mathrm{span}}\)
\( \newcommand{\id}{\mathrm{id}}\)
\( \newcommand{\kernel}{\mathrm{null}\,}\)
\( \newcommand{\range}{\mathrm{range}\,}\)
\( \newcommand{\RealPart}{\mathrm{Re}}\)
\( \newcommand{\ImaginaryPart}{\mathrm{Im}}\)
\( \newcommand{\Argument}{\mathrm{Arg}}\)
\( \newcommand{\norm}[1]{\| #1 \|}\)
\( \newcommand{\Span}{\mathrm{span}}\) \( \newcommand{\AA}{\unicode[.8,0]{x212B}}\)
\( \newcommand{\vectorA}[1]{\vec{#1}} % arrow\)
\( \newcommand{\vectorAt}[1]{\vec{\text{#1}}} % arrow\)
\( \newcommand{\vectorB}[1]{\overset { \scriptstyle \rightharpoonup} {\mathbf{#1}} } \)
\( \newcommand{\vectorC}[1]{\textbf{#1}} \)
\( \newcommand{\vectorD}[1]{\overrightarrow{#1}} \)
\( \newcommand{\vectorDt}[1]{\overrightarrow{\text{#1}}} \)
\( \newcommand{\vectE}[1]{\overset{-\!-\!\rightharpoonup}{\vphantom{a}\smash{\mathbf {#1}}}} \)
Trip Generation is the first step in the conventional four-step transportation forecasting process (followed by Destination Choice, Mode Choice, and Route Choice), widely used for forecasting travel demands. It predicts the number of trips originating in or destined for a particular traffic analysis zone.
Every trip has two ends, and we need to know where both of them are. The first part is determining how many trips originate in a zone and the second part is how many trips are destined for a zone. Because land use can be divided into two broad category (residential and non-residential) we have models that are household based and non-household based (e.g. a function of number of jobs or retail activity).
For the residential side of things, trip generation is thought of as a function of the social and economic attributes of households (households and housing units are very similar measures, but sometimes housing units have no households, and sometimes they contain multiple households, clearly housing units are easier to measure, and those are often used instead for models, it is important to be clear which assumption you are using).
At the level of the traffic analysis zone, the language is that of land uses "producing" or attracting trips, where by assumption trips are "produced" by households and "attracted" to non-households. Production and attractions differ from origins and destinations. Trips are produced by households even when they are returning home (that is, when the household is a destination). Again it is important to be clear what assumptions you are using.
People engage in activities, these activities are the "purpose" of the trip. Major activities are home, work, shop, school, eating out, socializing, recreating, and serving passengers (picking up and dropping off). There are numerous other activities that people engage on a less than daily or even weekly basis, such as going to the doctor, banking, etc. Often less frequent categories are dropped and lumped into the catchall "Other".
Every trip has two ends, an origin and a destination. Trips are categorized by purposes , the activity undertaken at a destination location.
Observed trip making from the Twin Cities (2000-2001) Travel Behavior Inventory by Gender
Some observations:
- Men and women behave differently on average, splitting responsibilities within households, and engaging in different activities,
- Most trips are not work trips, though work trips are important because of their peaked nature (and because they tend to be longer in both distance and travel time),
- The vast majority of trips are not people going to (or from) work.
People engage in activities in sequence, and may chain their trips. In the Figure below, the trip-maker is traveling from home to work to shop to eating out and then returning home.

Specifying Models
How do we predict how many trips will be generated by a zone? The number of trips originating from or destined to a purpose in a zone are described by trip rates (a cross-classification by age or demographics is often used) or equations. First, we need to identify what we think the relevant variables are.
The total number of trips leaving or returning to homes in a zone may be described as a function of:
\[T_h = f(housing \text{ }units, household \text{ }size, age, income, accessibility, vehicle \text{ }ownership)\]
Home-End Trips are sometimes functions of:
- Housing Units
- Household Size
- Accessibility
- Vehicle Ownership
- Other Home-Based Elements
At the work-end of work trips, the number of trips generated might be a function as below:
\[T_w=f(jobs(area \text{ }of \text{ } space \text{ } by \text{ } type, occupancy \text{ } rate\]
Work-End Trips are sometimes functions of:
- Area of Workspace
- Occupancy Rate
- Other Job-Related Elements
Similarly shopping trips depend on a number of factors:
\[T_s = f(number \text{ }of \text{ }retail \text{ }workers, type \text{ }of \text{ }retail, area, location, competition)\]
Shop-End Trips are sometimes functions of:
- Number of Retail Workers
- Type of Retail Available
- Area of Retail Available
- Competition
- Other Retail-Related Elements
A forecasting activity conducted by planners or economists, such as one based on the concept of economic base analysis, provides aggregate measures of population and activity growth. Land use forecasting distributes forecast changes in activities across traffic zones.
Estimating Models
Which is more accurate: the data or the average? The problem with averages (or aggregates) is that every individual’s trip-making pattern is different.
To estimate trip generation at the home end, a cross-classification model can be used. This is basically constructing a table where the rows and columns have different attributes, and each cell in the table shows a predicted number of trips, this is generally derived directly from data.
In the example cross-classification model: The dependent variable is trips per person. The independent variables are dwelling type (single or multiple family), household size (1, 2, 3, 4, or 5+ persons per household), and person age.
The figure below shows a typical example of how trips vary by age in both single-family and multi-family residence types.

The figure below shows a moving average.

Non-home-end
The trip generation rates for both “work” and “other” trip ends can be developed using Ordinary Least Squares (OLS) regression (a statistical technique for fitting curves to minimize the sum of squared errors (the difference between predicted and actual value) relating trips to employment by type and population characteristics.
The variables used in estimating trip rates for the work-end are Employment in Offices (\(E_{off}\)), Retail (\(E_{ret}\)), and Other (\(E_{oth}\))
A typical form of the equation can be expressed as:
\[T_{D,k}=a_1E_{off,k}+a_2E_{oth,k}+a_3E_{ret,k}\]
- \(T_{D,k}\) - Person trips attracted per worker in Zone k
- \(E_{off,i}\) - office employment in the ith zone
- \(E_{oth,i}\) - other employment in the ith zone
- \(E_{ret,i}\)- retail employment in the ith zone
- \(a_1,a_2,a_3\) - model coefficients
Normalization
For each trip purpose (e.g. home to work trips), the number of trips originating at home must equal the number of trips destined for work. Two distinct models may give two results. There are several techniques for dealing with this problem. One can either assume one model is correct and adjust the other, or split the difference.
It is necessary to ensure that the total number of trip origins equals the total number of trip destinations, since each trip interchange by definition must have two trip ends.
The rates developed for the home end are assumed to be most accurate,
The basic equation for normalization:
\[T'_{D,j}=T_{D,j} \dfrac{ \displaystyle \sum{i=1}^I T_{O,i}}{\displaystyle \sum{j=1}^J T_{TD,j}}\]
Sample Problems
Planners have estimated the following models for the AM Peak Hour
\(T_{O,i}=1.5*H_i\)
\(T_{D,j}=(1.5*E_{off,j})+(1*E_{oth,j})+(0.5*E_{ret,j})\)
\(T_{O,i}\) = Person Trips Originating in Zone \(i\)
\(T_{D,j}\) = Person Trips Destined for Zone \(j\)
\(H_i\) = Number of Households in Zone \(i\)
You are also given the following data
A. What are the number of person trips originating in and destined for each city?
B. Normalize the number of person trips so that the number of person trip origins = the number of person trip destinations. Assume the model for person trip origins is more accurate.
Solution to Trip Generation Problem Part A
\[T'_{D,j}=T_{D,j} \dfrac{ \displaystyle \sum{i=1}^I T_{O,i}}{\displaystyle \sum{j=1}^J T_{TD,j}}=>T_{D,j} \dfrac{37500}{36750}=T_{D,j}*1.0204\]
Solution to Trip Generation Problem Part B
Modelers have estimated that the number of trips leaving Rivertown (\(T_O\)) is a function of the number of households (H) and the number of jobs (J), and the number of trips arriving in Marcytown (\(T_D\)) is also a function of the number of households and number of jobs.
\(T_O=1H+0.1J;R^2=0.9\)
\(T_D=0.1H+1J;R^2=0.5\)
Assuming all trips originate in Rivertown and are destined for Marcytown and:
Rivertown: 30000 H, 5000 J
Marcytown: 6000 H, 29000 J
Determine the number of trips originating in Rivertown and the number destined for Marcytown according to the model.
Which number of origins or destinations is more accurate? Why?
T_Rivertown =T_O ; T_O= 1(30000) + 0.1(5000) = 30500 trips
T_(MarcyTown)=T_D ; T_D= 0.1(6000) + 1(29000) = 29600 trips
Origins(T_{Rivertown}) because of the goodness of fit measure of the Statistical model (R^2=0.9).
Modelers have estimated that in the AM peak hour, the number of trip origins (T_O) is a function of the number of households (H) and the number of jobs (J), and the number of trip destinations (T_D) is also a function of the number of households and number of jobs.
\(T_O=1.0H+0.1J;R^2=0.9\)
Suburbia: 30000 H, 5000 J
Urbia: 6000 H, 29000 J
1) Determine the number of trips originating in and destined for Suburbia and for Urbia according to the model.
2) Does this result make sense? Normalize the result to improve its accuracy and sensibility?
- \(T_{O,i}\) - Person trips originating in Zone i
- \(T_{D,j}\) - Person Trips destined for Zone j
- \(T_{O,i'}\) - Normalized Person trips originating in Zone i
- \(T_{D,j'}\) - Normalized Person Trips destined for Zone j
- \(T_h\) - Person trips generated at home end (typically morning origins, afternoon destinations)
- \(T_w\) - Person trips generated at work end (typically afternoon origins, morning destinations)
- \(T_s\) - Person trips generated at shop end
- \(H_i\) - Number of Households in Zone i
- \(E_{off,k}\) - office employment in Zone k
- \(E_{ret,k}\) - retail employment in Zone k
- \(E_{oth,k}\) - other employment in Zone k
- \(B_n\) - model coefficients
Abbreviations
- H2W - Home to work
- W2H - Work to home
- W2O - Work to other
- O2W - Other to work
- H2O - Home to other
- O2H - Other to home
- O2O - Other to other
- HBO - Home based other (includes H2O, O2H)
- HBW - Home based work (H2W, W2H)
- NHB - Non-home based (O2W, W2O, O2O)
External Exercises
Use the ADAM software at the STREET website and try Assignment #1 to learn how changes in analysis zone characteristics generate additional trips on the network.
Additional Problems
- the start and end time (to the nearest minute)
- start and end location of each trip,
- primary mode you took (drive alone, car driver with passenger, car passenger, bus, LRT, walk, bike, motorcycle, taxi, Zipcar, other). (use the codes provided)
- purpose (to work, return home, work related business, shopping, family/personal business, school, church, medical/dental, vacation, visit friends or relatives, other social recreational, other) (use the codes provided)
- if you traveled with anyone else, and if so whether they lived in your household or not.
Bonus: Email your professor at the end of everyday with a detailed log of your travel diary. (+5 points on the first exam)
- Are number of destinations always less than origins?
- Pose 5 hypotheses about factors that affect work, non-work trips? How do these factors affect accuracy, and thus normalization?
- What is the acceptable level of error?
- Describe one variable used in trip generation and how it affects the model.
- What is the basic equation for normalization?
- Which of these models (home-end, work-end) are assumed to be more accurate? Why is it important to normalize trip generation models
- What are the different trip purposes/types trip generation?
- Why is it difficult to know who is traveling when?
- What share of trips during peak afternoon peak periods are work to home (>50%, <50%?), why?
- What does ORIO abbreviate?
- What types of employees (ORIO) are more likely to travel from work to home in the evening peak
- What does the trip rate tell us about various parts of the population?
- What does the “T-statistic” value tell us about the trip rate estimation?
- Why might afternoon work to home trips be more or less than morning home to work trips? Why might the percent of trips be different?
- Define frequency.
- Why do individuals > 65 years of age make fewer work to home trips?
- Solve the following problem. You have the following trip generation model:
\[Trips=B_1Off+B_2Ind+B_3Ret\]
And you are given the following coefficients derived from a regression model.
If there are 600 office employees, 300 industrial employees, and 200 retail employees, how many trips are going from work to home?
- Forecasting
7 comments
Traffic Impact Study Improvements: Part 5 – When is a Trip Not a Trip?
By Mike Spack
October 27, 2015
Guest Post by Bryant Ficek, PE, PTOE, Vice President at Spack Consulting
Earlier this year, I detailed how our standard process for a Traffic Impact Study has several points of assumptions at best or guesses at worst. This post continues that discussion. Check out the “ Top 6 Ways to Pick Apart a Traffic Study ” for more on the general topic and expect more posts to follow on this subject.
Trip generation is the process of estimating the amount of traffic a proposed development will have once it is built and operating. Trip distribution is the process by which we take the raw projected traffic for a development (trip generation) and add it to the existing volumes on the transportation network. The step in-between is determining whether all the trip generation will be new to the roadway.
To start with, there are several types of trips as follows (with definition summarized from the Institute of Transportation Engineers or ITE). The figure below illustrates the different types of trips.
- Primary or New. Traffic with the specific purpose of visiting the site being studied.
- Pass-By. Traffic already on the way from an origin to a primary trip destination that will make an intermediate stop at the site being studied without a route diversion.
- Diverted. Traffic attracted to the site being studied from adjacent facilities without direct access to the site. A diverted trip example is a through trip on a freeway that diverts to an exit and a development, adding traffic to the local road but removing traffic from the freeway.
- Internal. Traffic associated with multi-use developments where trips among various land uses can be made on the site being studied without using the major street system. These trips can be made either by walking or by vehicles using internal roadways.
These different trip generation options, combined with so many different types of land uses, leads to virtually limitless possibilities for the amount and type of traffic a particular site could generate on the roadway system. As with our trip distribution column, we initially thought about testing multiple scenarios, which would be relatively easy with today’s software. At least, theoretically. To restate our collective conclusion – While interesting on a pure research level, a thicker actual traffic impact study report covering multiple results leads us down a path no one wants to go.
Furthermore, sub-dividing the raw trip generation into parts is not something that can be quantified into a “one-size fits all” equation. Given the possibilities and the limits of our collective traffic research to date, ITE provides the best procedure to follow. So this article is dedicated to reviewing that procedure, which is spelled out in ITE’s Trip Generation Handbook and Trip Generation Manual, Volume 1 . That step-by-step process is as follows:
- Raw Trip Generation. Using ITE or other land use information (try tripgeneration.org !), calculate the raw trip generation for the site.
- Pass-By and Diverted Number of Trips. Use either local data or ITE data to determine a percentage of the reduced trip generation that is pass-by or diverted. Similar to the ITE Trip Generation data, both pass-by and diverted trip percentages are available by average rate or an equation for many land uses. Use this percentage to calculate the total pass-by and diverted trips for the site.
- Pass-By and Diverted Trip Patterns. Use the existing traffic to determine how the pass-by and diverted trips will access the site.
- Pass-By and Diverted Trip Volume Adjustment. Apply the existing traffic patterns to the pass-by and diverted trips to establish the impact on the roadway system for these trips.
- Remaining Primary/New trips. Determine the remaining trip generation after reducing for internal trips and then removing the pass-by and diverted trips.
- Primary/New Trip Pattern. We discussed factors to consider for the primary/new trip distribution in Part 4 of this series.
- Primary/New Trip Volume Adjustment . Apply the trip distribution to the primary/new trips to determine the impact on the roadway system for these trips.
- Final Volumes. Combine the pass-by, diverted, and primary/new trips at each study intersection to determine the final impact of the site being studied.
We can demonstrate this process on a theoretical study site with the following characteristics:
- 17,000 square feet of office, 3,000 square feet of fast food with a drive-thru, and 10 vehicle fueling positions at a gas station with convenience market
- One driveway accesses the site off a busy road (1,000 vehicles in the p.m. peak hour)
- A highway interchange with the busy road is located just east of the site
- Trip Generation (PM Peak)
- General Office, Land Use 710 – 98 raw trips
- Fast Food with Drive Thru, Land Use 934 – 98 raw trips
- Gas Station with Convenience Market, Land Use 945 – 136 raw trips
- Internal Trips
4. Pass-By and Diverted Patterns (per the theoretical roadway data)
6. Remaining Primary/New trips:
- Office – (98 raw – 5 internal – 0 pass-by – 0 diverted) = 93 primary/new trips
- Fast Food – (98 raw – 21 internal – 43 pass-by – 23 diverted) = 11 primary/new trips
- Gas Station – (136 raw – 22 internal – 57 pass-by – 26 diverted) = 31 primary/new trips
7. Primary/New Trip Pattern (per knowledge of theoretical area)
8. Primary/New Trip Volume Adjustment
9. Final Volumes (add the pass-by, diverted, and primary/new trips together)
We don’t include all of the above example graphs in our reports. Instead, our short-hand method is a trip generation table that looks like this:
As a final note, the internal, pass-by, diverted, and new percentages are often adjusted from the base ITE information. ITE itself notes the limited amount of data available and the inherent variability in surveyed sites. The best approach, if possible, is to discuss the percentages with the governing agency to achieve agreement and buy-in before you get too far down the path in your analysis.
Did you miss the other installments of the Traffic Impact Study Improvements series? Here are the links to the other articles:
- Part 1 – Traffic Counts
- Part 2 – Would Multiple Results Help Us?
- Part 3 – All Trips are Equal, But Some Trips are More Equal Than Others
At what point would you apply a reduction for non-SOV trips such as transit? It looks like ITE would have you apply internal capture, transit and then pass-by.
very precious and informative post…thanks so much..I had found the answer of one of my question in another post of yours..thanks for sharing your experiences
Sometimes I drive right by a coffee shop and then think, hmm, I could really use a good cup of black coffee and then I turn around and go back. Is this a pass-by trip or a diverted trip (or both?). What about a cappuccino?
If the need for java hit as you approached the site, and you turned right in, this would be a standard diversion. But since you passed the driveway and turned around, ….you need to take public transit and stop burning up our resources.
Hey Mike, After you do trip gen. calcs (let’s say for a built-up year 2029) but you want to consider design year (typical 20 years) and you want to design/improve nearby roads for the design year 2049 (2029 + 20 years); do you apply same growth rate on trip generated as you would do for existing traffic? or you apply the growth rate on existing traffic, and use trip gen numbers without applying a growth rate?
No – the growth rate is not applied to the trip generation. For instance, the trips generated by a single family home isn’t going to keep growing over time. They’re assumed to be static.
My mission is to help traffic engineers, transportation planners, and other transportation professionals improve our world.
Get these blog posts sent to your email! Sign up below.
Session expired
Please log in again. The login page will open in a new tab. After logging in you can close it and return to this page.
Get our information in your Inbox!
Sign up below to stay informed

Trip generation
What is trip generation .
A trip is usually defined in transport modeling as a single journey made by an individual between two points by a specified mode of travel and for a defined purpose. Trips are often considered as productions of a particular land-use and attracted to other specified land-uses. The number of trips arises in unit time, usually for a specified zonal land use , is called the trip generation rate.
How to estimate trip generation ?
Trip generation is estimated in three ways:
(i) traditionally by linear and multiple regression
(ii) by aggregating the trip generating capability of a household or car and aggregating the total according to the distribution of each selected category in the zones, and
(iii) by household classification method through a catalogue of the characteristic mean trip rates for specific types of household.
The attraction points are identified as trip generated by work, and other purpose visits. By assigning suitable values to the independent variables of the regression equations forecasts can be made of the future trip ends for zones by either method.

Trip distribution :Trip generation estimates the number and types of trips originating and terminating in zones. Trip distribution is the process of computing the number of trips between one zone and all other. A trip matrix is drawn up with the sums of rows indicating the total number of trips originating in zone i and the sums of columns the total number of destinations attracted to zone j.
Each cell in the matrix indicates the number of trips that go from each origin zone to each destination zone. The trips on the diagonal are intra-zonal trips, trips that originate and end in the same zone. The balancing equation is implemented in a series of steps that include modeling the number of trips originating in each cases, adding in trips originating from outside the study area(external trips), and statistically balancing the origins and destinations.
This is done in the trip generation stage. But, it is essential that the step should have been completed for the trip distribution to be implemented. Two trip distribution matrices need to be distinguished. The first is the observed distribution. This is the actual number of trips that are observed traveling between each origin zone and each destination zone. It is calculated by simply enumerating the number of trips by each origin-destination combination. It is also called trip-link. The second distribution is a model of the trip distribution matrix, called the predicted distribution.
Generally trips should be distributed over the area proportionally to the attractiveness of activities and inversely proportional to the travel resistances between areas. It is assumed that the trips between zones will be by the most direct or cheapest routes and, taking each zone in turn, a minimum path is traced out to all other zones to form a minimum path tree. The trip distribution is a model of travel between zones-trips or links. The modeled trip distribution can then be compared to the actual distribution to see whether the model produces a reasonable approximation.
Read about: Zoning of Land for OD Survey , Traffic Volume Count , Origin Destination Survey Methods
About The Author
Fundamentals of Transportation/Trip Generation
Trip Generation is the first step in the conventional four-step transportation forecasting process (followed by Destination Choice , Mode Choice , and Route Choice ), widely used for forecasting travel demands. It predicts the number of trips originating in or destined for a particular traffic analysis zone.
Every trip has two ends, and we need to know where both of them are. The first part is determining how many trips originate in a zone and the second part is how many trips are destined for a zone. Because land use can be divided into two broad category (residential and non-residential) we have models that are household based and non-household based (e.g. a function of number of jobs or retail activity).
For the residential side of things, trip generation is thought of as a function of the social and economic attributes of households (households and housing units are very similar measures, but sometimes housing units have no households, and sometimes they contain multiple households, clearly housing units are easier to measure, and those are often used instead for models, it is important to be clear which assumption you are using).
At the level of the traffic analysis zone, the language is that of land uses "producing" or attracting trips, where by assumption trips are "produced" by households and "attracted" to non-households. Production and attractions differ from origins and destinations. Trips are produced by households even when they are returning home (that is, when the household is a destination). Again it is important to be clear what assumptions you are using.
- 1 Activities
- 2.1 Home-end
- 2.2 Work-end
- 2.3 Shop-end
- 3 Input Data
- 4.1 Home-end
- 4.2 Non-home-end
- 5 Normalization
- 6 Sample Problems
- 7 Variables
- 8 Abbreviations
- 9 External Exercises
- 10 Additional Problems
- 11 End Notes
- 12 Further reading
- 14 References
People engage in activities, these activities are the "purpose" of the trip. Major activities are home, work, shop, school, eating out, socializing, recreating, and serving passengers (picking up and dropping off). There are numerous other activities that people engage on a less than daily or even weekly basis, such as going to the doctor, banking, etc. Often less frequent categories are dropped and lumped into the catchall "Other".
Every trip has two ends, an origin and a destination. Trips are categorized by purposes , the activity undertaken at a destination location.
Some observations:
- Men and women behave differently on average, splitting responsibilities within households, and engaging in different activities,
- Most trips are not work trips, though work trips are important because of their peaked nature (and because they tend to be longer in both distance and travel time),
- The vast majority of trips are not people going to (or from) work.
People engage in activities in sequence, and may chain their trips. In the Figure below, the trip-maker is traveling from home to work to shop to eating out and then returning home.

Specifying Models
How do we predict how many trips will be generated by a zone? The number of trips originating from or destined to a purpose in a zone are described by trip rates (a cross-classification by age or demographics is often used) or equations. First, we need to identify what we think the relevant variables are.
The total number of trips leaving or returning to homes in a zone may be described as a function of:
Home-End Trips are sometimes functions of:
- Housing Units
- Household Size
- Accessibility
- Vehicle Ownership
- Other Home-Based Elements
At the work-end of work trips, the number of trips generated might be a function as below:
Work-End Trips are sometimes functions of:
- Area of Workspace
- Occupancy Rate
- Other Job-Related Elements
Similarly shopping trips depend on a number of factors:
Shop-End Trips are sometimes functions of:
- Number of Retail Workers
- Type of Retail Available
- Area of Retail Available
- Competition
- Other Retail-Related Elements
A forecasting activity conducted by planners or economists, such as one based on the concept of economic base analysis, provides aggregate measures of population and activity growth. Land use forecasting distributes forecast changes in activities across traffic zones.
Estimating Models
Which is more accurate: the data or the average? The problem with averages (or aggregates) is that every individual’s trip-making pattern is different.
To estimate trip generation at the home end, a cross-classification model can be used. This is basically constructing a table where the rows and columns have different attributes, and each cell in the table shows a predicted number of trips, this is generally derived directly from data.
In the example cross-classification model: The dependent variable is trips per person. The independent variables are dwelling type (single or multiple family), household size (1, 2, 3, 4, or 5+ persons per household), and person age.
The figure below shows a typical example of how trips vary by age in both single-family and multi-family residence types.

The figure below shows a moving average.

Non-home-end
The trip generation rates for both “work” and “other” trip ends can be developed using Ordinary Least Squares (OLS) regression (a statistical technique for fitting curves to minimize the sum of squared errors (the difference between predicted and actual value) relating trips to employment by type and population characteristics.
A typical form of the equation can be expressed as:
- Normalization
For each trip purpose (e.g. home to work trips), the number of trips originating at home must equal the number of trips destined for work. Two distinct models may give two results. There are several techniques for dealing with this problem. One can either assume one model is correct and adjust the other, or split the difference.
It is necessary to ensure that the total number of trip origins equals the total number of trip destinations, since each trip interchange by definition must have two trip ends.
The rates developed for the home end are assumed to be most accurate,
The basic equation for normalization:
Sample Problems
- Problem ( Solution )
Abbreviations
- H2W - Home to work
- W2H - Work to home
- W2O - Work to other
- O2W - Other to work
- H2O - Home to other
- O2H - Other to home
- O2O - Other to other
- HBO - Home based other (includes H2O, O2H)
- HBW - Home based work (H2W, W2H)
- NHB - Non-home based (O2W, W2O, O2O)
External Exercises
Use the ADAM software at the STREET website and try Assignment #1 to learn how changes in analysis zone characteristics generate additional trips on the network.
- Additional Problems
Further reading
- Trip Generation article on wikipedia
- Trip Generation
- Book:Fundamentals of Transportation
Navigation menu
- Experiment List
You have not logged in!!!
please login to perform the experiment.
Trip Generation
Trip Generation is the first step in the Sequential Demand Modelling arrangement which is also called as the Four Step Transportation Planning Process(FSTP) as mentioned earlier. In order to carry out modelling, the variable consists of total number of person-trips generated by a zone as a dependent variable and the independent variable consists of household and socio- economic factors which influence the trip making behaviour of the person. The data for the independent variable should be attained from an analyst. The output thus obtained consists of trip making or trip ends for each zone within a region.
In contemporary transportation planning language, A Trip is defined as a one way person movement by a mechanized mode of transport, having two trip ends. The start of the trip is called as origin and the end of trip is called as destination. Trip is classified as Production or Origin and Attraction or Destination. It should be note that the terminologies used are not identical. To understand with an example consider a single worker on a typical working day making a trip from his house which is in zone P to his office in Zone Q . Thus his trip origin will be zone P and trip destination will be zone Q . For the return trip from office to house his trip origin will be zone Q and trip Destination will be Zone P . Thus from the above Example it can be understood that the term Origin and Destination are defined in terms of direction of the trip while Production and Attraction in terms of land use associated with each trip end. Trip Production is the home end of home based trip and is the origin of trip of non home based trip. Trip Attraction is the non home end of home based trip and is the destination of a non home based trip.

It has been found that better trip generation models can be obtained if the trips by different purpose are identified and modelled separately.The trips can be classified as given below:
1. Home Based Trip: One of the trip end is home.
Example: A trip from home to office.
Following are the list of home based trips that is trip purpose which are classified into five categories:
a. Work Trips
b. School Trips
c. Shopping Trips
d. Social- recreational Trips
e. Other Trips
The first two trips are mandatory trips while other trips are discretional trips. The other trip class encompasses all the trips made for less routine purpose such as health bureaucracy etc.
2. Non Home based trips: None of the trip end is home.
Example: A trip from office to Shopping Mall.
3. Time based trips
The proportion of journey is different by different purposes usually varies with time of the day. Thus the classification is often given as Peak and Off Peak Period Trip.
4. Person-type based trips
The travel behaviour of an individual is mainly dependent on its Socio-Economic attributes. Following are the categories which are usually employed.
a. Income Level- Poor, Middle Class, Rich
b. Car Ownership- 0,1,2,3
c. Household Size- 1,2,3,4... etc
a. No. of workers in a household.
b. No. of Students.
c. Household size and composition.
d. The household income.
e. Some proxy of income such as number of cars etc.
a. Floor area and number of employment opportunities in retail trade, service, offices manufacturing and wholesale areas.
b. School and college enrolment
c. Other activity centres like transport terminals, sports stadium, major recreational/ cultural/religious places
Table below represents base year data of Trip Production for exact zone.
Similarly Trip Attraction Table is obtained with respect to its influencing variables.
Trip generation study typically involves the application of residential trip production which contains variable that defines the demographic makeup of zonal population and trip attraction that captures the activity of non residential activities within the zone.
In the example given below the zones are connected by a two way link. Each zone will have its own demographic and non residential characteristics depending on which the Trip Generation table is represented below.

Modelling basically relates the dependent variable ie trips produced by a zone for aggregated model or household trip production rate for household based models to the corresponding Independent variables characterised by the whole zone or household characteristic respectively. Calibration is done based on the set of observations obtained corresponding to the zones for aggregate model and for disaggregate model employs a number of base year observations corresponding to an individual household in a sample of household drawn randomly from the region. Thus we first need to identify what are the relevant variables: a. Home end b. Work End c. Shop End
Analytical tools used for Trip Generation Modelling are given below: 1. Regression Model (Regression Analysis) 2. Cross Classification Model (Category Analysis)
The purpose of trip generation is to estimate the number of trip ends for each zones for the targeted year. The trip end is calculated for different travel purpose within the zone. These trips are represented as residential trip production obtained from household based cross classification tables or non residential trip attractions which is obtained by projection of land use. Trip generation Models that are often used are Multiple Linear Regression Model or Cross Classification Model or involves combination of both.

Long-Distance and Rural Travel Transferable Parameters for Statewide Travel Forecasting Models (2012)
Chapter: chapter 4 - trip generation parameters and benchmark statistics.
Below is the uncorrected machine-read text of this chapter, intended to provide our own search engines and external engines with highly rich, chapter-representative searchable text of each book. Because it is UNCORRECTED material, please consider the following text as a useful but insufficient proxy for the authoritative book pages.
47 This, and subsequent sections on transferable parameters and benchmark statistics, will fol- low a similar format. The first subsection will provide benchmark statistics and parameters from existing statewide models. This will be followed by a discussion of analytical approaches used to estimate transferable parameters. Next will be a presentation of long-distance transferable parameters and benchmarks. Each section will then conclude with rural travel parameters and benchmarks. The section on trip generation specifically touches on alternate trip generation approaches to statewide models, and statewide model trip purposes, as well as differences between urban, rural, and long-distance trip-making. This will be followed by a presentation of transferable trip production rates and guidance on making adjustments to these parameters. This section will also provide benchmark statistics on aggregate trip rates and percent trips by purpose. 4.1 Long-Distance and Rural Trip Generation Benchmark Statistics from Statewide Models and Other Sources This section of the trip generation chapter explores the characteristics of statewide models further to identify sources that could be used in comparing, developing, and recommending trip production rates for estimating rural and long-distance travel. Other statewide model statistics such as friction factors, mode choice coefficients, and peak-to-daily/time-of-day factors, and other model parameters, are summarized later in Sections 5.1, 6.1, and 7.1, which are devoted to other steps in the four-step modeling process. Other secondary sources of model parameters and benchmarks are provided for comparative purposes. Statewide Model Parameters and Benchmarks The final report for the NCHRP Statewide Model Validation Study (Cambridge Systematics, Inc., 2010d) included a series of tables describing model parameters and benchmark statistics from statewide models, including information on long-distance and rural trip purposes, where these were separated from typical urban model purposes. Some of this information was derived either from recent work on the NCHRP model validation report or prior work on national model research for FHWA. Establishment of trip purposes used in statewide models is important because this will largely determine the stratifications used in subsequent model statistics (i.e., these are reported by trip purpose). Some trip purposes in statewide models are duplicative, using different names but meaning the same thing. This has been fleshed out through discussions with state DOT contacts C h a p t e r 4 Trip Generation Parameters and Benchmark Statistics
48 Long-Distance and rural travel transferable parameters for Statewide travel Forecasting Models and their consultants. Some models differentiate short-distance from long-distance trip purposes while others do not. Where long-distance trips are separated from routine travel, the percent of long-distance trips varies widely in statewide models from less than 1 percent (Florida, Louisiana) to greater than 4 percent (Massachusetts); this may reflect, to some extent, the close proximity of densely developed urbanized areas, resulting high levels of through-trip activity, long-distance commuting, and other unique factors that make transferability of this statistic difficult. Also, reported statistics make use of different thresholds for long-distance travel. Trip generation model statistics compiled by trip purpose include aggregate trip rates and percent trips by purpose. In many cases, states have incorporated methods for forecasting long- distance trips along with shorter regional trips in their statewide models. In most cases, statewide models incorporate truck and auto long-distance trips; however, in some cases, additional modes are incorporated such as air and intercity transit. The threshold for defining long-distance trips also varies among statewide models, with some states considering trips over 100 miles to be long distance, and others considering 50 miles or 75 minutes as long distance. Table 4.1 is a summary of the percentage of trips that are long-distance for each statewide model, along with a breakdown of each stateâs definition for long-distance trips, as reported in available technical reports. Long-distance trip production rates were documented for Georgia and Wisconsin statewide models only, as depicted in Tables 4.2 and 4.3. No trip attraction rates were found for rural or long-distance travel in any of statewide model documents reviewed. These tables, as well as other statewide model statistics found in subsequent sections, depict passenger trips except where noted otherwise. The numbers found in these tables, in all cases, came directly from statewide model technical reports because the study team was not tasked with obtaining and executing these models. Bureau of Transportation Statistics In May 2006, the Bureau of Transportation Statistics (BTS) published findings from the 2001 NHTS on long-distance trip-making. A number of these statistics could be useful as transferable parameters or benchmark statistics against which to compare statewide model results. Although later tasks in this study will include data analysis of 2009 NHTS, 2001 NHTS, 1995 ATS, and other relevant state survey datasets, it was thought that information from the BTS report, America on the Go, Findings from the National Household Travel Survey (U.S. Department of Transportation, Long-Distance Threshold Long-Distance Total Percentage in Miles in Minutes Trips Trips Long Distance Arizona 50 â â â â California 100 â â â â Florida 50 â 176,587 52,281,363 0.34% Georgia â 75 418,000 31,223,000 1.34% Indiana â â 280,395 25,158,208 1.11% Louisiana 100 â 75,087 11,717,965 0.64% Massachusetts â â 957,046 22,951,483 4.17% Mississippi 100 â 212,862 7,095,161 3.00% Ohio 50 â 248,628 36,702,991 0.60% Utah â â 68,866 7,313,412 0.94% Virginia â 100 1,071,566 37,868,443 2.83% Wisconsin 50 â 42,966 71,313,993 0.06% Table 4.1. Percentages of long-distance trips in statewide models.
trip Generation parameters and Benchmark Statistics 49 Table 4.2. Georgia long-distance internal and external trip rates by purpose, income, area, and persons per household. Income Area Persons per Household HBW-IE (GA Int-Ext) HBW-II (GA Internal) HBO-II (GA Internal) NHB-II (GA Internal) Low Urban 1 0.008 0.001 0.036 0.005 2 0.045 0.002 0.063 0.009 3 0.025 0.003 0.083 0.020 4 0.077 0.005 0.060 0.154 Rural 1 0.045 0.045 0.016 0.010 2 0.020 0.043 0.087 0.130 3 0.091 0.003 0.045 0.040 4 0.056 0.167 0.667 0.056 Non-Low Urban 1 0.016 0.003 0.013 0.010 2 0.046 0.005 0.041 0.017 3 0.051 0.009 0.041 0.054 4 0.051 0.015 0.127 0.036 Rural 1 0.015 0.002 0.032 0.021 2 0.035 0.022 0.104 0.042 3 0.052 0.007 0.095 0.087 4 0.070 0.022 0.081 0.059 Source: Atkins, Development of Statewide Model Draft Report, prepared for Georgia Department of Transportation, April 15, 2011. 1 Household Member 2 Household Members 3 Household Members 4 Household Members 0 Autos 1 Auto 2 Autos 0 Autos 1 Auto 2 Autos 0 Autos 1 Auto 2 Autos 0 Autos 1 Auto 2 Autos Business Appleton/Oshkosh/Green Bay 0.00000 0.00367 0.01316 0.00000 0.00479 0.01997 0.00000 0.00057 0.02917 0.00000 0.00792 0.03429 Madison 0.00046 0.00545 0.02347 0.00000 0.00624 0.02737 0.00000 0.01451 0.02480 0.00000 0.06359 0.03617 All other MPOs 0.00145 0.00633 0.03949 0.00145 0.01656 0.02953 0.00145 0.00829 0.04266 0.00145 0.02101 0.04851 SEWRPC Region 0.00148 0.00405 0.00851 0.00148 0.00370 0.02202 0.00148 0.00251 0.01687 0.00148 0.00909 0.03399 Rest of Wisconsin 0.00060 0.00647 0.04464 0.00060 0.01875 0.02487 0.00060 0.00590 0.04473 0.00060 0.02524 0.05001 Personal Business Appleton/Oshkosh/Green Bay 0.00133 0.00293 0.00576 0.00133 0.01358 0.01133 0.00133 0.01370 0.02396 0.00133 0.01475 0.02079 Madison 0.00352 0.00427 0.00583 0.00352 0.01175 0.01246 0.00352 0.01092 0.01461 0.00352 0.00836 0.02078 All other MPOs 0.00107 0.00436 0.01110 0.00107 0.01415 0.02099 0.00107 0.01373 0.03127 0.00107 0.03690 0.02986 SEWRPC Region 0.00895 0.00895 0.00895 0.00895 0.00895 0.00895 0.00895 0.00895 0.00895 0.00895 0.00895 0.00895 Rest of Wisconsin 0.00077 0.00479 0.01266 0.00077 0.01523 0.02234 0.00077 0.01025 0.03376 0.00077 0.04535 0.03144 Pleasure Appleton/Oshkosh/Green Bay 0.00752 0.01399 0.02085 0.00752 0.05138 0.06096 0.00752 0.05508 0.07750 0.00752 0.05330 0.12458 Madison 0.01538 0.01773 0.01712 0.01538 0.04875 0.06335 0.01538 0.04614 0.08682 0.01538 0.07146 0.12193 All other MPOs 0.00684 0.01829 0.02851 0.00684 0.04550 0.07099 0.00684 0.04713 0.08072 0.00684 0.06755 0.09718 SEWRPC Region 0.00717 0.01443 0.01740 0.00717 0.03076 0.05512 0.00717 0.03350 0.06772 0.00717 0.02557 0.07727 Rest of Wisconsin 0.00440 0.01575 0.26130 0.00440 0.03908 0.05959 0.00440 0.05192 0.08034 0.00440 0.08730 0.10344 Source: Cambridge Systematics, Inc. and HNTB, Wisconsin Statewide Model âPassenger and Freight Models, prepared for Wisconsin Department of Transportation, September 2006. Table 4.3. Wisconsin daily long-distance trip rates by purpose, household size, and number of autos.
50 Long-Distance and rural travel transferable parameters for Statewide travel Forecasting Models Research and Innovative Technology Administration, Bureau of Transportation Statistics, 2006) fit into the context of this discussion. This 2006 BTS analysis of long-distance trips identified characteristics such as percent of trips by mode and purpose, as depicted in Table 4.4. According to BTSâ analysis, over 50 per- cent of long-distance trips would be considered for the purposes of pleasure, with another 16 percent of trips occurring for business purposes. Travel modes are fairly consistent for most long-distance purposes with the exception of business trips, which are far more likely to use air travel than other long-distance trip purposes. This shows the importance of modeling long- distance business trips separately from other LD trip types when modeling multiple transpor- tation modes. Oak Ridge National Laboratories A 2006 report, Trends in New York State Long-Distance Travel (Oak Ridge National Labora- tory, 2006), produced by staff from the Oak Ridge National Laboratory, provides a number of statistics on long-distance travel patterns based on analyses of 1995 ATS and 2001 NHTS data for residents of, and visitors to, the State of New York. Statistics provided in this report include growth in long-distance trips, the number of person trips, trips per person, miles per person, and miles per trip tabulated by means of transportation, trip purpose, income, age, and gen- der. These statistics are not necessarily transferable to other states but could be useful in benchmark checking against comparable statistics calculated from other surveys or for other states. A 2009 NHTS Update to this report is under way and select chapters are available for downloading (https://www.dot.ny.gov/divisions/policy-and-strategy/darb/dai-unit/ttss/nhts/ 2009-comparision-report). Statewide Travel Surveys by State DOTs The Ohio statewide model considers long-distance trips to be 50 miles or greater, excluding work tours (Ohio Department of Transportation, Report-Ohio-LongDistanceTravelModule- Extracted.pdf Section 4.7, LDT). Background information on the Ohio Long-Distance Travel Survey was provided in Section 2.5 of this report. Attention was focused on summary statis- tics that already were reported in available survey documentation. Section 2.5 provides graphs depicting the frequency of, and travel modes used in, long-distance trip-making based on the Ohio surveys. In addition to concerns over resource sufficiency to analyze additional state data- sets using SAS as part of this research effort, there is the issue of whether or not a supplemental Percent Trips by Mode LD Purpose Percent by Purpose Personal Vehicle Air Bus Train Other Pleasure 55.5% 90.4% 6.7% 2.2% 0.5% 0.2% Business 15.9% 79.3% 17.8% 0.8% 1.6% 0.5% Commuting 12.6% 96.4% 1.5% 0.5% 1.7% 0.0% Personal Business 12.6% 89.3% 4.7% 5.6% 0.3% 0.1% Other 3.4% 96.6% 1.9% 0.5% 0.0% 1.0% Total 100.0% 89.5% 7.4% 2.1% 0.8% 0.2% Source: BTS. Table 4.4. 2001 long-distance trips by purpose and mode.
trip Generation parameters and Benchmark Statistics 51 analysis of state DOT datasets would provide the same results as previously reported, due to weighting/expansion and tools used in the analysis. The Ohio statewide travel survey documentation provided to the study team did include person trip rates per household (7.78) and person (4.94) for rural versus urban settings (7.56â8.76 per HH and 4.83â5.49 per person, depending on the specific urban area). The survey documentation, however, does not include long-distance trip rates, trips by pur- pose, trip distribution factors, average trip lengths, mode splits, or auto occupancy rates. Beyond sociodemographic characteristics of survey respondents and the graphs and charts previously depicted in earlier chapters of this Guidebook, information was also provided on the number of stops (60.9 percent made stops) for long-distance trips and the percent of nonhome-based long-distance trips (53 percent). Section 2.6 of this report provides background information on the Michigan surveys, includ- ing annual long-distance household trip rates (7.34 in 2004 and 6.25 in 2009), trip purposes, travel modes, and long-distance trip distribution by state. The difference between Michigan long-distance trip rates and those based on ATS (10.15) and 2001 NHTS (12.32) excluding 50â100 mile trips, indicate potential issues of transferability as long-distance trips were defined as 100 miles or greater in the Michigan surveys. Considerable information was provided in the Michigan documentation about trip characteristics that are more relevant to the discussion of rural trip rates in Chapter 3 of this report. The Michigan statewide household survey documentation provided household person trip rates for different urban and rural stratifications. Table 4.5 depicts person trip rates per house- hold for the first and second Michigan Travel Counts Surveys (2004 and 2009, respectively). Nonurbanized and rural household and person trip rates are depicted in bold underlined text, Sample Areas Households Weighted Persons Weighted HH Trip Rates Person Trip Rates Unweighted Weighted Unweighted Weighted Estimated Number of HHs, Persons, and Trips by MTC I (2004) SEMCOG 1,846,277 4,638,216 9.09 9.14 3.62 3.64 Small Cities (0-50k pop) 129,369 296,162 9.74 8.82 3.89 3.85 Upper Peninsula Rural 87,115 209,919 8.35 8.40 3.49 3.49 Northern Lower Peninsula Rural 206,210 501,075 8.08 7.96 3.30 3.27 Southern Lower Peninsula Rural 394,588 1,044,969 8.98 9.41 3.53 3.55 TMAs 579,415 1,465,017 9.68 9.53 3.78 3.77 Small Urban Modeled Areas 545,557 1,360,511 9.29 9.39 3.75 3.77 State Total 3,788,531 9,515,870 9.05 9.17 3.63 3.65 Estimated Number of HHs, Persons, and Trips by MTC II (2009) SEMCOG 2,071,786 4,820,277 7.73 8.60 3.66 3.70 Small Cities (0-50k pop) 147,121 315,640 9.10 8.14 3.96 3.80 Upper Peninsula Rural 90,553 212,970 7.29 7.64 3.28 3.25 Northern Lower Peninsula Rural 218,238 520,125 7.32 7.93 3.30 3.33 Southern Lower Peninsula Rural 412,944 1,078,905 8.07 9.15 3.43 3.50 TMAs 622,928 1,519,419 8.34 8.83 3.65 3.62 Small Urban Modeled Areas 593,556 1,399,086 7.87 8.72 3.68 3.70 State Total 4,157,125 9,866,421 7.97 8.63 3.57 3.64 Source: Michigan Travel Counts Surveys. Table 4.5. Michigan TCS rural versus urban household trip rates.
52 Long-Distance and rural travel transferable parameters for Statewide travel Forecasting Models separately for small cities, upper peninsula, northern lower peninsula, and southern lower peninsula. With the exception of the more heavily populated southern lower peninsula, non- urbanized households exhibit lower weighted trip rates than urban households. This finding is somewhat contrary to the 2009 NHTS analysis in Appendix G, unless most of the Michigan urbanized household surveys were conducted in suburban settings, since suburban house- holds showed higher trip rates than rural households in the 2009 NHTS. It is also worth noting that the differences found among nonurbanized areas of Michigan might indicate limitations to transferability. Recent and Ongoing GPS Surveys in the United States Table 4.6 depicts the split between weekend and weekday travel from recent GPS surveys described earlier in Section 2.3 of this Guidebook. These splits, after survey expansion, are simi- lar in each survey. In all cases, as expected, the percent of weekend trips is highest for the long- distance trips (most 30 percent or higher) when compared against urban and rural travel. Table 4.7 depicts four different trip/demographic measurements for each of the four surveys and overall, for the same three different geographic definitions found in the prior two tables. Daily trip production rates for long-distance trips are low, as expected. Rural trip production rates are substantially higher than urban rates for these four surveys, although the rates are comparable to analysis of 2009 NHTS, as described later in this chapter. Long-Distance Rural Urban Weekday Weekend Weekday Weekend Weekday Weekend Overall 68% 32% 77% 23% 79% 21% Atlanta 66% 34% 75% 25% 79% 21% Denver 72% 28% 78% 22% 80% 20% Massachusetts 68% 32% 86% 14% 88% 12% Chicago 64% 36% 73% 27% 75% 25% Source: Geostats based on recent GPS-based travel surveys. Table 4.6. Travel day statistics from recent GPS-based surveys. Number of Trips Trip Production Rate Average Household Size Average Number of Vehicles/HH L on g D is ta n ce R u ra l U rb an A ll T ri p s L on g D is ta n ce R u ra l U rb an L on g D is ta n ce R u ra l U rb an L on g D is ta n ce R u ra l U rb an Overall 1,253 31,689 103,832 6.20 0.04 9.39 5.23 2.85 2.86 2.75 2.23 2.18 2.05 Atlanta 580 16,932 48,098 5.92 0.03 8.24 5.03 2.89 2.85 2.77 Denver 395 9,836 31,377 6.11 0.04 9.42 4.85 2.77 2.81 2.68 2.22 2.16 2.07 Massachusetts 176 3,349 10,325 5.90 0.04 13.56 5.04 2.92 2.96 2.82 2.27 2.21 2.02 Chicago 102 1,572 14,032 7.89 0.05 8.86 7.05 Source: Geostats based on recent GPS-based travel surveys. Table 4.7. Trips and households from recent GPS-based surveys.
trip Generation parameters and Benchmark Statistics 53 2010 Travel Survey of Residents of Canada As described in Appendix B, the Travel Survey of Residents of Canada (TSRC) is designed to measure the size and status of Canadaâs tourism industry at the national level. Through direct contact with Canadian officials, the research team was able to obtain a spreadsheet data analysis of the 2010 TSRC. Without direct access to the data, which would have required additional budget for purchasing data, this study was limited to information provided in this spreadsheet. Table 4.8 depicts the percent of long-distance trips by purpose, with single-day travel sepa- rated from overnight travel. Although the trip purposes used in the TSRC are different from those found in the ATS, business-related trips are considerably less in the TSRC, at slightly more than 5 percent, versus the ATS at 22 percent. These statistics are from fully weighted survey data; however, without additional analysis, it is unclear whether the lower percent is a function of sampling or that long-distance business travel is considerably less common than in the United States. 4.2 Analytical Approach to Estimating Long-Distance and Rural Trip Generation Parameters and Benchmarks One key to implementing the analytical plan and developing transferable parameters was to obtain access to all datasets from the American Travel Survey (ATS) and identify trip purposes, average trip lengths, vehicle occupancies, and other statistics typified by long-distance travelers. The 1995 ATS datasets are dated; however, these data are the only long-distance data that provide statistically sound estimates of long-distance travel in and between the states. Although the 2001 National Household Travel Survey (NHTS) had a long-distance compo- nent, this survey did not have sufficient samples to calculate estimates of long-distance travel for most states (New York and Wisconsin were exceptions to this, because of the large Add-On in the former and stratified sampling of the latter, although neither Add-On was included in the official 2001 NHTS long-distance file). The approach to using NHTS 2001 data was based on discussions with FHWA NHTS support staff, both past and present, as well as members of the research team with extensive experience using different versions of the NHTS. All of these dis- cussions pointed to concerns over the use of NHTS 2001 for long-distance trips and at least some Trip Duration: Total â Domestic Travel (Age 18+): Person Trips with the Destination in Canada *** Row Percents *** Main Trip Purpose: Standard person trip stub variables Total Pleasure, Vacation, Holiday Visiting Friends or Relatives Business and All Conferences or Conventions Shopping and Other >>> Final Data <<< Total Long-Distance Trips: 100.00% 36.99% 46.72% 5.40% 10.90% Single-Day Long-Distance Trips 100.00% 34.43% 45.59% 5.14% 14.84% Overnight Long-Distance Trips 100.00% 40.79% 48.41% 5.77% 5.03% Source: Travel Survey of Residents of Canada. Table 4.8. Canadian residentsâ long-distance trips by percent purpose.
54 Long-Distance and rural travel transferable parameters for Statewide travel Forecasting Models of these concerns are documented elsewhere in this report. All of the NHTS 2001 long-distance data, including state Add-On samples, were made available for use by the research team as well. These two long-distance datasets can be used together, yet separately, since the 2001 ques- tionnaire relied heavily on the 1995 ATS as a template. Definitional categories for mode and purpose are comparable. The study team also obtained readily available state DOT survey data and documentation from statewide household travel surveys for Michigan and Ohio. The research team also coordinated with Canadian officials to identify available long-distance travel parameters readily available from their recent household travel surveys. Finally, recent travel surveys using Global Positioning Systems (GPS) were mined for parameters on long-distance travel as well as rural parameters. Transferable rural travel parameters largely focused on the 2009 NHTS and its state Add-On surveys. Analysis of variance (ANOVA) and other statistical tests were run on 2009 NHTS data in an attempt to identify which available attributes best explain differences in rural trip-making and whether certain parameters should be stratified for different conditions such as urban clusters and proximity to urbanized area boundaries. Existing statewide models also played a significant role in this analytical plan, in terms of quantifying reasonableness ranges against which to compare resulting ATS/NHTS survey-based model parameters. Also, documented model parameters were identified for potential transfer- ability to other statewide models, based on the characteristics of the state where the data were collected versus the state to which a parameter might be proposed for transferability. Inter- regional or intercity travel components are included in some statewide models to capture both intrastate and interstate trips. The core model design feature is the recognition that interregional travel is very different from urban area travel, where different sets of explanatory variables or different sensitivities to levels of service are involved. A set of typical long-distance and rural trip purposes was established from this analysis so that model parameters could be stratified by such categories and reasonableness benchmarks could be established for percent trips by purpose. Mean trip length statistics, both in miles and min- utes, also were estimated from the survey databases for use as benchmarks in future statewide model validation efforts; however, the survey analysis for this study did not include the calculation of state-by-state trip lengths. As discussed previously, statewide models and travel surveys have used a range of thresholds to define long-distance trip-making. Most sources cited in this study used either 50, 75, or 100 miles as the minimum threshold for trips to be considered âlong-distance.â In an effort to maximize the number of long-distance trip samples, this report looks at model parameters at three different long-distance trip thresholds: 50â100 miles, 100â300 miles, and more than 300 miles. By separat- ing out 50â100 mile trips from 100â300 miles, this allows for differentiation of long-distance trips by the two most common thresholds, beginning and ending at 100 miles. The rationale for using 300 miles as another cutoff point is that preliminary data analysis indicated a mode shift from personal auto to air travel at this distance. The remainder of this section of the chapter on trip generation focuses on the data sources used for parameter estimation, along with some general comparisons among sources. American Travel Survey (ATS) As stated elsewhere, the 1995 ATS is still seen as the most robust sample of long-distance travel behavior, in spite of its age. The ATS was entirely focused on long-distance travel, unlike the 2001 NHTS, which also surveyed typical daily urban and rural travel patterns. There are numerous ways to analyze the data. For this study, household frequencies and statistical means were cal- culated separately for all households and per capita as well. Trip rates and frequencies also were
trip Generation parameters and Benchmark Statistics 55 calculated separately for annual and daily conditions. The reason for this is that long-distance trips are not an âevery dayâ occurrence for most households. Trip rates were initially calculated on an annual basis using the ATS and then divided by 365 to provide daily trip rates as an option for users of this report. Since the ATS reported annual trips, long-distance trip characteristics in this Guidebook are likewise summarized as annual trips. Another consideration was whether to include weekdays and/or weekends, of which both were calculated. According to available documentation on the ATS âeach trip was classified as a weekend trip or as not a weekend trip. A weekend trip is a trip of one to five nights, includ- ing a Friday and/or Saturday night stay. Travelers who stay one or two nights away, including a Friday or Saturday night are defined as regular weekend travelers. Those who stay three to five nights away, including a Friday and/or Saturday night stay are defined as long weekend travelersâ (http://www.bts.gov/publications/1995_american_travel_survey/an_overview_ of_the_survey_design_and_methodology/index.html). Based on this description it was not practical to summarize only weekday trips because some of the âweekendâ trips were partially âweekdayâ trips. Furthermore, analysis of weekday-only trips resulted in a dramatic drop in long-distance trip rates that would be inconsistent with results from other long-distance surveys. Therefore, long-distance trip statistics found in this report include both weekday and weekend trips. Cross-classification was used to evaluate different attributes against one another, as well as to calculate trip rates based on socioeconomic characteristics. The latter used household size by income, which is consistent with one cross-classification scheme used and documented in the previously referenced NCHRP Report 716 on urban transferable parameters. It is important to note that the ATS did not include trips of 50â100 miles in length, and so there are no 50- to 100-mile trips in the ATS statistical tables in this chapter. Statistics reported in the next section on parameters from the 2001 NHTS do include 50- to 100-mile trips, consistent with the lower long-distance trip threshold used in that survey. Statistics for 50- to 100-mile trips are presented only for analysis of the 2001 NHTS survey. Table 4.9 is an assessment of household trip rates by trip purpose and the relevant trip dis- tance categories noted earlier. As shown in this table, a typical household generates 10.15 trips of over 100 miles, or 0.0278 daily long-distance trips (annual trip rate divided by 365 days). Trips were grouped into three purposes: business, pleasure, and personal business. Pleasure trips had the highest average trip rate for all three distance categories while the majority of trips were 100â300 miles for all trip purposes. Table 4.10 compares trip rates by household income level and mileage range. A review of this table shows that the highest-income group has the greatest long-distance trip rate for both mile- age categories. Trip rates show that the propensity of making long-distance trips, and the length of those trips, has a lot to do with household income. Purpose -300 miles > 300 miles Total 01 Business 1.37 0.91 2.28 02 Pleasure 4.08 2.13 6.21 03 Personal Business 1.19 0.47 1.66 Total 6.64 3.51 10.15 Source: 1995 ATS. Table 4.9. ATS annual trip rates by distance/purpose, round-trip.
56 Long-Distance and rural travel transferable parameters for Statewide travel Forecasting Models Table 4.11 provides information from the 1995 ATS on weekday versus weekend long-distance travel based on three trip purposes. Not surprisingly, business and personal business trips are less likely to occur on weekends when compared to pleasure trips, of which over 50 percent involve weekend travel. As noted earlier, some portion of the âweekendâ trips in fact take place on weekdays, and so the term ânot weekendâ was chosen instead of âweekday.â Simply put, the ânot weekendâ trips are those that take place entirely on weekdays, with no portion of the long- distance trip taking place on a weekend. Table 4.12 presents the number of long-distance intermediate stops by trip/tour purpose. More than 90 percent of long-distance trips did not include any stops. The percent of intermedi- ate stops is highest for business trips and lowest for personal business trips. The trip, including all intermediate stops, could be analogous to the concept of a trip tour. 2001 National Household Travel Survey The 2001 NHTS database also was used to develop long-distance model parameters and benchmarks similar to those produced using the 1995 ATS. A primary reason for developing these statistics using the 2001 NHTS was to overcome concerns about the age of the 1995 ATS data. Use of the 2001 NHTS was considered acceptable for long-distance analysis because this survey included a targeted sample of long-distance trips, unlike the more recent 2009 NHTS database. Unfortunately, there are several shortcomings with the 2001 long-distance survey com- ponent, including the following: ⢠Much lower response rate using a telephone survey approach for the 2001 NHTS versus the panel survey approach used in the 1995 ATS; ⢠Shorter recall period of the 2001 NHTS also resulted in a much smaller sample size of long- distance trip-makers (45,000 in 2001 NHTS versus 550,000 in 1995 ATS); Income -300 miles > 300 mi Total 01 $0-$24,999 2.97 1.30 4.27 02 $25,000-$99,999 8.48 4.34 12.82 03 $ 100,000+ 13.78 12.49 26.26 Total 6.64 3.51 10.15 Source: 1995 ATS. Table 4.10. ATS annual trip rates by distance/income, round-trip. Purpose Not Weekend Weekend Total Percent Weekend 01 Business 85,261 33,910 119,171 28.45% 02 Pleasure 156,188 159,595 315,783 50.54% 03 Personal Business/Other 67,097 33,469 100,566 33.28% Total 308,546 226,974 535,520 Trip % 58% 42% 100% Source: 1995 ATS. Table 4.11. ATS annual frequency by purpose/weekend trip, round-trip.
trip Generation parameters and Benchmark Statistics 57 ⢠The impacts of 9/11 on travel resulted in a much lower share of air travel in the 2001 NHTS when compared against the 1995 ATS; and ⢠Thresholds used to define long-distance trips differ between the two surveys with 1995 ATS defined as 100 miles or greater and 2001 NHTS as more than 50 miles. Chapter 2 of this Guidebook described the latter difference as a potential strong point of NHTS 2001 as a way of obtaining information on these mid-range 50â100 mile trips. Analysis of 2001 NHTS long-distance trip data showed a sizeable sample of 50â100 mile trips (21,500 out of 45,000 trips, or nearly 48 percent of the 2001 NHTS long-distance sample), such that exclusion of these trips for NHTS 2001 statistics would be problematic. Hence, 50â100 mile trips were included for NHTS 2001 analyses. Table 4.13 depicts annual long-distance household trip rates from the 2001 NHTS, including 50â100 mile trips. The overall trip rate (excluding the 50â100 mile trips) is 12.32, about 21 percent higher than the 1995 ATS trip rate of 10.15. The patterns within the cross-classification table are relatively similar between the two surveys (i.e., which cells have higher or lower rates than others). It is worth noting that the percentages of trips by purpose are somewhat similar between the two long-distance surveys, as follows: ⢠Businessâ28.38 percent for NHTS 2001 versus 22.25 percent for ATS; ⢠Pleasureâ54.84 percent for NHTS 2001 versus 58.97 percent for ATS; and ⢠Personal Businessâ16.78 percent for NHTS 2001 versus 18.78 percent for ATS. Rural Typologies Identification of rural travel parameters took a different focus than long-distance travel param- eters. First, rural trip-making data are well represented in the recent 2009 NHTS. Therefore, the Purpose 2 3 4 Total Percent Stop/ Purpose 01 Business 106,212 2,059 7,515 3,385 119,171 10.87% 02 Pleasure 293,727 8,243 8,412 5,401 315,783 6.98% 03 Personal Business 94,888 2,470 1,998 1,210 100,566 5.65% Total 494,827 12,772 17,925 9,996 535,520 7.60% Percent Stops by Number 92.40% 2.38% 3.35% 1.87% 100.00% Source: 1995 ATS. Table 4.12. Annual frequency by stops from destination/purpose, round-trip. Purpose 50-100 Miles 100-300 Miles > 300 Miles Total 01 Business 4.04 1.85 0.97 6.85 02 Pleasure 5.71 5.08 2.39 13.17 03 Personal Business/Other 1.78 1.52 0.52 3.83 Total 11.53 8.45 3.87 23.85 100+ Mile Trip Rate 12.32 Source: 2001 NHTS. Table 4.13. 2001 NHTS annual trip rates by distance/purpose.
58 Long-Distance and rural travel transferable parameters for Statewide travel Forecasting Models research team was able to focus primarily on this one survey database, unlike the multiple and considerably older survey databases used to identify long-distance travel parameters. Second, the points of reference are quite different for rural trips. Long-distance travel characteristics were generally summarized by different trip length categories, whereas rural travel parameters required establishing typologies for classification and comparison against comparable statistics on travel in urbanized areas. Finally, the temporal issues for rural travel are not as complex as long-distance trips. For example, the database does not deal with international travel or multiple stops, and the greater share of travel is on the weekdays, with a much smaller share of weekend travel than with long-distance trips. The first step in the assessment of rural travel parameters was the identification of rural typolo- gies and an exploration of how these different typologies can be used to describe the trip-making of rural households. This also includes the need to define what is and is not rural travel and how typical rural travel behavior differs from that in more urban settings. These efforts started with a focus on attributes contained within the NHTS 2009 âDOT versionâ of the database, including the Claritas attributes described earlier in this Guidebook. The following similar, yet not identical, attributes from the 2009 NHTS DOT version were used to identify potential rural typologies: ⢠URBANâIdentifies whether or not the home address is located in an urban area, typically defined as a concentrated area with a population of 50,000 or greater; ⢠URBRURâIdentifies whether or not the home address is located in a rural area; ⢠URBANSIZEâPopulation size of the urban area in which the home address is located; ⢠HBHURâUrban/Rural Indicator, appended to the NHTS by Claritas (http://nhts.ornl. gov/2009/pub/UsersGuideClaritas.pdf)âthis classification reflects the population density of a grid square into which the householdâs block falls; ⢠HBRESDNâThe number of housing units per square mile by block group; and ⢠HBPOPDNâThe population per square mile by block group. Additionally, the rural typologies recommended as part of NCHRP Project 25-36, âImpacts of Land Use Strategies on Travel Behavior in Small Communities and Rural Areasâ and described earlier also were considered in this effort. The four typologies recommended by NCHRP Proj- ect 25-36 were as follows, along with the study definitions of each, as quantified by âcommuting zonesâ developed by the USDAâs Economic Research Service: ⢠Population DensityâComputed as number of people divided by unit area of developed or developable land; ⢠Road DensityâCalculated as road length in miles per square mile of developed or develop- able land; ⢠Land-Use MixtureâA proxy of land-use mixture measuring how residents, jobs, and other activities are distributed in relation to each other; and ⢠Variation in Population DensityâVariation in population density distinguished where most residents are located in a relatively small set of concentrated areas at relatively high densities from locations where residents are spread more evenly. This project did not pursue full consideration of commuting zones, which are defined in NCHRP Project 25-36 as âmulticounty regions that convey the typical pattern of commuting trips in a spatially defined labor market: a much higher proportion of commuting trips have origins and destinations that are both inside the zone than those trips for which one end is outsideâ (Department of City and Regional Planning Center for Urban and Regional Studies, University of North Carolina at Chapel Hill, 2011). In place of data on commuting zones, the analysis presented here uses readily available data to simulate some of these typologies. Population Density already was an attribute included in
trip Generation parameters and Benchmark Statistics 59 the 2009 NHTS dataset so it was easily addressed. Road Density was calculated using the 2005 National Highway Planning Network and geographic information systems (GIS) tools, based on a simple formula of Road Length/Census Tract Area. The resulting Road Density was a con- tinuous variable, so a regression analysis was conducted and then the variable was re-coded as a categorical variable. There was no practical way to simulate land-use mixture or the variation in population density using the data readily available for this project. One additional typology analyzed was âurban proximityâ because the NCHRP Project 8-84 research team thought that the proximity to urban areas could impact the number and purpose of trips. Latitude/longitude address information was not stored for each household in the 2009 NHTS DOT database, which is necessary for accurate depiction in GIS. The database did have Census tract and block group information, and this information was appended to an NHTS Census tract/block group shapefile. Once the 2009 NHTS DOT database was joined to the NHTS CT/BG shapefile by a block group ID number, the households were spatially referenced to the block group. Figure 4.1 depicts a map of concentric rings formed during the proximity analysis, zoomed into north Florida/south Georgia, as an example. In cases where a block group was in proximity to multiple urban areas, distance to the closest urban area was applied. Unfortunately, Proximity to Urban Area did not show any clear trip rate trend, and so the analysis focused on the other measures. As noted previously, while it would have been ideal to use a national land coverage database to identify subcategories of rural areas such as exurban, agricultural, and recreational, the research Figure 4.1. Example map depicting proximity to urban area.
60 Long-Distance and rural travel transferable parameters for Statewide travel Forecasting Models team had concerns over how to define and classify rural areas. It was thought that proximity to urbanized area, residential density, and roadway density allowed for a more objective classification of rural households. In order to narrow the number of rural typologies used in recommending transferable rural parameters, analysis of variance (ANOVA) and t-tests were conducted on each of the typolo- gies discussed below. The trending of trip rates up or down in relation to different settings for each attribute also was reviewed to further ascertain the explanatory power of each typology variable. In some cases, the number of categories was narrowed to assess the viability of each. Appendix G includes a separate page for each typology variable, along with t-test values for each category, analytical and trend observations, and revisions to the number of categories for each attribute. Four typologies were subsequently recommended for the purposes of calculating transferable rural trip production rates. These four typology variables were as follows: 1. HBHURâUrban/Rural Indicator reflecting population density of a grid square; 2. URBANâWhether or not the home address is located in an urban area; 3. URBRURâWhether or not the home address is located in a rural area; and 4. HBRESDNâNumber of housing units per square mile by block group. Table 4.14 depicts how these typology attributes could interact and be cross-classified into three dimensions for trip generation, focusing on the first three attributes to deal with different geog- raphies and the latter, housing units per square mile (HBRESDN), being applied against all rural and urban categories. The number of 2009 NHTS household samples by row and column also is provided in parentheses. Cells with âN/Aâ represent combinations of three attributes that should not exist. For example, rural areas should not also be classified into suburban, second city, or urban. This approach is further refined into a set of trip production rates cross-classified by socio- economic household characteristics, and specified by trip purpose, as described in a later section of this chapter. 2009 National Household Travel Survey With its large sample size of rural households, the 2009 NHTS was the principal source of rural travel model parameters described in this study. Based on the rural typology analysis described in the previous section, four trip production cross-classification matrix sets were prepared. As with long-distance rates, socioeconomic data used in the cross-classification scheme are income (three categories) by household size (five categories). Each set includes separate trip rates for home-based work, home-based nonwork, and nonhome-based purposes, as well as separate rates for each substrata included with the typology. The substrata for each cross-classification set are depicted below (the underlined strata reflect the rural components/subsets of each set): ⢠HBHURâTown and Country, Suburban Areas, Secondary City, Urban All; ⢠URBANâNot in Urbanized Area, Urbanized Area; ⢠URBRURâIn Rural Area, In Urban Area; and ⢠HBRESDNâLow Density (0â999 units/square mile), Medium Density (1,000â9,999 units/ square mile), High Density (10,000+ units/square mile). Although the definition of what is predominantly rural changes from one attribute/set to another, if the underlined categories above are compared, there is little difference in the trip rates. For example, the total number of person trips per household for all trip purposes is 9.72 for URBRUR, 9.83 for URBAN, and 9.56 for HBHUR. A more significant difference is shown using HBRESDN with a total trip rate of 11.76, although it is not clear how much of this lower housing unit density category consists of rural households. Appendix H depicts trip rates for
trip Generation parameters and Benchmark Statistics 61 URBRUR URBAN HBRESDN Housing units per sq mile â Block group 0-999 (75,937) 1,000-9,999 (52,450) 10,000-999,999 (2,120) Household in urban/rural area Home address in urbanized area Rural (38,014) Not in an urban area (38,014) (In an urban cluster â placeholder) N/A N/A N/A Urban (92,493) In an urban area (79,569) In an area surrounded by urban areas (51)a In an urban cluster (12,873) Household in urban/rural area Urban/Rural indicator â Block group Rural (38,014) Town and Country (38,014) Suburban (N/A) N/A N/A N/A Second City (N/A) N/A N/A N/A Urban (N/A) N/A N/A N/A Urban (92,493) Town and Country (24,227) N/A N/A N/A Suburban (30,491) Second City (23,550) Urban (14,225) Size of urban area in which home address is located Urban/Rural indicator â Block group Not in an urbanized area (50,938) Town and Country (50,938) Suburban (N/A) N/A N/A N/A Second City (N/A) N/A N/A N/A Urban (N/A) N/A N/A N/A All other categories combined â AKA urbanized (79,569) Town and Country (11,303) N/A N/A N/A Suburban (30,491) Second City (23,550) Urban (14,225) Note: Numbers in parentheses represent 2009 NHTS sample sizes for each category. Sample size numbers were found to be somewhat inconsistent among different urban/rural attributes and categories. âN/Aâ reflects an attribute combination that does not exist/would be illogical. a Probably should merge with âIn an urban areaâ due to small sample size. Table 4.14. Recommended rural typology variables.
62 Long-Distance and rural travel transferable parameters for Statewide travel Forecasting Models each category of every variable analyzed for estimating transferable trip production rates. The tables in Appendix H include both rural and urban trip rates using definitions unique to each attribute/rate set. 4.3 Long-Distance Trip Generation Model Parameters For the purposes of recommending transferable long-distance parameters, it was decided to focus on the 1995 ATS due to its larger sample size and based on the similarity of trip frequencies by purpose between the ATS and 2001 NHTS long-distance component. Although there are a multitude of ways these parameters can be summarized, this report uses the following considerations in reporting long-distance model parameters: ⢠Include all days of the week (weekends and weekdays); ⢠Report parameters on an annual (rather than daily) basis; ⢠Exclude trips less than 100 miles in length; ⢠Limit analysis to domestic travel (no international trips); and ⢠Report at the household level rather than person level (per capita rates). The primary reason for including weekday and weekend travel is that ATS weekend travel includes weekday trips that include a weekend component. Furthermore, average annual daily traffic (AADT), by definition, includes both weekdays and weekends. Annual, rather than daily, trips are reported because this is how ATS trips were reported. Trips less than 100 miles in length were excluded because the ATS did not include these trips. International travel was excluded because these trips are not included in most statewide passenger models and these trips tend to skew trip length. Finally, household, rather than per capita, trip rates were selected because these are more commonly found in four-step travel demand models. All transferable parameters are calculated for the predominant three trip purposes (Business, Pleasure, and Personal Business) and total trips (All Purposes). The transferable parameters are described below and in subsequent chapters by model step. Trip Generation: Long-Distance Person Trip Production Rates For transferable long-distance trip production rates, it was decided to cross-classify socio- economic characteristics of each household in a comparable manner to NCHRP Report 716. The recommended cross-classification scheme for long-distance trip production rates is household income by household size. The correlation between income and long-distance trip-making is significant. For the purposes of cross-classification, household size is stratified into five categories, similar to NCHRP Report 716, whereas household income was collapsed into three categories. It also was decided to report annual trip rates since daily and monthly trip rates resulted in very low values. Table 4.15 depicts recommended long-distance person trip production rates for the three trip purposes. Since this study is primarily focused on passenger travel, the reader should refer to NCHRP Report 716 for a summary of sample truck trip generation rates derived from multiple sources. Appendix E of this report also provides metrics on rural versus urban truck travel. 4.4 Rural Trip Generation Model Parameters All rural travel parameters summarized in this section of the report were derived from sta- tistical analysis of 2009 NHTS datasets. As with long-distance parameters, the rural parameter discussion is divided into separate chapters reflecting each step in the typical model chain.
trip Generation parameters and Benchmark Statistics 63 Trip Generation: Rural Trip Production Rates From each of the four trip rate stratifications described in Section 4.2, URBAN showed the fewest trip rate anomalies (cells having higher or lower trip rates than expected compared to adjacent cells). Table 4.16 depicts unchained rural trip rates using URBAN as the 2009 NHTS attribute to differentiate between rural and urban households. Only two minor anomalies were identified in this table. HBW trip rates for highest-income four-person households and NHB 5+ person households were initially lower than found in adjacent trip rate cells (those with lower-income or household size). Rates were subsequently adjusted and smoothed for these two cells. The resulting total person trip rate per rural household using the URBAN attribute is 10.06, as opposed to an urbanized area trip rate of 9.91 as depicted in Appendix H. Urbanized area trip rates do vary by subcategory, such as secondary cities (9.50), suburban (10.34), and non suburban or second city urbanized (9.36). Some of these differences could possibly be minimized through testing of alternate socioeconomic cross-classification schemes. Trip rate comparisons found in Appendix G show a strong correlation between housing density and trip rates, with lowest hous- ing density trip rates being the highest at 9.60 and highest density housing trip rates the lowest at 7.77. In theory, opportunities for mixed-use development are more prevalent in higher density areas, thus reducing the trip rate, as opposed to lower density areas where mixed uses are less common, resulting in more trip-making to satisfy household needs. It is also possible that some of these differences might be explained by differences in household size, with testing of alterna- tive cross-classification schemes. Income by HH Size 1 2 3 4 5+ Total Business Trip Rates by Household Size/Income 01 <$25,000 0.44 0.80 0.88 0.99a 1.59 0.66 02 $25,000-$99,999 2.34 2.62 2.97 3.24 3.80 2.90 03 $100,000+ 3.70 8.00a 8.20 a 8.40 a 8.54 8.61 Total 0.99 2.35 2.70 3.24 3.51 2.28 Pleasure Trip Rates by Household Size/Income 01 <$25,000 1.70 3.49 3.50 a 5.10 5.15 2.77 02 $25,000-$99,999 3.88 6.70 8.42 9.77 11.93 7.84 03 $100,000+ 4.76 11.51 14.15 18.91 21.27 14.59 Total 2.32 6.03 7.48 9.46 10.77 6.21 Personal Business Trip Rates by Household Size/Income 01 <$25,000 0.41 1.12 1.16 1.51 2.05 0.84 02 $25,000-$99,999 1.03 1.63 2.15 2.66 3.75 2.08 03 $100,000+ 0.46 2.56 2.78 3.99 4.79 3.07 Total 0.58 1.53 1.94 2.53 3.38 1.66 Total Annual Trip Rates by Household Size/Income 01 <$25,000 2.54 5.41 5.48 7.28 8.79 4.27 02 $25,000-$99,999 7.25 10.95 13.54 15.67 19.48 12.82 03 $100,000+ 8.92 22.87 24.72 34.03 34.60 26.26 Total 3.89 9.91 12.12 15.22 17.66 10.15 a Indicates where estimated trip rates were manually adjusted and smoothed. Table 4.15. Annual long-distance person trip production rates.
64 Long-Distance and rural travel transferable parameters for Statewide travel Forecasting Models Cross-classification matrices are also provided for auto availability and number of workers, con- sistent with cross-classification schemes documented in NCHRP Report 716. Rural trip production rates for auto availability by household size are found in Table 4.17, while number of workers by household size are depicted in Table 4.18. Overall, Table 4.17 (auto availability) has slightly lower total weekday trip rates than Table 4.16 (income). There are only three instances where the reverse is true, households with 5+ members for all purposes and NHB; and households with 3 members for HBW. Whereas Table 14.12 produced four trip rate anomalies, there are only two small anomalies in Table 14.13, depicted in underlined italics. HH Person Trip Rates: In Other (Rural) Areas, All Trip Purposes Trip Rates HH Size Income 1 2 3 4 5+ Total Less than $25,000 2.8 6.4 9.9 15.0 15.6 6.8 $25,000-$99,999 4.2 7.9 12.8 17.5 22.1 10.2 Above $100,000 5.1 8.8 14.0 20.1 26.2 14.0 Total 3.6 7.8 12.5 18.0 20.9 10.0 In Other (Rural) Areas HBW Trip Rates HH Size Income 1 2 3 4 5+ Total Less than $25,000 0.2 0.8 1.3 1.5 1.7 0.7 $25,000-$99,999 0.7 1.2 2.1 2.4 2.5 1.5 Above $100,000 0.9 1.6 2.3 2.4a 2.6 1.9 Total 0.5 1.2 2.0 2.2 2.3 1.4 In Other (Rural) Areas HBNW Trip Rates HH Size Income 1 2 3 4 5+ Total Less than $25,000 1.6 3.8 5.9 9.7 10.8 4.2 $25,000-$99,999 1.9 4.0 6.8 10.1 13.8 5.6 Above $100,000 2.2 4.0 7.2 11.4 15.2 7.4 Total 1.8 4.0 6.7 10.4 13.1 5.6 In Other (Rural) Areas NHB Trip Rates HH Size Income 1 2 3 4 5+ Total Less than $25,000 0.8 1.7 2.6 3.8 4.0a 1.7 $25,000-$99,999 1.5 2.6 3.9 4.9 5.7 3.1 Above $100,000 1.9 3.1 4.4 6.4 8.3 4.6 Total 1.3 2.5 3.8 5.2 5.4 3.0 Source: 2009 NHTS. a Indicates where estimated trip rates were manually adjusted and smoothed. Table 4.16. Rural person trip production rates: HH size by income, URBAN attribute identifies rural HHs.
trip Generation parameters and Benchmark Statistics 65 In Other (Rural) Areas, All Trip Purposes Trip Rates HH Size Autos/HH 1 2 3 4 5+ Total 0 Veh 2.3 6.2 9.1 12.0 13.5 4.9 1 Veh 3.8 6.9 11.4 14.4 15.8 6.4 2 Veh 4.6 7.9 12.4 18.2 21.4 11.8 3+ Veh 4.6 8.1 13.8 19.5 25.1 15.3 Total 3.6 7.6 12.4 17.8 20.9 9.7 In Other (Rural) Areas HBW Trip Rates HH Size Autos/HH 1 2 3 4 5+ Total 0 Veh 0.2 0.7 1.2 1.1 1.2* 0.5 1 Veh 0.5 0.8 1.2 1.5 1.4 0.8 2 Veh 0.6 1.4 2.0 2.1* 2.2 1.6 3+ Veh 1.0 1.5 2.6 3.0 3.3 2.5 Total 0.5 1.2 2.0 2.2 2.3 1.3 In Other (Rural) Areas HBNW Trip Rates HH Size Autos/HH 1 2 3 4 5+ Total 0 Veh 1.3 3.7 5.7 7.9 9.9 3.1 1 Veh 1.8 3.8 6.7 9.0 11.1 3.6 2 Veh 2.2 3.9 6.5 10.7 13.4 6.5 3+ Veh 2.3 3.9 7.1 10.8 14.6 8.3 Total 1.7 3.9 6.7 10.4 13.1 5.4 In Other (Rural) Areas NHB HH Size Autos/HH 1 2 3 4 5+ Total 0 Veh 0.7 1.7 2.2 2.9 3.0* 1.2 1 Veh 1.3 2.2 3.4 3.8 3.9* 2.0 2 Veh 1.7 2.5 3.8 5.4 5.7 3.6 3+ Veh 1.2 2.6 4.0 5.7 7.0 4.5 Total 1.2 2.4 3.7 5.2 5.4 2.9 Source: 2009 NHTS. * Indicates where estimated trip rates were manually adjusted and smoothed. Table 4.17. Rural person trip production rates: HH size by auto availability, URBAN attribute identifies rural HHs.
66 Long-Distance and rural travel transferable parameters for Statewide travel Forecasting Models In Other (Rural) Areas, All Trip Purposes Trip Rates HH Size HH Size 1 2 3 4 5+ Total 0 worker 2.796 5.863 8.456 11.721 12.494 4.952 1 worker 4.249 7.436 11.247 15.733 18.245 8.750 2 worker 0.000 9.438 14.136 19.545 23.806 14.610 3+ worker 0.000 0.000 16.316 23.564 27.678 22.544 Total 3.605 7.607 12.452 17.868 20.962 9.783 In Other (Rural) Areas HBW Trip Rates HH Size HH Size 1 2 3 4 5+ Total 0 worker 0.003 0.011 0.009 0.049 0.074 0.010 1 worker 0.966 1.148 1.298 1.460 1.500* 1.166 2 worker 0.000 2.403 2.752 2.665 2.688 2.580 3+ worker 0.000 0.000 4.993 4.808 5.414 5.063 Total 0.539 1.211 2.026 2.226 2.342 1.378 In Other (Rural) Areas HBNW Trip Rates HH Size HH Size 1 2 3 4 5+ Total 0 worker 1.931 4.088 5.603 8.807 9.688 3.483 1 worker 1.691 3.914 6.650 9.646 11.975 4.878 2 worker 0.000 3.825 7.125 11.152 15.113 7.655 3+ worker 0.000 0.000 6.637 11.773 14.417 10.963 Total 1.797 3.937 6.700 10.434 13.139 5.453 In Other (Rural) Areas NHB HH Size HH Size 1 2 3 4 5+ Total 0 worker 0.862 1.764 2.844 2.865 2.900* 1.459 1 worker 1.593 2.374 3.299 4.628 4.832 2.706 2 worker 0.000 3.211 4.259 5.728 6.005 4.376 3+ worker 0.000 0.000 4.687 6.983 7.847 6.518 Total 1.269 2.459 3.726 5.209 5.482 2.951 Source: 2009 NHTS. * Indicates where estimated trip rates were manually adjusted and smoothed. Table 4.18. Rural person trip production rates: HH size by number of workers, URBAN attribute identifies rural HHs.
trip Generation parameters and Benchmark Statistics 67 The percentage of rural trips by purpose could be a useful statistic for use in model valida- tion and reasonableness checking. Assuming the weighted number of surveys for 2009 NHTS adequately reflects the share of rural versus urban trips, the percentage of rural trips by purpose has been summarized in Table 4.19. Since the definition of rural varies somewhat from one NHTS attribute/typology to another, the number and percentage of trips by purpose was esti- mated for each of these typologies and subsequently averaged. Regardless of the attribute used to identify rural households, the results show a considerably smaller percentage of home-based work trips than commonly found in urban areas. This is not entirely surprising in a population that generally consists of a higher-than-average share of farmers, retirees, and unemployed, as well as above average household sizes (http://205.254.135.7/emeu/recs/recs2005/hc2005_tables/ hc3demographics/pdf/tablehc8.3.pdf). Typology/NHTS 2009 Attribute URBANR â Other (Not Urbanized) HBHUR â Town and Country HBRESDEN â 0-999 Units/ Square Mile URBRUR â Rural Areas Average Trip Purpose No. of Trips % No. of Trips % No. of Trips % No. of Trips % % Rural Home- Based Work 63,057 11.82 12.03 23,194 12.60 29,983 12.26 12.06 Rural Home- Based Nonwork 308,005 57.74 218,398 54.41 96,301 52.31 129,875 53.09 55.19 Rural Nonhome- Based 162,405 30.44 134,711 33.56 64,619 35.10 84,761 34.65 32.74 Non Urban Totals â All Purposes 533,467 100.00 401,388 100.00 184,114 100.00 244,619 100.00 100.00 Source: 2009 NHTS. Table 4.19. Rural trips by purpose.
TRB’s National Cooperative Highway Research Program (NCHRP) Report 735: Long-Distance and Rural Travel Transferable Parameters for Statewide Travel Forecasting Models explores transferable parameters for long-distance and rural trip-making for statewide models.
Appendixes G, H, and I are not contained in print or PDF versions of the report but are available online. Appendix G presents a series of rural typology variables considered in stratifying model parameters and benchmarks and identifies the statistical significance of each. Appendix H contains rural trip production rates for several different cross-classification schemes and the trip rates associated with each. Finally, Appendix I provides additional information on auto occupancy rates.
NCHRP Report 735 is a supplement to NCHRP Report 716 : Travel Demand Forecasting: Parameters and Techniques , which focused on urban travel.
READ FREE ONLINE
Welcome to OpenBook!
You're looking at OpenBook, NAP.edu's online reading room since 1999. Based on feedback from you, our users, we've made some improvements that make it easier than ever to read thousands of publications on our website.
Do you want to take a quick tour of the OpenBook's features?
Show this book's table of contents , where you can jump to any chapter by name.
...or use these buttons to go back to the previous chapter or skip to the next one.
Jump up to the previous page or down to the next one. Also, you can type in a page number and press Enter to go directly to that page in the book.
To search the entire text of this book, type in your search term here and press Enter .
Share a link to this book page on your preferred social network or via email.
View our suggested citation for this chapter.
Ready to take your reading offline? Click here to buy this book in print or download it as a free PDF, if available.
Get Email Updates
Do you enjoy reading reports from the Academies online for free ? Sign up for email notifications and we'll let you know about new publications in your areas of interest when they're released.
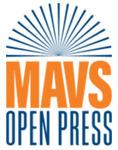
Want to create or adapt books like this? Learn more about how Pressbooks supports open publishing practices.
9 Introduction to Transportation Modeling: Travel Demand Modeling and Data Collection
Chapter overview.
Chapter 9 serves as an introduction to travel demand modeling, a crucial aspect of transportation planning and policy analysis. As explained in previous chapters, the spatial distribution of activities such as employment centers, residential areas, and transportation systems mutually influence each other. The utilization of travel demand forecasting techniques leads to dynamic processes in urban areas. A comprehensive grasp of travel demand modeling is imperative for individuals involved in transportation planning and implementation.
This chapter covers the fundamentals of the traditional four-step travel demand modeling approach. It delves into the necessary procedures for applying the model, including establishing goals and criteria, defining scenarios, developing alternatives, collecting data, and conducting forecasting and evaluation.
Following this chapter, each of the four steps will be discussed in detail in Chapters 10 through 13.
Learning Objectives
- Describe the need for travel demand modeling in urban transportation and relate it to the structure of the four-step model (FSM).
- Summarize each step of FSM and the prerequisites for each in terms of data requirement and model calibration.
- Summarize the available methods for each of the first three steps of FSM and compare their reliability.
- Identify assumptions and limitations of each of the four steps and ways to improve the model.
Introduction
Transportation planning and policy analysis heavily rely on travel demand modeling to assess different policy scenarios and inform decision-making processes. Throughout our discussion, we have primarily explored the connection between urban activities, represented as land uses, and travel demands, represented by improvements and interventions in transportation infrastructure. Figure 9.1 provides a humorous yet insightful depiction of the transportation modeling process. In preceding chapters, we have delved into the relationship between land use and transportation systems, with the houses and factories in the figure symbolizing two crucial inputs into the transportation model: households and jobs. The output of this model comprises transportation plans, encompassing infrastructure enhancements and programs. Chapter 9 delves into a specific model—travel demand modeling. For further insights into transportation planning and programming, readers are encouraged to consult the UTA OERtransport book, “Transportation Planning, Policies, and History.”
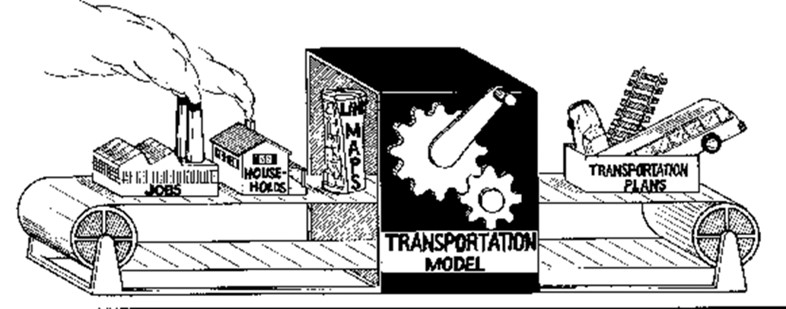
Travel demand models forecast how people will travel by processing thousands of individual travel decisions. These decisions are influenced by various factors, including living arrangements, the characteristics of the individual making the trip, available destination options, and choices regarding route and mode of transportation. Mathematical relationships are used to represent human behavior in these decisions based on existing data.
Through a sequential process, transportation modeling provides forecasts to address questions such as:
- What will the future of the area look like?
- What is the estimated population for the forecasting year?
- How are job opportunities distributed by type and category?
- What are the anticipated travel patterns in the future?
- How many trips will people make? ( Trip Generation )
- Where will these trips end? ( Trip Distribution )
- Which transportation mode will be utilized? ( Mode Split )
- What will be the demand for different corridors, highways, and streets? ( Traffic Assignment )
- Lastly, what impact will this modeled travel demand have on our area? (Rahman, 2008).
9.2 Four-step Model
According to the questions above, Transportation modeling consists of two main stages, regarding the questions outlined above. Firstly, addressing the initial four questions involves demographic and land use analysis, which incorporates the community vision collected through citizen engagement and input. Secondly, the process moves on to the four-step travel demand modeling (FSM), which addresses questions 5 through 8. While FSM is generally accurate for aggregate calculations, it may occasionally falter in providing a reliable test for policy scenarios. The limitations of this model will be explored further in this chapter.
In the first stage, we develop an understanding of the study area from demographic information and urban form (land-use distribution pattern). These are important for all the reasons we discussed in this book. For instance, we must obtain the current age structure of the study area, based on which we can forecast future birth rates, death, and migrations (Beimborn & Kennedy, 1996).
Regarding economic forecasts, we must identify existing and future employment centers since they are the basis of work travel, shopping travel, or other travel purposes. Empirically speaking, employment often grows as the population grows, and the migration rate also depends on a region’s economic growth. A region should be able to generate new employment while sustaining the existing ones based upon past trends and form the basis for judgment for future trends (Mladenovic & Trifunovic, 2014).
After forecasting future population and employment, we must predict where people go (work, shop, school, or other locations). Land-use maps and plans are used in this stage to identify the activity concentrations in the study area. Future urban growth and land use can follow the same trend or change due to several factors, such as the availability of open land for development and local plans and zoning ordinances (Beimborn & Kennedy, 1996). Figure 9.3 shows different possible land-use patterns frequently seen in American cities.
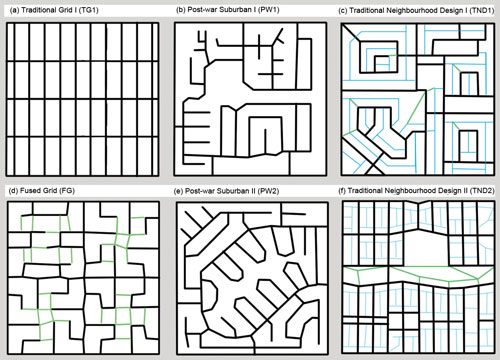
Land-use pattern can also be forecasted through the integration of land use and transportation as we explored in previous chapters.
Figure 9.3 above shows a simple structure of the second stage of FSM.
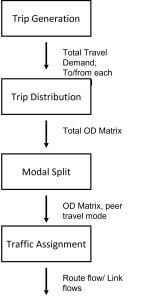
Once the number and types of trips are predicted, they are assigned to various destinations and modes. In the final step, these trips are allocated to the transportation network to compute the total demand for each road segment. During this second stage, additional choices such as the time of travel and whether to travel at all can be modeled using choice models (McNally, 2007). Travel forecasting involves simulating human behavior through mathematical series and calculations, capturing the sequence of decisions individuals make within an urban environment.
The first attempt at this type of analysis in the U.S. occurred during the post-war development period, driven by rapid economic growth. The influential study by Mitchell and Rapkin (1954) emphasized the need to establish a connection between travel and activities, highlighting the necessity for a comprehensive framework. Initial development models for trip generation, distribution, and diversion emerged in the 1950s, leading to the application of the four-step travel demand modeling (FSM) approach in a transportation study in the Chicago area. This model was primarily highway-oriented, aiming to compare new facility development and improved traffic engineering. In the 1960s, federal legislation mandated comprehensive and continuous transportation planning, formalizing the use of FSM. During the 1970s, scholars recognized the need to revise the model to address emerging concerns such as environmental issues and the rise of multimodal transportation systems. Consequently, enhancements were made, leading to the development of disaggregate travel demand forecasting and equilibrium assignment methods that complemented FSM. Today, FSM has been instrumental in forecasting travel demand for over 50 years (McNally, 2007; Weiner, 1997).
Initially outlined by Mannheim (1979), the basic structure of FSM was later expanded by Florian, Gaudry, and Lardinois (1988). Figure 9.3 illustrates various influential components of travel demand modeling. In this representation, “T” represents transportation, encompassing all elements related to the transportation system and its services. “A” denotes the activity system, defined according to land-use patterns and socio-demographic conditions. “P” refers to transportation network performance. “D,” which stands for demand, is generated based on the land-use pattern. According to Florian, Gaudry, and Lardinois (1988), “L” and “S” (location and supply procedures) are optional parts of FSM and are rarely integrated into the model.
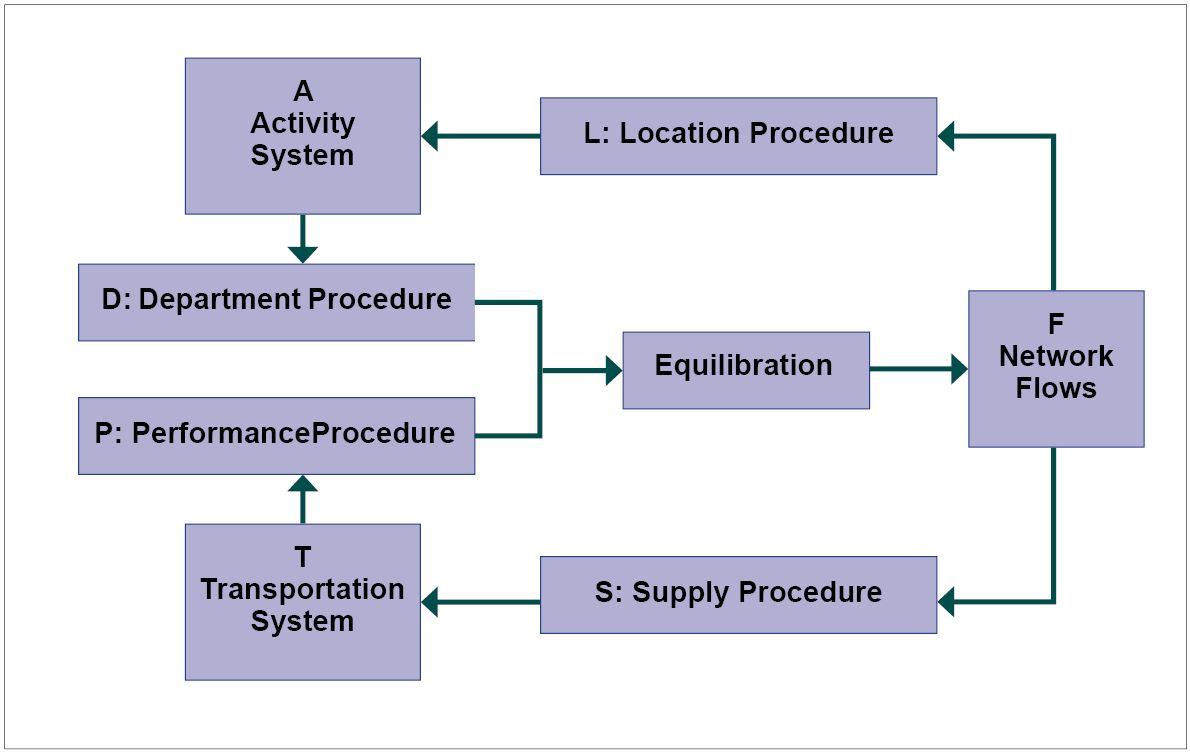
A crucial aspect of the process involves understanding the input units, which are defined both spatially and temporally. Demand generates person trips, which encompass both time and space (e.g., person trips per household or peak-hour person trips per zone). Performance typically yields a level of service, defined as a link volume capacity ratio (e.g., freeway vehicle trips per hour or boardings per hour for a specific transit route segment). Demand is primarily defined at the zonal level, whereas performance is evaluated at the link level.
It is essential to recognize that travel forecasting models like FSM are continuous processes. Model generation takes time, and changes may occur in the study area during the analysis period.
Before proceeding with the four steps of FSM, defining the study area is crucial. Like most models discussed, FSM uses traffic analysis zones (TAZs) as the geographic unit of analysis. However, a higher number of TAZs generally yield more accurate results. The number of TAZs in the model can vary based on its purpose, data availability, and vintage. These zones are characterized or categorized by factors such as population and employment. For modeling simplicity, FSM assumes that trip-making begins at the center of a zone (zone centroid) and excludes very short trips that start and end within a TAZ, such as those made by bike or on foot.
Furthermore, highway systems and transit systems are considered as networks in the model. Highway or transit line segments are coded as links, while intersections are represented as nodes. Data regarding network conditions, including travel times, speeds, capacity, and directions, are utilized in the travel simulation process. Trips originate from trip generation zones, traverse a network of links and nodes, and conclude at trip attraction zones.
Trip Generation
Trip generation is the first step in the FSM model. This step defines the magnitude of daily travel in the study area for different trip purposes. It will also provide us with an estimate of the total trips to and from each zone, creating a trip production and attraction matrix for each trip’s purpose. Trip purposes are typically categorized as follows:
- Home-based work trips (work trips that begin or end at home),
- Home-based shopping trips,
- Home-based other trips,
- School trips,
- Non-home-based trips (trips that neitherbeginnorendathome),
- Trucktrips,and
- Taxitrips(Ahmed,2012).
Trip attractions are based on the level of employment in a zone. In the trip generation step, the assumptions and limitations are listed below:
- Independent decisions: Travel behavior is affected by many factors generated within a household; the model ignores most of these factors. For example, childcare may force people to change their travel plans.
- Limited trip purposes: This model consists of a limited number of trip purposes for simplicity, giving rise to some model limitations. Take shopping trips, for example; they are all considered in the same weather conditions. Similarly, we generate home-based trips for various purposes (banking, visiting friends, medical reasons, or other purposes), all of which are affected by factors ignored by the model.
- Trip combinations: Travelers are often willing to combine various trips into a chain of short trips. While this behavior creates a complex process, the FSM model treats this complexity in a limited way.
- Feedback, cause, and effect problems: Trip generation often uses factors that are a function of the number of trips. For instance, for shopping trip attractions in the FSM model, we assume they are a retail employment function. However, it is logical to assume how many customers these retail centers attract. Alternatively, we can assume that the number of trips a household makes is affected by the number of private cars they own. Nevertheless, the activity levels of families determine the total number of cars.
As mentioned, trip generation process estimations are done separately for each trip purpose. Equations 1 and 2 show the function of trip generation and attraction:

where Oi and Dj trip are generated and attracted respectively, x refers to socio-economic characteristics, and y refers to land-use properties.
Generally, FSM aggregates different trip purposes previously listed into three categories: home-based work trips (HBW) , home-based other (or non-work) trips (HBO) , and non-home-based trips (NHB) . Trip ends are either the origin (generation) or destination (attraction), and home-end trips comprise most trips in a study area. We can also model trips at different levels, such as zones, households, or person levels (activity-based models). Household-level models are the most common scale for trip productions, and zonal-level models are appropriate for trip attractions (McNally, 2007).
There are three main methods for a trip generation or attraction.
- The first method is multiple regression based on population, jobs, and income variables.
- The second method in this step is experience-based analysis, which can show us the ratio of trips generated frequently.
- The third method is cross-classification . Cross-classification is like the experience-based analysis in that it uses trip rates but in an extended format for different categories of trips (home-based trips or non-home-based trips) and different attributes of households, such as car ownership or income.
Elaborating on the differences between these methods, category analysis models are more common for the trip generation model, while regression models demonstrate better performance for trip attractions (Meyer, 2016). Production models are recognized to be influenced by a range of explanatory and policy-sensitive variables (e.g., car ownership, household income, household size, and the number of workers). However, estimation is more problematic for attraction models because regional travel surveys are at the household level (thus providing more accurate data for production models) and not for nonresidential land uses (which is important for trip attraction). Additionally, estimation can be problematic because explanatory trip attraction variables may usually underperform (McNally, 2007). For these reasons, survey data factoring is required prior to relating sample trips to population-level attraction variables, typically achieved via regression analysis. Table 9.1 shows the advantages and disadvantages of each of these two models.
Trip Distribution
Thus far, the number of trips beginning or ending in a particular zone have been calculated. The second step explores how trips are distributed between zones and how many trips are exchanged between two zones. Imagine a shopping trip. There are multiple options for accessible shopping malls accessible. However, in the end, only one will be selected for the destination. This information is modeled in the second step as a distribution of trips. The second step results are usually a very large Origin-Destination (O-D) matrix for each trip purpose. The O-D matrix can look like the table below (9.2), in which sum of Tij by j shows us the total number of trips attracted in zone J and the sum of Tij by I yield the total number of trips produced in zone I.
Up to this point, we have calculated the number of trips originating from or terminating in a specific zone. The next step involves examining how these trips are distributed across different zones and how many trips are exchanged between pairs of zones. To illustrate, consider a shopping trip: there are various options for reaching shopping malls, but ultimately, only one option is chosen as the destination. This process is modeled in the second step as the distribution of trips. The outcome of this step typically yields a large Origin-Destination (O-D) matrix for each trip purpose. An O-D matrix might resemble the table below (9.2), where the sum of Tij by j indicates the total number of trips attracted to zone J, and the sum of Tij by I represents the total number of trips originating from zone I.
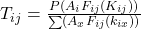
T ij = trips produced at I and attracted at j
P i = total trip production at I
A j = total trip attraction at j
F ij = a calibration term for interchange ij , (friction factor) or travel
time factor ( F ij =C/t ij n )
C= calibration factor for the friction factor
K ij = a socioeconomic adjustment factor for interchange ij
i = origin zone
n = number of zones
Different methods (units) in the gravity model can be used to perform distance measurements. For instance, distance can be represented by time, network distance, or travel costs. For travel costs, auto travel cost is the most common and straightforward way of monetizing distance. A combination of different costs, such as travel time, toll payments, parking payments, etc., can also be used. Alternatively, a composite cost of both car and transit costs can be used (McNally, 2007).
Generalized travel costs can be a function of time divided into different segments. For instance, public transit time can be divided into the following segments: in-vehicle time, walking time, waiting time, interchange time, fare, etc. Since travelers perceive time value differently for each segment (like in-vehicle time vs. waiting time), weights are assigned based on the perceived value of time (VOT). Similarly, car travel costs can be categorized into in-vehicle travel time or distance, parking charge, tolls, etc.
As with the first step in the FSM model, the second step has assumptions and limitations that are briefly explained below.
- Constant trip times: In order to utilize the model for prediction, it assumes that the duration of trips remains constant. This means that travel distances are measured by travel time, and the assumption is that enhancements in the transportation system, which reduce travel times, are counterbalanced by the separation of origins and destinations.
- Automobile travel times to represent distance: We utilize travel time as a proxy for travel distance. In the gravity model, this primarily relies on private car travel time and excludes travel times via other modes like public transit. This leads to a broader distribution of trips.
- Limited consideration of socio-economic and cultural factors: Another drawback of the gravity model is its neglect of certain socio-economic or cultural factors. Essentially, this model relies on trip production and attraction rates along with travel times between them for predictions. Consequently, it may overestimate trip rates between high-income groups and nearby low-income Traffic Analysis Zones (TAZs). Therefore, incorporating more socio-economic factors into the model would enhance accuracy.
- Feedback issues: The gravity model’s reliance on travel times is heavily influenced by congestion levels on roads. However, measuring congestion proves challenging, as discussed in subsequent sections. Typically, travel times are initially assumed and later verified. If the assumed values deviate from actual values, they require adjustment, and the calculations need to be rerun.
Mode choice
FSM model’s third step is a mode-choice estimation that helps identify what types of transportation travelers use for different trip purposes to offer information about users’ travel behavior. This usually results in generating the share of each transportation mode (in percentages) from the total number of trips in a study area using the utility function (Ahmed, 2012). Performing mode-choice estimations is crucial as it determines the relative attractiveness and usage of various transportation modes, such as public transit, carpooling, or private cars. Modal split analysis helps evaluate improvement programs or proposals (e.g., congestion pricing or parking charges) aimed at enhancing accessibility or service levels. It is essential to identify the factors contributing to the utility and disutility of different modes for different travel demands (Beimborn & Kennedy, 1996). Comparing the disutility of different modes between two points aids in determining mode share. Disutility typically refers to the burdens of making a trip, such as time, costs (fuel, parking, tolls, etc.). Once disutility is modeled for different trip purposes between two points, trips can be assigned to various modes based on their utility. As discussed in Chapter 12, a mode’s advantage in terms of utility over another can result in a higher share of trips using that mode.
The assumptions and limitations for this step are outlined as follows:
- Choices are only affected by travel time and cost: This model assumes that changes in mode choices occur solely if transportation cost or travel time in the transportation network or transit system is altered. For instance, a more convenient transit mode with the same travel time and cost does not affect the model’s results.
- Omitted factors: Certain factors like crime, safety, and security, which are not included in the model, are assumed to have no effect, despite being considered in the calibration process. However, modes with different attributes regarding these omitted factors yield no difference in the results.
- Simplified access times: The model typically overlooks factors related to the quality of access, such as neighborhood safety, walkability, and weather conditions. Consequently, considerations like walkability and the impact of a bike-sharing program on the attractiveness of different modes are not factored into the model.
- Constant weights: The model assumes that the significance of travel time and cost remains constant for all trip purposes. However, given the diverse nature of trip purposes, travelers may prioritize travel time and cost differently depending on the purpose of their trip.
The most common framework for mode choice models is the nested logit model, which can accommodate various explanatory variables. However, before the final step, results need to be aggregated for each zone (Koppelman & Bhat, 2006).
A generalized modal split chart is depicted in Figure 9.5.
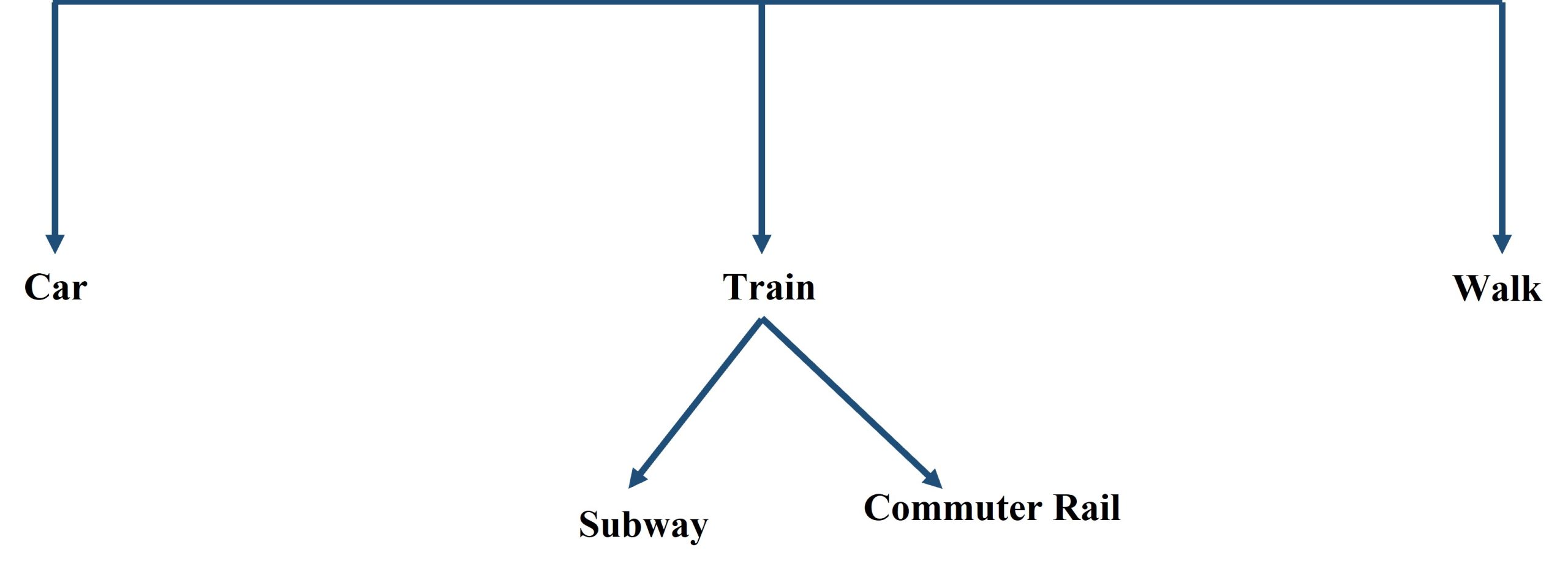
In our analysis, we can use binary logit models (dummy variable for dependent variable) if we have two modes of transportation (like private cars and public transit only). A binary logit model in the FSM model shows us if changes in travel costs would occur, such as what portion of trips changes by a specific mode of transport. The mathematical form of this model is:

where: P_ij 1= The proportion of trips between i and j by mode 1 . Tij 1= Trips between i and j by mode 1.
Cij 1= Generalized cost of travel between i and j by mode 1 .
Cij^2= Generalized cost of travel between i and j by mode 2 .
b= Dispersion Parameter measuring sensitivity to cost.
It is also possible to have a hierarchy of transportation modes for using a binary logit model. For instance, we can first conduct the analysis for the private car and public transit and then use the result of public transit to conduct a binary analysis between rail and bus.
Trip assignment
After breaking down trip counts by mode of transportation, we analyze the routes commuters take from their starting point to their destination, especially for private car trips. This process is known as trip assignment and is the most intricate stage within the FSM model. Initially, the minimum path assigns trips for each origin-destination pair based on either travel costs or time. Subsequently, the assigned volume of trips is compared to the capacity of the route to determine if congestion would occur. If congestion does happen (meaning that traffic volume exceeds capacity), the speed of the route needs to be decreased, resulting in increased travel costs or time. When the Volume/Capacity ratio (v/c ratio) changes due to congestion, it can lead to alterations in both speed and the shortest path. This characteristic of the model necessitates an iterative process until equilibrium is achieved.
The process for public transit is similar, but with one distinction: instead of adjusting travel times, headways are adjusted. Headway refers to the time between successive arrivals of a vehicle at a stop. The duration of headways directly impacts the capacity and volume for each transit vehicle. Understanding the concept of equilibrium in the trip assignment step is crucial because it guides the iterative process of the model. The conclusion of this process is marked by equilibrium, a concept known as Wardrop equilibrium. In Wardrop equilibrium, traffic naturally organizes itself in congested networks so that individual commuters do not switch routes to reduce travel time or costs. Additionally, another crucial factor in this step is the time of day.
Like previous steps, the following assumptions and limitations are pertinent to the trip assignment step:
1. Delays on links: Most traffic assignment models assume that delays occur on the links, not the intersections. For highways with extensive intersections, this can be problematic because intersections involve highly complex movements. Intersections are excessively simplified if the assignment process does not modify control systems to reach an equilibrium.
2. Points and links are only for trips: This model assumes that all trips begin and finish at a single point in a zone (centroids), and commuters only use the links considered in the model network. However, these points and links can vary in the real world, and other arterials or streets might be used for commutes.
3. Roadway capacities: In this model, a simple assumption helps determine roadways’ capacity. Capacity is found based on the number of lanes a roadway provides and the type of road (highway or arterial).
4. Time of the day variations: Traffic volume varies greatly throughout the day and week. In this model, a typical workday of the week is considered and converted to peak hour conditions. A factor used for this step is called the hour adjustment factor. This value is critical because a small number can result in a massive difference in the congestion level forecasted on the model.
5. Emphasis on peak hour travel: The model forecasts for the peak hour but does not forecast for the rest of the day. The models make forecasts for a typical weekday but neglect specific conditions of that time of the year. After completing the fourth step, precise approximations of travel demand or traffic count on each road are achieved. Further models can be used to simulate transportation’s negative or positive externalities. These externalities include air pollution, updated travel times, delays, congestion, car accidents, toll revenues, etc. These need independent models such as emission rate models (Beimborn & Kennedy, 1996).
The basic equilibrium condition point calculation is an algorithm that involves the computation of minimum paths using an all-or-nothing (AON) assignment model to these paths. However, to reach equilibrium, multiple iterations are needed. In AON, it is assumed that the network is empty, and a free flow is possible. The first iteration of the AON assignment requires loading the traffic by finding the shortest path. Due to congestion and delayed travel times, the
previous shortest paths may no longer be the best minimum path for a pair of O-D. If we observe a notable decrease in travel time or cost in subsequent iterations, then it means the equilibrium point has not been reached, and we must continue the estimation. Typically, the following factors affect private car travel times: distance, free flow speed on links, link capacity, link speed capacity, and speed flow relationship .
The relationship between the traffic flow and travel time equation used in the fourth step is:

t= link travel time per length unit
t 0 =free-flow travel time
v=link flow
c=link capacity
a, b, and n are model (calibrated) parameters
Model improvement
Improvements to FSM continue to generate more accurate results. Since transportation dynamics in urban and regional areas are under the complex influence of various factors, the existing models may not be able to incorporate all of them. These can be employer-based trip reduction programs, walking and biking improvement schemes, a shift in departure (time of the day), or more detailed information on socio-demographic and land-use-related factors. However, incorporating some of these variables is difficult and can require minor or even significant modifications to the model and/or computational capacities or software improvements. The following section identifies some areas believed to improve the FSM model performance and accuracy.
• Better data: An effective way of improving the model accuracy is to gather a complete dataset that represents the general characteristics of the population and travel pattern. If the data is out- of-date or incomplete, we will get poor results.
• Better modal split: As you saw in previous sections, the only modes incorporated into the model are private car and public transit trips, while in some cities, a considerable fraction of trips are made by bicycle or by walking. We can improve our models by producing methods to consider these trips in the first and third steps.
• Auto occupancy: In contemporary transportation planning practices, especially in the US, some new policies are emerging for carpooling. We can calculate auto occupancy rates using different mode types, such as carpooling, sensitive to private car trips’ disutility, parking costs, or introducing a new HOV lane.
• Time of the day: In this chapter, the FSM framework discussed is oriented toward peak hour (single time of the day) travel patterns. Nonetheless, understanding the nature of congestion in other hours of the day is also helpful for understanding how travelers choose their travel time.
• A broader trip purpose: Additional trip purposes may provide a better understanding of the
factors affecting different trip purposes and trip-chaining behaviors. We can improve accuracy by having more trip purposes (more disaggregate input and output for the model).
- The concept of access: As discussed, land-use policies that encourage public transit use or create amenities for more convenient walking are not present in the model. Developing factors or indices that reflect such improvements in areas with high demand for non-private vehicles and incorporating them in choice models can be a good improvement.
- Land use feedback: To better understand interactions between land use and travel demand, a land-use simulation model can be added to these steps to determine how a proposed transportation change will lead to a change in land use.
- Intersection delays: As mentioned in the fourth step, intersections in major highways create significant delays. Incorporating models that calculate delays at these intersections, such as stop signs, could be another improvement to the model.
A Simple Example of the FSM model
An example of FSM is provided in this section to illustrate a typical application of this model in the U.S. In the first phase, the specifications about the transportation network and household data are needed. In this hypothetical example, 5 percent of households in each TAZ were sampled and surveyed, which generated 1,955 trips in 200 households. As a hypothetical case study, this sample falls below the standard required for statistical significance but is relevant to demonstrate FSM.
A home interview survey was carried out to gather data from a five percent sample of households in each TAZ. This survey resulted in 1,852 trips from 200 households. It is important to note that the sample size in this example falls below the minimum required for statistical significance, as it is intended for learning purposes only.
Table 9.3 provides network information such as speed limits, number of lanes, and capacity. Table 9.4 displays the total number of households and jobs in three industry sectors for each zone. Additionally, Table 9.5 breaks down the household data into three car ownership groups, which is one of the most significant factors influencing trip making.
In the first step (trip generation), a category model (i.e., cross-classification) helped estimate trips. The sampled population’s sociodemographic and trip data for different purposes helped calculate this estimate. Since research has shown the significant effect of auto ownership on private car trip- making (Ben-Akiva & Lerman, 1974), disaggregating the population based on the number of private cars generates accurate results. Table 9.7 shows the trip-making rate for different income and auto ownership groups.
Also, as mentioned in previous sections, multiple regression estimation analysis can be used to generate the results for the attraction model. Table 9.7 shows the equations for each of the trip purposes.
After estimating production and attraction, the models are used for population data to generate results for the first step. Next, comparing the results of trip production and attraction, we can observe that the total number of trips for each purpose is different. This can be due to using different methods for production and attraction. Since the production method is more reliable, attraction is typically normalized by production. Also, some external zones in our study area are either attracting trips from our zones or generating them. In this case, another alternative is to extend the boundary of the study area and include more zones.
As mentioned, the total number of trips produced and attracted are different in these results. To address this mismatch, we can use a balance factor to come up with the same trip generation and attraction numbers if we want to keep the number of zones within our study area. Alternatively, we can consider some external stations in addition to designated zones. In this example, using the latter seems more rational because, as we saw in Table 9.4, there are more jobs than the number of households aggregately, and our zone may attract trips from external locations.
For the trip distribution step, we use the gravity model. For internal trips, the gravity model is:

and f(tij) is some function of network level of service (LOS)
To apply the gravity model, we need to calculate the impedance function first, which is represented here by travel cost. Table 9.9 shows the minimum travel path between each pair of zones ( skim tree ) in a matrix format in which each cell represents travel time required to travel between the corresponding row and column of that cell.
Table 9.9-Travel cost table (skim tree)
Note. Table adapted from “The Four-Step Model” by M. McNally, In D. A. Hensher, & K. J. Button (Eds.), Handbook of transport modelling , Volume1, p. 5, Bingley, UK: Emerald Publishing. Copyright 2007 by Emerald Publishing.
With having minimum travel costs between each pair of zones, we can calculate the impedance function for each trip purpose using the formula

Table 9.10 shows the model parameters for calculating the impedance function for different trip purposes:
After calculating the impedance function , we can calculate the result of the trip distribution. This stage generates trip matrices since we calculate trips between each zone pair. These matrices are usually in “Origin-Destination” (OD) format and can be disaggregated by the time of day. Field surveys help us develop a base-year trip distribution for different periods and trip purposes. Later, these empirical results will help forecast trip distribution. When processing the surveys, the proportion of trips from the production zone to the attraction zone (P-A) is also generated. This example can be seen in Table 9.11. Looking at a specific example, the first row in table is for the 2-hour morning peak commute time period. The table documents that the production to attraction factor for the home-based work trip is 0.3. Unsurprisingly, the opposite direction, attraction to production zone is 0.0 for this time of day. Additionally, the table shows that the factor for HBO and NHB trips are low but do occur during this time period. This could represent shopping trips or trips to school. Table 9.11 table also contains the information for average occupancy levels of vehicles from surveys. This information can be used to convert person trips to vehicle trips or vice versa.
Table 9.11 Trip distribution rates for different time of the day and trip purposes
The O-D trip table is calculated by adding the multiplication of the P-to-A factor by corresponding cell of the P-A trip table and adding the corresponding cell of the transposed P-A trip table multiplied by the A-to-P factor. These results, which are the final output of second step, are shown in Table 9.12.
Once the Production-Attraction (P-A) table is transformed into Origin-Destination (O-D) format and the complete O-D matrix is computed, the outcomes will be aggregated for mode choice and traffic assignment modeling. Further elaboration on these two steps will be provided in Chapters 11 and 12.
In this chapter, we provided a comprehensive yet concise overview of four-step travel demand modeling including the process, the interrelationships and input data, modeling part and extraction of outputs. The complex nature of cities and regions in terms of travel behavior, the connection to the built environment and constantly growing nature of urban landscape, necessitate building models that are able to forecast travel patterns for better anticipate and prepare for future conditions from multiple perspectives such as environmental preservation, equitable distribution of benefits, safety, or efficiency planning. As we explored in this book, nearly all the land-use/transportation models embed a transportation demand module or sub model for translating magnitude of activities and interconnections into travel demand such as VMT, ridership, congestion, toll usage, etc. Four-step models can be categorized as gravity-based, equilibrium-based models from the traditional approaches. To improve these models, several new extensions has been developed such as simultaneous mode and destination choice, multimodality (more options for mode choice with utility), or microsimulation models that improve granularity of models by representing individuals or agents rather than zones or neighborhoods.
Travel demand modeling are models that predicts the flow of traffic or travel demand between zones in a city using a sequence of steps.
- Intermodality refers to the concept of utilizing two or more travel modes for a trip such as biking to a transit station and riding the light rail.
- Multimodality is a type of transportation network in which a variety of modes such as public transit, rail, biking networks, etc. are offered.
Zoning ordinances is legal categorization of land use policies that permits or prohibits certain built environment factors such as density.
Volume capacity ratio is ratio that divides the demand on a link by the capacity to determine the level of service.
- Zone centroid is usually the geometric center of a zone in modeling process where all trips originate and end.
Home-based work trips (HBW) are the trips that originates from home location to work location usually in the AM peak.
- Home-based other (or non-work) trips (HBO) are the trips that originates from home to destinations other than work like shopping or leisure.
Non-home-based trips (NHB) are the trips that neither origin nor the destination are home or they are part of a linked trip.
Cross-classification is a method for trip production estimation that disaggregates trip rates in an extended format for different categories of trips like home-based trips or non-home-based trips and different attributes of households such as car ownership or income.
- Generalized travel costs is a function of time divided into sections such as in vehicle time vs. waiting time or transfer time in a transit trip.
Binary logit models is a type of logit model where the dependent variable can take only a value of 0 or 1.
- Wardrop equilibrium is a state in traffic assignment model where are drivers are reluctant to change their path because the average travel time is at a minimum.
All-or-nothing (AON) assignment model is a model that assumes all trips between two zones uses the shortest path regardless of volume.
Speed flow relationship is a function that determines the speed based on the volume (flow)
skim tree is structure of travel time by defining minimum cost path for each section of a trip.
Key Takeaways
In this chapter, we covered:
- What travel demand modeling is for and what the common methods are to do that.
- How FSM is structured sequentially, what the relationships between different steps are, and what the outputs are.
- What the advantages and disadvantages of different methods and assumptions in each step are.
- What certain data collection and preparation for trip generation and distribution are needed through a hypothetical example.
Prep/quiz/assessments
- What is the need for regular travel demand forecasting, and what are its two major components?
- Describe what data we require for each of the four steps.
- What are the advantages and disadvantages of regression and cross-classification methods for a trip generation?
- What is the most common modeling framework for mode choice, and what result will it provide us?
- What are the main limitations of FSM, and how can they be addressed? Describe the need for travel demand modeling in urban transportation and relate it to the structure of the four-step model (FSM).
Ahmed, B. (2012). The traditional four steps transportation modeling using a simplified transport network: A case study of Dhaka City, Bangladesh. International Journal of Advanced Scientific Engineering and Technological Research , 1 (1), 19–40. https://discovery.ucl.ac.uk/id/eprint/1418961/
ALMEC, C . (2015). The Project for capacity development on transportation planning and database management in the republic of the Philippines: MMUTIS update and enhancement project (MUCEP) : Project Completion Report . Japan International Cooperation Agency. (JICA) Department of Transportation and Communications (DOTC) . https://books.google.com/books?id=VajqswEACAAJ .
Beimborn, E., and Kennedy, R. (1996). Inside the black box: Making transportation models work for livable communities . Washington, DC: Citizens for a Better Environment and the Environmental Defense Fund. https://www.piercecountywa.gov/DocumentCenter/View/755/A-GuideToModeling?bidId
Ben-Akiva, M., & Lerman, S. R. (1974). Some estimation results of a simultaneous model of auto ownership and mode choice to work. Transportation , 3 (4), 357–376. https://doi.org/10.1007/bf00167966
Ewing, R., & Cervero, R. (2010). Travel and the built environment: A meta-analysis. Journal of the American Planning Association , 76 (3), 265–294. https://doi.org/10.1080/01944361003766766
Florian, M., Gaudry, M., & Lardinois, C. (1988). A two-dimensional framework for the understanding of transportation planning models. Transportation Research Part B: Methodological , 22 (6), 411–419. https://doi.org/10.1016/0191-2615(88)90022-7
Hadi, M., Ozen, H., & Shabanian, S. (2012). Use of dynamic traffic assignment in FSUTMS in support of transportation planning in Florida. Florida International University Lehman Center for Transportation Research. https://rosap.ntl.bts.gov/view/dot/24925
Hansen, W. (1959). How accessibility shapes land use.” Journal of the American Institute of Planners 25 (2): 73–76. https://doi.org/10.1080/01944365908978307
Gavu, E. K. (2010). Network based indicators for prioritising the location of a new urban transport connection: Case study Istanbul, Turkey (Master’s thesis, University of Twente). International Institute for Geo-Information Science and Earth Observation Enschede. http://essay.utwente.nl/90752/1/Emmanuel%20Kofi%20Gavu-22239.pdf
Karner, A., London, J., Rowangould, D., & Manaugh, K. (2020). From transportation equity to transportation justice: Within, through, and beyond the state. Journal of Planning Literature , 35 (4), 440–459. https://doi.org/10.1177/0885412220927691
Kneebone, E., & Berube, A. (2013). Confronting suburban poverty in America . Brookings Institution Press.
Koppelman, Frank S, and Chandra Bhat. (2006). A self instructing course in mode choice modeling: multinomial and nested logit models. U.S. Department of Transportation Federal Transit Administration https://www.caee.utexas.edu/prof/bhat/COURSES/LM_Draft_060131Final-060630.pdf
Manheim, M. L. (1979). Fundamentals of transportation systems analysis. Volume 1: Basic Concepts . The MIT Press https://mitpress.mit.edu/9780262632898/fundamentals-of-transportation-systems-analysis/
McNally, M. G. (2007). The four step model. In D. A. Hensher, & K. J. Button (Eds.), Handbook of transport modelling , Volume1 (pp.35–53). Bingley, UK: Emerald Publishing.
Meyer, M. D., & Institute Of Transportation Engineers. (2016). Transportation planning handbook . Wiley.
Mladenovic, M., & Trifunovic, A. (2014). The shortcomings of the conventional four step travel demand forecasting process. Journal of Road and Traffic Engineering , 60 (1), 5–12.
Mitchell, R. B., and C. Rapkin. (1954). Urban traffic: A function of land use . Columbia University Press. https://doi.org/10.7312/mitc94522
Rahman, M. S. (2008). “ Understanding the linkages of travel behavior with socioeconomic characteristics and spatial Environments in Dhaka City and urban transport policy applications .” Hiroshima: (Master’s thesis, Hiroshima University.) Graduate School for International Development and Cooperation. http://sr-milan.tripod.com/Master_Thesis.pdf
Rodrigue, J., Comtois, C., & Slack, B. (2020). The geography of transport systems . London ; New York Routledge.
Shen, Q. (1998). Location characteristics of inner-city neighborhoods and employment accessibility of low-wage workers. Environment and Planning B: Planning and Design , 25 (3), 345–365.
Sharifiasl, S., Kharel, S., & Pan, Q. (2023). Incorporating job competition and matching to an indicator-based transportation equity analysis for auto and transit in Dallas-Fort Worth Area. Transportation Research Record , 03611981231167424. https://doi.org/10.1177/03611981231167424
Weiner, Edward. 1997. Urban transportation planning in the United States: An historical overview . US Department of Transportation. https://rosap.ntl.bts.gov/view/dot/13691
Xiongbing, J, Grammenos, F. (2013, May, 21) . Taking the Guesswork out of Designing for Walkability. Planetizen . https://www.planetizen.com/node/63248
Home-based other (or non-work) trips (HBO) are the trips that originates from home to destinations other than work like shopping or leisure.
gravity model is a type of accessibility measurement in which the employment in destination and population in the origin defines thee degree of accessibility between the two zones.
Impedance function is a function that convert travel costs (usually time or distance) to the level of difficulty of getting from one location to the other.
Transportation Land-Use Modeling & Policy Copyright © by Soheil Sharifi and Qisheng Pan is licensed under a Creative Commons Attribution-NonCommercial-ShareAlike 4.0 International License , except where otherwise noted.
Share This Book
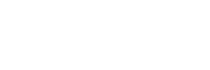
INRIX Trip Analytics
Ready-to-Query Trip Data that Accelerates Insights and Decisions
Make better decisions by understanding the movement of people through accurate trip analysis
Trip Analytics powered by the CATT Lab provides aggregated insights into the trips and routes people take.
Traditionally, manual survey methods have proven costly, labor-intensive and prone to inaccuracies. INRIX eliminates all that. Trip Analytics is derived from industry-leading geospatial data processing, which enables a new understanding of population movement information such as origin and destination zones, diversion routes during peak time and incidents, corridor usage and more.
The use cases are almost limitless and include helping cities better serve and route commercial freight, allowing agencies to track trends for better decision making, helping retailers select new retail sites, and helping financial services professionals understand economic signals and inflection points to inform asset allocation decisions.
CLICK HERE TO GET STARTED
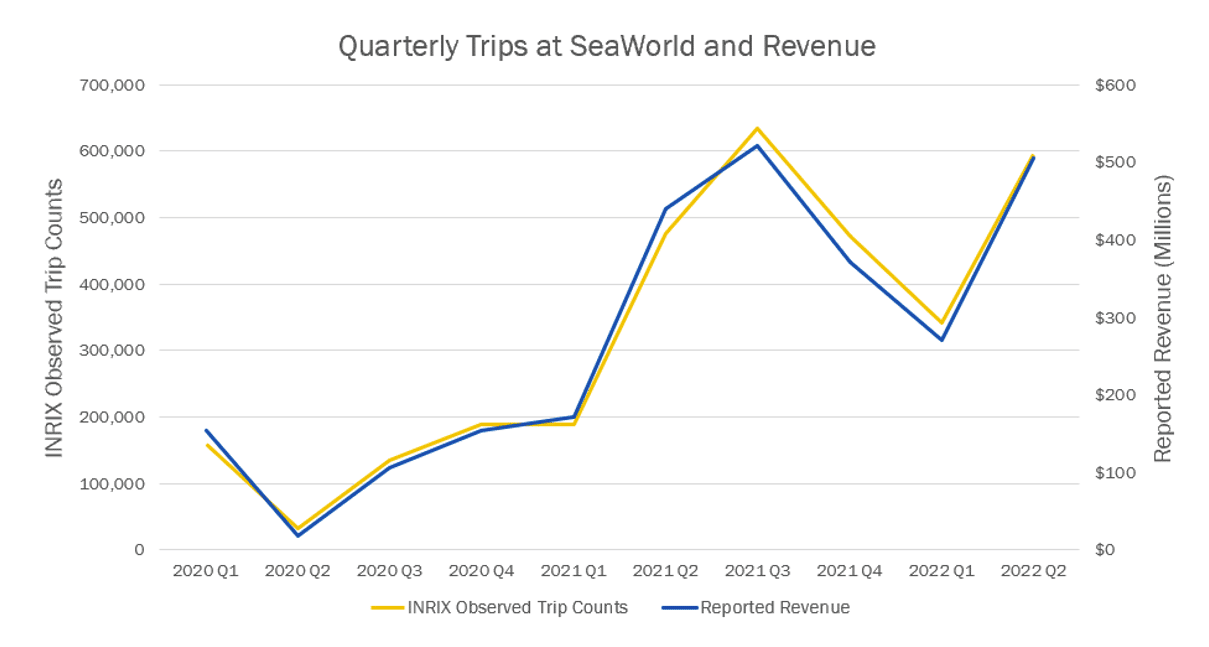
Optimize Your Investment Strategies
Alternative Data from Trip Analytics helps portfolio managers, data analysts, and other financial services professionals understand location-based histories, trends, economic signals, and inflection points to inform asset allocation decisions.
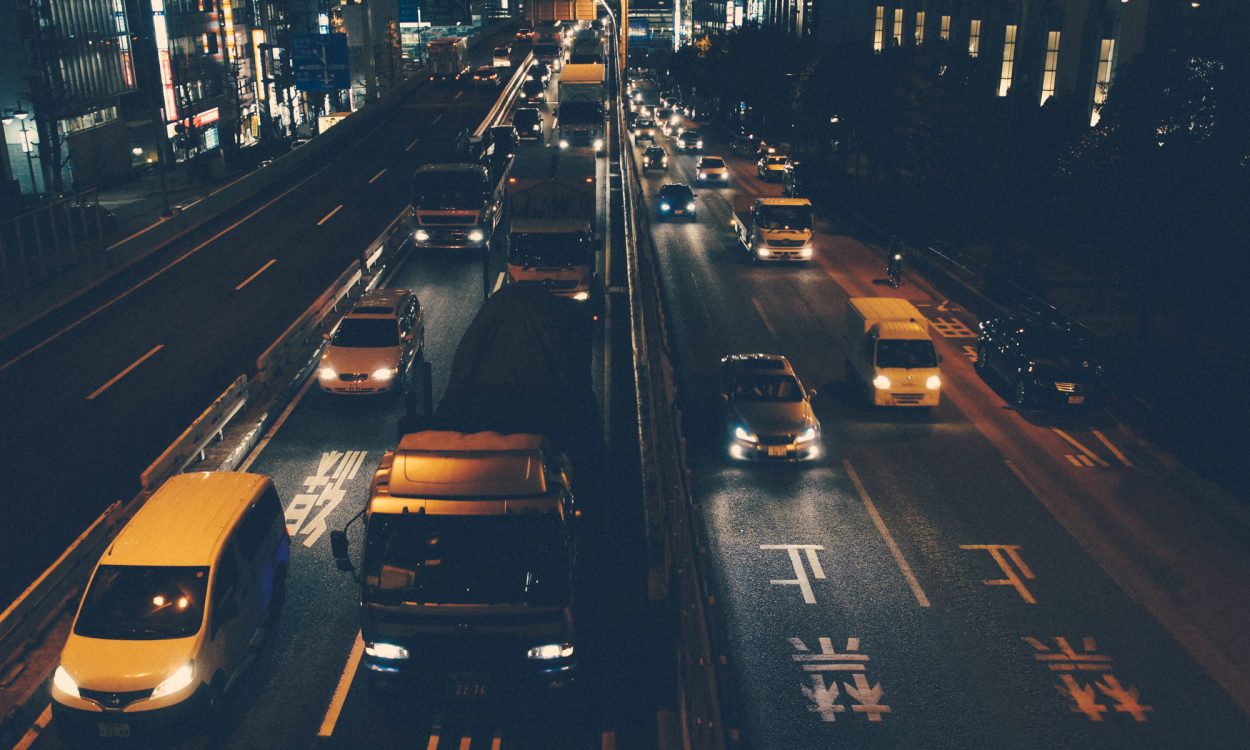
Manage transportation with greater precision
INRIX Trip Analytics provides transportation and planning agencies with accurate and affordable trip data, allowing them to track vehicle traffic data trends and accurately calibrate models to make more informed decisions for their transportation network investments.
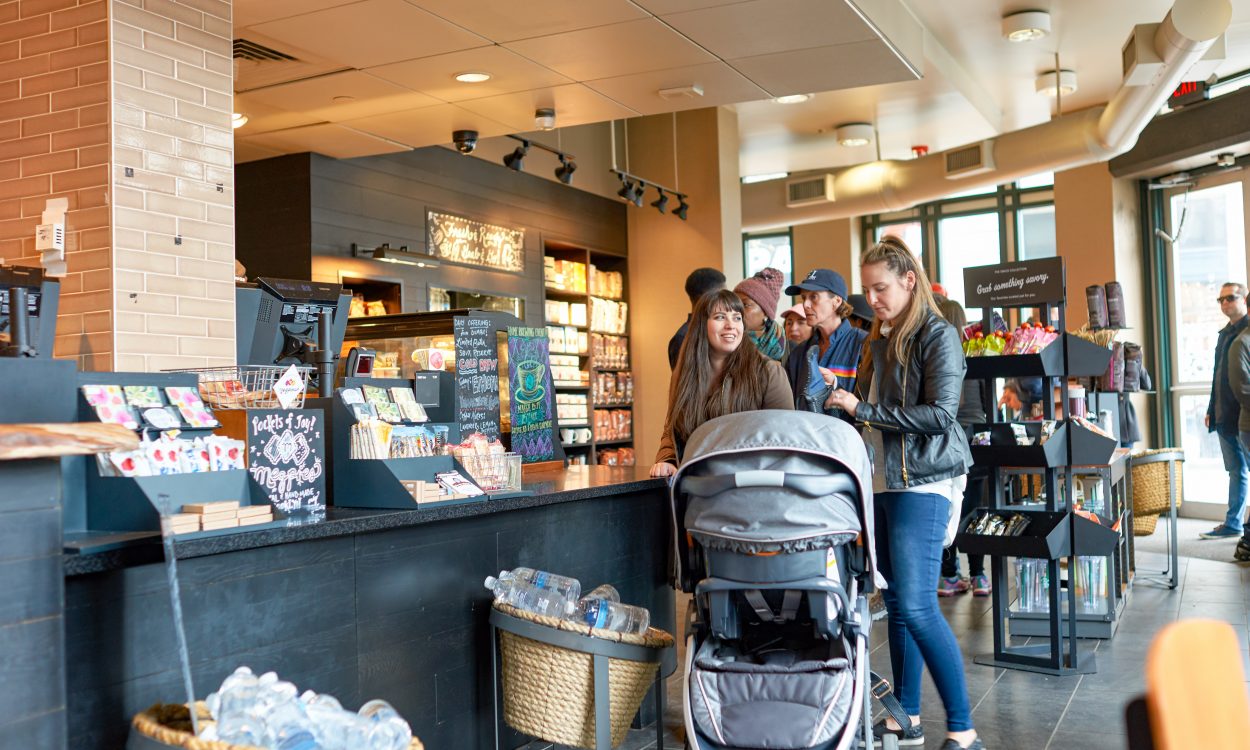
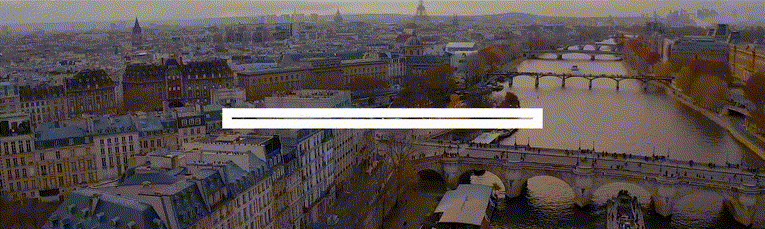
More efficiently select new retail sites
INRIX Trip Analytics provides retailers with comprehensive, competitive location and population analysis to help them select new retail sites without having to physically visit each potential location.
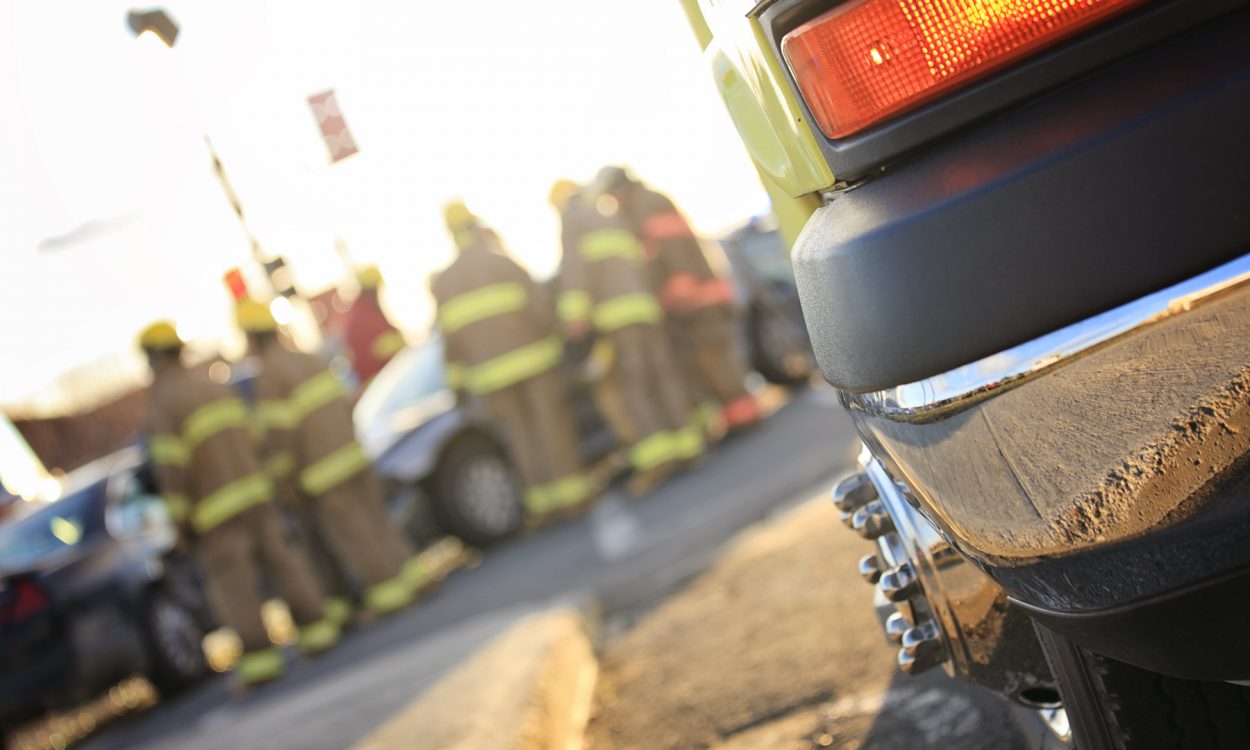
Respond more quickly to emergencies
INRIX Trip Analytics gives emergency management accurate trip data analyses on major roads in and out of an urban area, so they can effectively evacuate people in the event of a natural disaster or other major events.
Trip Reports
Aggregated lists of anonymized trips with route details and waypoints.
Trip Matrices
Compiled according to customer Traffic Analysis Zones
Flexible Analysis Tools
Define your study area via shapefile or user defined zones or customize your analysis by mode, time, or origin/destination
Offline Data Download
Export raw trip records or matrices for offline analysis.
Aggregated list of anonymized trips with defined paths
Connect & Learn More
Defining and understanding trip chaining behaviour
- Published: 19 July 2007
- Volume 35 , pages 55–72, ( 2008 )
Cite this article
- Frank Primerano 1 ,
- Michael A. P. Taylor 2 ,
- Ladda Pitaksringkarn 2 &
- Peter Tisato 1
4711 Accesses
138 Citations
Explore all metrics
Trip chaining is a phenomenon that we know exists but rarely investigate. This could be attributed to either the difficulty in defining trip chains, extracting such information from travel diary surveys, the difficulty in analysing all the possible trip chain types, or all of the above. Household travel diary surveys provide a wealth of information on the travel patterns of individuals and households. Since such surveys collect all information related to travel undertaken, in theory it should be possible to extract trip-chaining characteristics of travel from them. Due to the difficulty in establishing and analysing all of the possible trip chain types, the majority of research on trip chaining has appeared to focus on work travel only. However, work related travel in many cities does not represent the majority of activities undertaken and, for some age groups, does not represent any travel at all. This paper begins by reviewing existing research in the field of trip chaining. In particular, investigations into the definitions of trip chaining, the defined typologies of trip chains and the research questions that have been addressed are explored. This review of previous research into trip chaining facilitates the following tasks: the identification of the most useful questions to be addressed by this research; defining trip chaining and associated typologies and defining data structures to extract trip chaining information from the household travel surveys conducted in metropolitan Adelaide, South Australia. The definition and typology developed in our research was then used to extract trip-chaining information from the household travel diary survey (MAHTS99) conducted in Adelaide in 1999. The extracted trip chaining information was then used to investigate trip-chaining behaviour by households. The paper reports the results of this analysis and concludes with a summary of the findings and recommendations for further investigations.
This is a preview of subscription content, log in via an institution to check access.
Access this article
Price includes VAT (Russian Federation)
Instant access to the full article PDF.
Rent this article via DeepDyve
Institutional subscriptions
Similar content being viewed by others
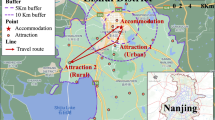
Trip chaining patterns of tourists: a real-world case study
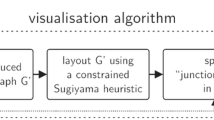
Visualisation of trip chaining behaviour and mode choice using household travel survey data
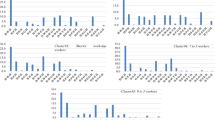
Trip chaining propensity and tour mode choice of out-of-home workers: evidence from a mid-sized Canadian city
Adams, P.C.: Application of a CAD-based accessibility model. In: Janelle, D.G., Hodge, D.C. (eds.) Information, Place, and Cyberspace: Issues in Accessibility, pp. 217–239. Berlin, Springer, Berlin (2000)
Google Scholar
Bowman, J.L., Ben-Akiva, M.E.: Activity-based disaggregate travel demand model system with activity schedules. Transport. Res. A 35 , 1–28 (2001)
Article Google Scholar
Clarke, M.L.: Household activity-travel patterns study in Adelaide: Documentation of the PISA technique. Stage 2: Report 3. Transport Studies Unit, Oxford University, December (1987)
Davidson, D.: Impact of suburban employee trip chaining on transportation demand management. Transport. Res. Record 1321 , 82–89 (1991)
D’Este, G.: A technique for incorporating the effect of changing patterns of travel behaviour into the traditional transport planning paradigm. J Eastern Asia Society Transport. Stud. 2 , 1099–1111 (1997)
Forer, P., Huisman, O.: Space, time and sequencing: Substitution of the physical virtual interface. In: Janelle, D.G., Hodge, D.C. (eds.) Information, Place, and Cyberspace: Issues in Accessibility, pp. 73–90. Berlin, Springer (2000)
Golob, T.F.: A nonlinear canonical correlation analysis of weekly trip chaining behaviour. Transport. Res. A 24 , 385–399 (1986)
Goulias, K.G., Kitamura, R.: Recursive model system for trip generation and trip chaining. Transport. Res. Record 1236 , 59–66 (1989)
Hagerstrand, T.: What about people in regional science? Papers Regional Sci. Assoc. 14 (1970)
Hensher, D.A., Reyes, A.J.: Trip chaining as a barrier to the propensity to use public transport. Transportation 27 , 341–361 (2000)
Holzapfel H.: Trip Relationships in Urban Areas. Aldershot, Gower (1986)
Jou, R.-C., Mahmassani, H.S.: Comparative analysis of day-to-day trip-chaining behavior of urban commuters in two cities. Transport. Res. Record 1607 , 163–170 (1998)
Kondo, K., Kitamura, R.: Space-time constraints and the formation of trip chains. Regional Sci. Urban Econ. 17 , 49–65 (1987)
Kwan, M.-P.: Gender, the home-work link, and space-time patterns of nonemployment activities. Econ. Geogr. 75 (4), 370–394 (1999)
Kwan, M.-P.: Human extensibility and individual hybrid accessibility in space-time: a multi-scale representation using GIS. In: Janelle, D.G., Hodge, D.C. (eds.) Information, Place, and Cyberspace: Issues in Accessibility, pp. 241–256. Berlin, Springer (2000)
McGuckin, N., Murakami, E.: Examining trip-chaining behaviour: a comparison of travel by men and women, Federal Highway Administration. Washington, DC, FHWA (1995)
Nishii, K., Kondo, K., Kitamura, R.: Empirical analysis of trip chaining behavior. Transport. Res. Record. 1203, 48–59 (1988)
Primerano, F., Taylor, M.A.P.: An accessibility framework for evaluating transport policies. In: Levinson, D., Krizek, K.J. (eds.) Access to Destinations: Rethinking the Transportation Future. Elsevier, London (2005)
Srinivasan, S.: Linking land use, transportation and travel behaviour: understanding trip chaining in terms of land use and accessibility patterns. Cambridge, Department of Urban Studies and Planning, Massachusetts Institute of Technology (1998)
Stopher, P.R., Hartgen, D.T., Li, Y-J.: SMART: simulation model for activities, resources and travel. Transportation 23 , 293–312 (1996)
Strathman, J.G., Dueker, K.J.: Understanding Trip Chaining. Special Reports on Trip and Vehicle Attributes, 1990 NPTS Report Series. Washington DC, US Department of Transportation (1995)
Strathman, J.G., Dueker, K.J., Davis, J.S.: Effects of household structure and selected travel characteristics on trip chaining. Transportation 21 , 23–45 (1994)
Thill, J.-C., Thomas, I.: Towards conceptualising trip-chaining behaviour: a review. Geogr. Anal. 19 , 1–17 (1987)
Download references
Acknowledgements
This project was a joint effort between the Transport Systems Centre (TSC) of the University of South Australia and the South Australian Department for Transport, Energy and Infrastructure (DTEI). The authors would like to thank Chris Mayer, Peter Doble and Lindsay Oxlad for their input into and support of this research. The views expressed are those of the authors, and should not be attributed to the South Australian Government.
Author information
Authors and affiliations.
Policy and Planning Agency, South Australian Department for Transport, Energy and Infrastructure, Adelaide, Australia
Frank Primerano & Peter Tisato
Transport Systems Centre, University of South Australia, GPO Box 2471, Adelaide, 5001, SA, Australia
Michael A. P. Taylor & Ladda Pitaksringkarn
You can also search for this author in PubMed Google Scholar
Corresponding author
Correspondence to Michael A. P. Taylor .
Rights and permissions
Reprints and permissions
About this article
Primerano, F., Taylor, M.A.P., Pitaksringkarn, L. et al. Defining and understanding trip chaining behaviour. Transportation 35 , 55–72 (2008). https://doi.org/10.1007/s11116-007-9134-8
Download citation
Published : 19 July 2007
Issue Date : January 2008
DOI : https://doi.org/10.1007/s11116-007-9134-8
Share this article
Anyone you share the following link with will be able to read this content:
Sorry, a shareable link is not currently available for this article.
Provided by the Springer Nature SharedIt content-sharing initiative
- Travel behaviour
- Travel survey
- Activity analysis
- Find a journal
- Publish with us
- Track your research

Page categories
Needs Review
Trip distribution
The trip distribution step (within trip-based models) determines where trips go, spatially. Trip distribution allocates trips to pairs of traffic analysis zones (TAZs), from the production (P) end to the attraction (A) end. The result is a PA table, which describes the numbers of person trips between zones (usually over a 24-hour period of time) but does not describe the actual direction of travel. Inputs to the trip distribution step come principally from the trip generation step. There is also a requirement for calibrated parameters. The number of these parameters depends upon the model formulation.
There are a variety of trip distribution formulations. Among recent travel models two formulations dominate: the gravity “model” and the destination choice “model”. There are variations to each formulation.
The gravity “model” allocates trips roughly in proportion to the number of productions at the production end, roughly in proportion to the number of attractions at the attraction end, and roughly in proportion to a measure of proximity (often called a “friction factor”) of the two zones. A gravity “model” may be “singly-constrained” or “doubly-constrained”. A singly-constrained gravity “model” assures that the PA table is consistent with trip productions from trip generation. A doubly-constrained gravity “model” also assures that the PA table is consistent with trip attractions from trip generation.
A friction factor is a function of trip impedance (time, cost, distance, etc.) between zones, with impedance usually expressed in units of travel time. There is an inverse relation between the value of a friction factor and the value of impedance. This inverse relation is largely empirical in practice, although theories such as “entropy maximization” have been proposed to derive this relation. A functional form dubbed the “gamma” function has been recommended by NCHRP Report 365 and NCHRP Report 716; however, many travel models use a negative exponential function or a power function with a negative power. Some travel models use a table lookup to obtain friction factors.
A technical discussion of the gravity model, along with example friction factors, may be found in NCHRP Report 716. An alternative to a gravity “model” is a destination choice “model”. The preferred destination choice “model” takes the form of a multinomial logit equation. The utility function for the destination choice “model” may contain measures of impedance (time, cost, distance, etc.) as well as a measure of attraction zone size and perhaps socioeconomic variables. A singly-constraint gravity "model" containing a negative exponential friction factor function is very similar to a destination choice "model".
Trip distribution for multimodal forecasts needs a combined impedance across all modes that reflect the various mode shares. A feedback from mode split to trip distribution is not usually accomplished. Rather, multimodal trip distribution uses a “combined impedance” for all modes which is calculated as a “log sum”, similar to what is used in a nested logit formulation. See NCHRP Report 716 for a discussion about “log sum”.
← Trip Generation Destination choice models →
This site uses cookies to learn which topics interest our readers.
Multi-source Data-driven Procedure for Traffic Analysis Zones Definition
Ieee account.
- Change Username/Password
- Update Address
Purchase Details
- Payment Options
- Order History
- View Purchased Documents
Profile Information
- Communications Preferences
- Profession and Education
- Technical Interests
- US & Canada: +1 800 678 4333
- Worldwide: +1 732 981 0060
- Contact & Support
- About IEEE Xplore
- Accessibility
- Terms of Use
- Nondiscrimination Policy
- Privacy & Opting Out of Cookies
A not-for-profit organization, IEEE is the world's largest technical professional organization dedicated to advancing technology for the benefit of humanity. © Copyright 2024 IEEE - All rights reserved. Use of this web site signifies your agreement to the terms and conditions.
- Accessibility Policy
- Skip to content
- QUICK LINKS
- Oracle Cloud Infrastructure
- Oracle Fusion Cloud Applications
- Download Java
- Careers at Oracle
What Is Big Data?
Sherry Tiao | Senior Manager, AI & Analytics, Oracle | March 11, 2024

In This Article
Big Data Defined
The three “vs” of big data, the value—and truth—of big data, the history of big data, big data use cases, big data challenges, how big data works, big data best practices.
What exactly is big data?
The definition of big data is data that contains greater variety, arriving in increasing volumes and with more velocity. This is also known as the three “Vs.”
Put simply, big data is larger, more complex data sets, especially from new data sources. These data sets are so voluminous that traditional data processing software just can’t manage them. But these massive volumes of data can be used to address business problems you wouldn’t have been able to tackle before.
Two more Vs have emerged over the past few years: value and veracity . Data has intrinsic value. But it’s of no use until that value is discovered. Equally important: How truthful is your data—and how much can you rely on it?
Today, big data has become capital. Think of some of the world’s biggest tech companies. A large part of the value they offer comes from their data, which they’re constantly analyzing to produce more efficiency and develop new products.
Recent technological breakthroughs have exponentially reduced the cost of data storage and compute, making it easier and less expensive to store more data than ever before. With an increased volume of big data now cheaper and more accessible, you can make more accurate and precise business decisions.
Finding value in big data isn’t only about analyzing it (which is a whole other benefit). It’s an entire discovery process that requires insightful analysts, business users, and executives who ask the right questions, recognize patterns, make informed assumptions, and predict behavior.
But how did we get here?
Although the concept of big data itself is relatively new, the origins of large data sets go back to the 1960s and ‘70s when the world of data was just getting started with the first data centers and the development of the relational database.
Around 2005, people began to realize just how much data users generated through Facebook, YouTube, and other online services. Hadoop (an open source framework created specifically to store and analyze big data sets) was developed that same year. NoSQL also began to gain popularity during this time.
The development of open source frameworks, such as Hadoop (and more recently, Spark) was essential for the growth of big data because they make big data easier to work with and cheaper to store. In the years since then, the volume of big data has skyrocketed. Users are still generating huge amounts of data—but it’s not just humans who are doing it.
With the advent of the Internet of Things (IoT), more objects and devices are connected to the internet, gathering data on customer usage patterns and product performance. The emergence of machine learning has produced still more data.
While big data has come far, its usefulness is only just beginning. Cloud computing has expanded big data possibilities even further. The cloud offers truly elastic scalability, where developers can simply spin up ad hoc clusters to test a subset of data. And graph databases are becoming increasingly important as well, with their ability to display massive amounts of data in a way that makes analytics fast and comprehensive.

Discover the Insights in Your Data
- Who are the criminals passing dirty money around and committing financial services fraud?
- Who has been in contact with an infected person and needs to go into quarantine?
- How can feature engineering for data science be made simpler and more efficient?
Click below to access the 17 Use Cases for Graph Databases and Graph Analytics ebook.
Big Data Benefits
- Big data makes it possible for you to gain more complete answers because you have more information.
- More complete answers mean more confidence in the data—which means a completely different approach to tackling problems.
Big data can help you address a range of business activities, including customer experience and analytics. Here are just a few.

Download your free ebook to learn about:
- New ways you can use your data
- Ways the competition could be innovating
- Benefits and challenges of different use cases
While big data holds a lot of promise, it is not without its challenges.
First, big data is…big. Although new technologies have been developed for data storage, data volumes are doubling in size about every two years. Organizations still struggle to keep pace with their data and find ways to effectively store it.
But it’s not enough to just store the data. Data must be used to be valuable and that depends on curation. Clean data, or data that’s relevant to the client and organized in a way that enables meaningful analysis, requires a lot of work. Data scientists spend 50 to 80 percent of their time curating and preparing data before it can actually be used.
Finally, big data technology is changing at a rapid pace. A few years ago, Apache Hadoop was the popular technology used to handle big data. Then Apache Spark was introduced in 2014. Today, a combination of the two frameworks appears to be the best approach. Keeping up with big data technology is an ongoing challenge.
Discover more big data resources:
Big data gives you new insights that open up new opportunities and business models. Getting started involves three key actions:
1. Integrate Big data brings together data from many disparate sources and applications. Traditional data integration mechanisms, such as extract, transform, and load (ETL) generally aren’t up to the task. It requires new strategies and technologies to analyze big data sets at terabyte, or even petabyte, scale.
During integration, you need to bring in the data, process it, and make sure it’s formatted and available in a form that your business analysts can get started with.
2. Manage Big data requires storage. Your storage solution can be in the cloud, on premises, or both. You can store your data in any form you want and bring your desired processing requirements and necessary process engines to those data sets on an on-demand basis. Many people choose their storage solution according to where their data is currently residing. The cloud is gradually gaining popularity because it supports your current compute requirements and enables you to spin up resources as needed.
3. Analyze Your investment in big data pays off when you analyze and act on your data. Get new clarity with a visual analysis of your varied data sets. Explore the data further to make new discoveries. Share your findings with others. Build data models with machine learning and artificial intelligence. Put your data to work.
To help you on your big data journey, we’ve put together some key best practices for you to keep in mind. Here are our guidelines for building a successful big data foundation.
Learn More About Big Data at Oracle
- Try a free big data workshop
- Infographic: How to Build Effective Data Lakes
The state of AI in early 2024: Gen AI adoption spikes and starts to generate value
If 2023 was the year the world discovered generative AI (gen AI) , 2024 is the year organizations truly began using—and deriving business value from—this new technology. In the latest McKinsey Global Survey on AI, 65 percent of respondents report that their organizations are regularly using gen AI, nearly double the percentage from our previous survey just ten months ago. Respondents’ expectations for gen AI’s impact remain as high as they were last year , with three-quarters predicting that gen AI will lead to significant or disruptive change in their industries in the years ahead.
About the authors
This article is a collaborative effort by Alex Singla , Alexander Sukharevsky , Lareina Yee , and Michael Chui , with Bryce Hall , representing views from QuantumBlack, AI by McKinsey, and McKinsey Digital.
Organizations are already seeing material benefits from gen AI use, reporting both cost decreases and revenue jumps in the business units deploying the technology. The survey also provides insights into the kinds of risks presented by gen AI—most notably, inaccuracy—as well as the emerging practices of top performers to mitigate those challenges and capture value.
AI adoption surges
Interest in generative AI has also brightened the spotlight on a broader set of AI capabilities. For the past six years, AI adoption by respondents’ organizations has hovered at about 50 percent. This year, the survey finds that adoption has jumped to 72 percent (Exhibit 1). And the interest is truly global in scope. Our 2023 survey found that AI adoption did not reach 66 percent in any region; however, this year more than two-thirds of respondents in nearly every region say their organizations are using AI. 1 Organizations based in Central and South America are the exception, with 58 percent of respondents working for organizations based in Central and South America reporting AI adoption. Looking by industry, the biggest increase in adoption can be found in professional services. 2 Includes respondents working for organizations focused on human resources, legal services, management consulting, market research, R&D, tax preparation, and training.
Also, responses suggest that companies are now using AI in more parts of the business. Half of respondents say their organizations have adopted AI in two or more business functions, up from less than a third of respondents in 2023 (Exhibit 2).
Gen AI adoption is most common in the functions where it can create the most value
Most respondents now report that their organizations—and they as individuals—are using gen AI. Sixty-five percent of respondents say their organizations are regularly using gen AI in at least one business function, up from one-third last year. The average organization using gen AI is doing so in two functions, most often in marketing and sales and in product and service development—two functions in which previous research determined that gen AI adoption could generate the most value 3 “ The economic potential of generative AI: The next productivity frontier ,” McKinsey, June 14, 2023. —as well as in IT (Exhibit 3). The biggest increase from 2023 is found in marketing and sales, where reported adoption has more than doubled. Yet across functions, only two use cases, both within marketing and sales, are reported by 15 percent or more of respondents.
Gen AI also is weaving its way into respondents’ personal lives. Compared with 2023, respondents are much more likely to be using gen AI at work and even more likely to be using gen AI both at work and in their personal lives (Exhibit 4). The survey finds upticks in gen AI use across all regions, with the largest increases in Asia–Pacific and Greater China. Respondents at the highest seniority levels, meanwhile, show larger jumps in the use of gen Al tools for work and outside of work compared with their midlevel-management peers. Looking at specific industries, respondents working in energy and materials and in professional services report the largest increase in gen AI use.
Investments in gen AI and analytical AI are beginning to create value
The latest survey also shows how different industries are budgeting for gen AI. Responses suggest that, in many industries, organizations are about equally as likely to be investing more than 5 percent of their digital budgets in gen AI as they are in nongenerative, analytical-AI solutions (Exhibit 5). Yet in most industries, larger shares of respondents report that their organizations spend more than 20 percent on analytical AI than on gen AI. Looking ahead, most respondents—67 percent—expect their organizations to invest more in AI over the next three years.
Where are those investments paying off? For the first time, our latest survey explored the value created by gen AI use by business function. The function in which the largest share of respondents report seeing cost decreases is human resources. Respondents most commonly report meaningful revenue increases (of more than 5 percent) in supply chain and inventory management (Exhibit 6). For analytical AI, respondents most often report seeing cost benefits in service operations—in line with what we found last year —as well as meaningful revenue increases from AI use in marketing and sales.
Inaccuracy: The most recognized and experienced risk of gen AI use
As businesses begin to see the benefits of gen AI, they’re also recognizing the diverse risks associated with the technology. These can range from data management risks such as data privacy, bias, or intellectual property (IP) infringement to model management risks, which tend to focus on inaccurate output or lack of explainability. A third big risk category is security and incorrect use.
Respondents to the latest survey are more likely than they were last year to say their organizations consider inaccuracy and IP infringement to be relevant to their use of gen AI, and about half continue to view cybersecurity as a risk (Exhibit 7).
Conversely, respondents are less likely than they were last year to say their organizations consider workforce and labor displacement to be relevant risks and are not increasing efforts to mitigate them.
In fact, inaccuracy— which can affect use cases across the gen AI value chain , ranging from customer journeys and summarization to coding and creative content—is the only risk that respondents are significantly more likely than last year to say their organizations are actively working to mitigate.
Some organizations have already experienced negative consequences from the use of gen AI, with 44 percent of respondents saying their organizations have experienced at least one consequence (Exhibit 8). Respondents most often report inaccuracy as a risk that has affected their organizations, followed by cybersecurity and explainability.
Our previous research has found that there are several elements of governance that can help in scaling gen AI use responsibly, yet few respondents report having these risk-related practices in place. 4 “ Implementing generative AI with speed and safety ,” McKinsey Quarterly , March 13, 2024. For example, just 18 percent say their organizations have an enterprise-wide council or board with the authority to make decisions involving responsible AI governance, and only one-third say gen AI risk awareness and risk mitigation controls are required skill sets for technical talent.
Bringing gen AI capabilities to bear
The latest survey also sought to understand how, and how quickly, organizations are deploying these new gen AI tools. We have found three archetypes for implementing gen AI solutions : takers use off-the-shelf, publicly available solutions; shapers customize those tools with proprietary data and systems; and makers develop their own foundation models from scratch. 5 “ Technology’s generational moment with generative AI: A CIO and CTO guide ,” McKinsey, July 11, 2023. Across most industries, the survey results suggest that organizations are finding off-the-shelf offerings applicable to their business needs—though many are pursuing opportunities to customize models or even develop their own (Exhibit 9). About half of reported gen AI uses within respondents’ business functions are utilizing off-the-shelf, publicly available models or tools, with little or no customization. Respondents in energy and materials, technology, and media and telecommunications are more likely to report significant customization or tuning of publicly available models or developing their own proprietary models to address specific business needs.
Respondents most often report that their organizations required one to four months from the start of a project to put gen AI into production, though the time it takes varies by business function (Exhibit 10). It also depends upon the approach for acquiring those capabilities. Not surprisingly, reported uses of highly customized or proprietary models are 1.5 times more likely than off-the-shelf, publicly available models to take five months or more to implement.
Gen AI high performers are excelling despite facing challenges
Gen AI is a new technology, and organizations are still early in the journey of pursuing its opportunities and scaling it across functions. So it’s little surprise that only a small subset of respondents (46 out of 876) report that a meaningful share of their organizations’ EBIT can be attributed to their deployment of gen AI. Still, these gen AI leaders are worth examining closely. These, after all, are the early movers, who already attribute more than 10 percent of their organizations’ EBIT to their use of gen AI. Forty-two percent of these high performers say more than 20 percent of their EBIT is attributable to their use of nongenerative, analytical AI, and they span industries and regions—though most are at organizations with less than $1 billion in annual revenue. The AI-related practices at these organizations can offer guidance to those looking to create value from gen AI adoption at their own organizations.
To start, gen AI high performers are using gen AI in more business functions—an average of three functions, while others average two. They, like other organizations, are most likely to use gen AI in marketing and sales and product or service development, but they’re much more likely than others to use gen AI solutions in risk, legal, and compliance; in strategy and corporate finance; and in supply chain and inventory management. They’re more than three times as likely as others to be using gen AI in activities ranging from processing of accounting documents and risk assessment to R&D testing and pricing and promotions. While, overall, about half of reported gen AI applications within business functions are utilizing publicly available models or tools, gen AI high performers are less likely to use those off-the-shelf options than to either implement significantly customized versions of those tools or to develop their own proprietary foundation models.
What else are these high performers doing differently? For one thing, they are paying more attention to gen-AI-related risks. Perhaps because they are further along on their journeys, they are more likely than others to say their organizations have experienced every negative consequence from gen AI we asked about, from cybersecurity and personal privacy to explainability and IP infringement. Given that, they are more likely than others to report that their organizations consider those risks, as well as regulatory compliance, environmental impacts, and political stability, to be relevant to their gen AI use, and they say they take steps to mitigate more risks than others do.
Gen AI high performers are also much more likely to say their organizations follow a set of risk-related best practices (Exhibit 11). For example, they are nearly twice as likely as others to involve the legal function and embed risk reviews early on in the development of gen AI solutions—that is, to “ shift left .” They’re also much more likely than others to employ a wide range of other best practices, from strategy-related practices to those related to scaling.
In addition to experiencing the risks of gen AI adoption, high performers have encountered other challenges that can serve as warnings to others (Exhibit 12). Seventy percent say they have experienced difficulties with data, including defining processes for data governance, developing the ability to quickly integrate data into AI models, and an insufficient amount of training data, highlighting the essential role that data play in capturing value. High performers are also more likely than others to report experiencing challenges with their operating models, such as implementing agile ways of working and effective sprint performance management.
About the research
The online survey was in the field from February 22 to March 5, 2024, and garnered responses from 1,363 participants representing the full range of regions, industries, company sizes, functional specialties, and tenures. Of those respondents, 981 said their organizations had adopted AI in at least one business function, and 878 said their organizations were regularly using gen AI in at least one function. To adjust for differences in response rates, the data are weighted by the contribution of each respondent’s nation to global GDP.
Alex Singla and Alexander Sukharevsky are global coleaders of QuantumBlack, AI by McKinsey, and senior partners in McKinsey’s Chicago and London offices, respectively; Lareina Yee is a senior partner in the Bay Area office, where Michael Chui , a McKinsey Global Institute partner, is a partner; and Bryce Hall is an associate partner in the Washington, DC, office.
They wish to thank Kaitlin Noe, Larry Kanter, Mallika Jhamb, and Shinjini Srivastava for their contributions to this work.
This article was edited by Heather Hanselman, a senior editor in McKinsey’s Atlanta office.
Explore a career with us
Related articles.

Moving past gen AI’s honeymoon phase: Seven hard truths for CIOs to get from pilot to scale
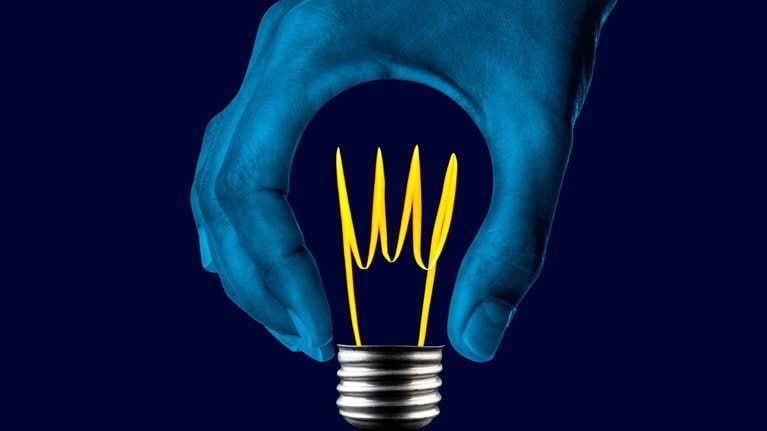
A generative AI reset: Rewiring to turn potential into value in 2024

Implementing generative AI with speed and safety
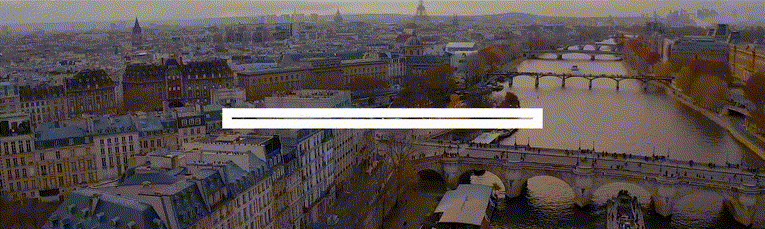
IMAGES
VIDEO
COMMENTS
Trip Generation is the first step in the conventional four-step transportation forecasting process (followed by Destination Choice, Mode Choice, and Route Choice), widely used for forecasting travel demands. It predicts the number of trips originating in or destined for a particular traffic analysis zone.
Pass-By and Diverted Number of Trips. Use either local data or ITE data to determine a percentage of the reduced trip generation that is pass-by or diverted. Similar to the ITE Trip Generation data, both pass-by and diverted trip percentages are available by average rate or an equation for many land uses. Use this percentage to calculate the ...
Trip generation is the first step in the conventional four-step transportation forecasting process used for forecasting travel demands. It predicts the number of trips originating in or destined for a particular traffic analysis zone (TAZ). Trip generation analysis focuses on residences and residential trip generation is thought of as a function of the social and economic attributes of households.
Since people traveling for different reasons behave differently, four-step models segment trips by trip purpose. The number and definition of trip purposes in a model depend on the types of information the model needs to provide for planning analyses, the char- acteristics of the region being modeled, and the availability of data with which to ...
Trip generation is estimated in three ways: (i) traditionally by linear and multiple regression. (ii) by aggregating the trip generating capability of a household or car and aggregating the total according to the distribution of each selected category in the zones, and. (iii) by household classification method through a catalogue of the ...
Trip Generation Analysis. The following excerpt was taken from the Transportation Planning Handbook published in 1992 by the Institute of Transportation Engineers (pp. 108-112). Trip Generation Models. (p. 110) There are two kinds of trip generation models: production models and attraction models. Trip production models estimate the number of ...
Trip Generation is the first step in the conventional four-step transportation forecasting process (followed by Destination Choice, Mode Choice, and Route Choice), widely used for forecasting travel demands.It predicts the number of trips originating in or destined for a particular traffic analysis zone. Every trip has two ends, and we need to know where both of them are. The first part is ...
Trip Generation is the first step in the Sequential Demand Modelling arrangement which is also called as the Four Step Transportation Planning Process (FSTP) as mentioned earlier. In order to carry out modelling, the variable consists of total number of person-trips generated by a zone as a dependent variable and the independent variable ...
This chapter describes the second step, trip distribution, of the four-step travel demand modeling (FSM). This step focuses on the procedure that distributes the trips after trip generation has been modeled, meaning after trips generated from or attracted to each zone in the study area are understood. The input for this step of FSM is the ...
Cross-Classification. The three major techniques used for Trip Generation Analysis are Cross-Classification, Multiple Regression Analysis, and Experience Based Analysis. Cross-Classification procedures measure the changes in one variable (trips) when other variables (land use etc.) are accounted for. Cross-Classification resembles multiple ...
Trip Generation Definition . Trip Generation is a type of transportation forecasting that predicts the number of trips originating in or destined for a particular traffic analysis zone. The purpose of a trip generation is to assist the Director of Traffic in determining whether a Traffic Impact Analysis will be required; although,
Analysis of 2001 NHTS long-distance trip data showed a sizeable sample of 50â 100 mile trips (21,500 out of 45,000 trips, or nearly 48 percent of the 2001 NHTS long-distance sample), such that exclusion of these trips for NHTS 2001 statistics would be problematic. ... Since the definition of rural varies somewhat from one NHTS attribute ...
Trip distribution. All trips have an origin and destination and these are considered at the trip distribution stage. Trip distribution (or destination choice or zonal interchange analysis) is the second component (after trip generation, but before mode choice and route assignment) in the traditional four-step transportation forecasting model.
The goal of trip generation is to predict the number of trips, by purpose, that are generated by and attracted to each zone in a study area. Trip generation is performed by relating the number or frequency of trips to the characteristics of the individuals, of the zone, and of the transportation network. The zone that contains the home end of ...
This procedure is called trip generation, and it estimates the number of "trip ends" produced in and/or attracted to each transportation analysis zone (TAZ) in the region. Each trip is made of two "trip ends," one at the production end of the trip and one at the attraction end of the trip. In the TPB model, eight trip purposes are modeled.
The results of trip assignment analysis can be: The traffic flows in the transportation system and the pattern of vehicular movements. The volume of traffic on network links. Travel costs between trip origins and destinations (O-D). Aggregated network metrics such as total vehicle flow, vehicle miles traveled (VMT), and vehicle travel time (VTT).
Introduction. Transportation planning and policy analysis heavily rely on travel demand modeling to assess different policy scenarios and inform decision-making processes. Throughout our discussion, we have primarily explored the connection between urban activities, represented as land uses, and travel demands, represented by improvements and interventions in transportation infrastructure.
Trip Reports. Aggregated lists of anonymized trips with route details and waypoints. Trip Matrices. Compiled according to customer Traffic Analysis Zones Flexible Analysis Tools. Define your study area via shapefile or user defined zones or customize your analysis by mode, time, or origin/destination. Offline Data Download
More than 80% of the tourists have an average travel time less than 1 h, while the total travel time mostly ranges from < 1 h to 2-3 h. 44.25% of the tourists stop at each attraction for 2-3 h on average, while the total activity time of 2-4 h and 4-6 h accounted for the most with 34.77% and 37.18% respectively.
Trip chaining is a phenomenon that we know exists but rarely investigate. This could be attributed to either the difficulty in defining trip chains, extracting such information from travel diary surveys, the difficulty in analysing all the possible trip chain types, or all of the above. Household travel diary surveys provide a wealth of information on the travel patterns of individuals and ...
The trip distribution step (within trip-based models) determines where trips go, spatially. Trip distribution allocates trips to pairs of traffic analysis zones (TAZs), from the production (P) end to the attraction (A) end. The result is a PA table, which describes the numbers of person trips between zones (usually over a 24-hour period of time) but does not describe the actual direction of ...
The choice of an appropriate Traffic Analysis Zones (TAZ) system is a critical step of travel demand modeling which is often overlooked. Studies have approached this process as an optimization problem aiming to maximize the homogeneity of socio-economic and geographic characteristics of zones while minimizing, at the same time, the number of intrazonal trips. However, beyond the application of ...
Testing means that element of inspection that determines the properties or elements, including functional operation of materials, equipment, or their components, by the application of established scientific principles and procedures. Define Trip Analysis. means a study or report that specifies the net trips from a regulated activity and ...
What Is Data Analysis? (With Examples) Data analysis is the practice of working with data to glean useful information, which can then be used to make informed decisions. "It is a capital mistake to theorize before one has data. Insensibly one begins to twist facts to suit theories, instead of theories to suit facts," Sherlock Holme's proclaims ...
Marketing analytics is a crucial way to develop stronger, more data-informed marketing strategies. Learn more about the tools and skills you'll need to know to work with marketing analytics. The vast majority of businesses use data to make more informed decisions across a wide array of functions. Marketing analytics is part of that trend.
Machine learning definition. Machine learning is a subfield of artificial intelligence (AI) that uses algorithms trained on data sets to create self-learning models that are capable of predicting outcomes and classifying information without human intervention. Machine learning is used today for a wide range of commercial purposes, including ...
The definition of big data is data that contains greater variety, arriving in increasing volumes and with more velocity. This is also known as the three "Vs.". Put simply, big data is larger, more complex data sets, especially from new data sources. These data sets are so voluminous that traditional data processing software just can't ...
But Trump is no longer a New York resident. In Florida, felons lose civil rights, including the ability to hold public office and serve on a jury. While he can't hold office in Florida or New ...
A data analyst is a person whose job is to gather and interpret data in order to solve a specific problem. The role includes plenty of time spent with data but entails communicating findings too. Here's what many data analysts do on a day-to-day basis: Gather data: Analysts often collect data themselves. This could include conducting surveys ...
If 2023 was the year the world discovered generative AI (gen AI), 2024 is the year organizations truly began using—and deriving business value from—this new technology. In the latest McKinsey Global Survey on AI, 65 percent of respondents report that their organizations are regularly using gen AI, nearly double the percentage from our ...