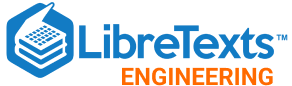
- school Campus Bookshelves
- menu_book Bookshelves
- perm_media Learning Objects
- login Login
- how_to_reg Request Instructor Account
- hub Instructor Commons
- Download Page (PDF)
- Download Full Book (PDF)
- Periodic Table
- Physics Constants
- Scientific Calculator
- Reference & Cite
- Tools expand_more
- Readability
selected template will load here
This action is not available.
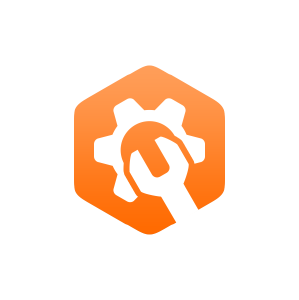
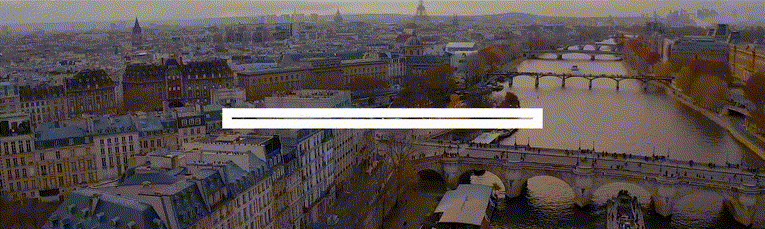
3.4: Trip Generation
- Last updated
- Save as PDF
- Page ID 47326
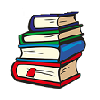
- David Levinson et al.
- Associate Professor (Engineering) via Wikipedia
Trip Generation is the first step in the conventional four-step transportation forecasting process (followed by Destination Choice, Mode Choice, and Route Choice), widely used for forecasting travel demands. It predicts the number of trips originating in or destined for a particular traffic analysis zone.
Every trip has two ends, and we need to know where both of them are. The first part is determining how many trips originate in a zone and the second part is how many trips are destined for a zone. Because land use can be divided into two broad category (residential and non-residential) we have models that are household based and non-household based (e.g. a function of number of jobs or retail activity).
For the residential side of things, trip generation is thought of as a function of the social and economic attributes of households (households and housing units are very similar measures, but sometimes housing units have no households, and sometimes they contain multiple households, clearly housing units are easier to measure, and those are often used instead for models, it is important to be clear which assumption you are using).
At the level of the traffic analysis zone, the language is that of land uses "producing" or attracting trips, where by assumption trips are "produced" by households and "attracted" to non-households. Production and attractions differ from origins and destinations. Trips are produced by households even when they are returning home (that is, when the household is a destination). Again it is important to be clear what assumptions you are using.
People engage in activities, these activities are the "purpose" of the trip. Major activities are home, work, shop, school, eating out, socializing, recreating, and serving passengers (picking up and dropping off). There are numerous other activities that people engage on a less than daily or even weekly basis, such as going to the doctor, banking, etc. Often less frequent categories are dropped and lumped into the catchall "Other".
Every trip has two ends, an origin and a destination. Trips are categorized by purposes , the activity undertaken at a destination location.
Observed trip making from the Twin Cities (2000-2001) Travel Behavior Inventory by Gender
Some observations:
- Men and women behave differently on average, splitting responsibilities within households, and engaging in different activities,
- Most trips are not work trips, though work trips are important because of their peaked nature (and because they tend to be longer in both distance and travel time),
- The vast majority of trips are not people going to (or from) work.
People engage in activities in sequence, and may chain their trips. In the Figure below, the trip-maker is traveling from home to work to shop to eating out and then returning home.

Specifying Models
How do we predict how many trips will be generated by a zone? The number of trips originating from or destined to a purpose in a zone are described by trip rates (a cross-classification by age or demographics is often used) or equations. First, we need to identify what we think the relevant variables are.
The total number of trips leaving or returning to homes in a zone may be described as a function of:
\[T_h = f(housing \text{ }units, household \text{ }size, age, income, accessibility, vehicle \text{ }ownership)\]
Home-End Trips are sometimes functions of:
- Housing Units
- Household Size
- Accessibility
- Vehicle Ownership
- Other Home-Based Elements
At the work-end of work trips, the number of trips generated might be a function as below:
\[T_w=f(jobs(area \text{ }of \text{ } space \text{ } by \text{ } type, occupancy \text{ } rate\]
Work-End Trips are sometimes functions of:
- Area of Workspace
- Occupancy Rate
- Other Job-Related Elements
Similarly shopping trips depend on a number of factors:
\[T_s = f(number \text{ }of \text{ }retail \text{ }workers, type \text{ }of \text{ }retail, area, location, competition)\]
Shop-End Trips are sometimes functions of:
- Number of Retail Workers
- Type of Retail Available
- Area of Retail Available
- Competition
- Other Retail-Related Elements
A forecasting activity conducted by planners or economists, such as one based on the concept of economic base analysis, provides aggregate measures of population and activity growth. Land use forecasting distributes forecast changes in activities across traffic zones.
Estimating Models
Which is more accurate: the data or the average? The problem with averages (or aggregates) is that every individual’s trip-making pattern is different.
To estimate trip generation at the home end, a cross-classification model can be used. This is basically constructing a table where the rows and columns have different attributes, and each cell in the table shows a predicted number of trips, this is generally derived directly from data.
In the example cross-classification model: The dependent variable is trips per person. The independent variables are dwelling type (single or multiple family), household size (1, 2, 3, 4, or 5+ persons per household), and person age.
The figure below shows a typical example of how trips vary by age in both single-family and multi-family residence types.

The figure below shows a moving average.

Non-home-end
The trip generation rates for both “work” and “other” trip ends can be developed using Ordinary Least Squares (OLS) regression (a statistical technique for fitting curves to minimize the sum of squared errors (the difference between predicted and actual value) relating trips to employment by type and population characteristics.
The variables used in estimating trip rates for the work-end are Employment in Offices (\(E_{off}\)), Retail (\(E_{ret}\)), and Other (\(E_{oth}\))
A typical form of the equation can be expressed as:
\[T_{D,k}=a_1E_{off,k}+a_2E_{oth,k}+a_3E_{ret,k}\]
- \(T_{D,k}\) - Person trips attracted per worker in Zone k
- \(E_{off,i}\) - office employment in the ith zone
- \(E_{oth,i}\) - other employment in the ith zone
- \(E_{ret,i}\)- retail employment in the ith zone
- \(a_1,a_2,a_3\) - model coefficients
Normalization
For each trip purpose (e.g. home to work trips), the number of trips originating at home must equal the number of trips destined for work. Two distinct models may give two results. There are several techniques for dealing with this problem. One can either assume one model is correct and adjust the other, or split the difference.
It is necessary to ensure that the total number of trip origins equals the total number of trip destinations, since each trip interchange by definition must have two trip ends.
The rates developed for the home end are assumed to be most accurate,
The basic equation for normalization:
\[T'_{D,j}=T_{D,j} \dfrac{ \displaystyle \sum{i=1}^I T_{O,i}}{\displaystyle \sum{j=1}^J T_{TD,j}}\]
Sample Problems
Planners have estimated the following models for the AM Peak Hour
\(T_{O,i}=1.5*H_i\)
\(T_{D,j}=(1.5*E_{off,j})+(1*E_{oth,j})+(0.5*E_{ret,j})\)
\(T_{O,i}\) = Person Trips Originating in Zone \(i\)
\(T_{D,j}\) = Person Trips Destined for Zone \(j\)
\(H_i\) = Number of Households in Zone \(i\)
You are also given the following data
A. What are the number of person trips originating in and destined for each city?
B. Normalize the number of person trips so that the number of person trip origins = the number of person trip destinations. Assume the model for person trip origins is more accurate.
Solution to Trip Generation Problem Part A
\[T'_{D,j}=T_{D,j} \dfrac{ \displaystyle \sum{i=1}^I T_{O,i}}{\displaystyle \sum{j=1}^J T_{TD,j}}=>T_{D,j} \dfrac{37500}{36750}=T_{D,j}*1.0204\]
Solution to Trip Generation Problem Part B
Modelers have estimated that the number of trips leaving Rivertown (\(T_O\)) is a function of the number of households (H) and the number of jobs (J), and the number of trips arriving in Marcytown (\(T_D\)) is also a function of the number of households and number of jobs.
\(T_O=1H+0.1J;R^2=0.9\)
\(T_D=0.1H+1J;R^2=0.5\)
Assuming all trips originate in Rivertown and are destined for Marcytown and:
Rivertown: 30000 H, 5000 J
Marcytown: 6000 H, 29000 J
Determine the number of trips originating in Rivertown and the number destined for Marcytown according to the model.
Which number of origins or destinations is more accurate? Why?
T_Rivertown =T_O ; T_O= 1(30000) + 0.1(5000) = 30500 trips
T_(MarcyTown)=T_D ; T_D= 0.1(6000) + 1(29000) = 29600 trips
Origins(T_{Rivertown}) because of the goodness of fit measure of the Statistical model (R^2=0.9).
Modelers have estimated that in the AM peak hour, the number of trip origins (T_O) is a function of the number of households (H) and the number of jobs (J), and the number of trip destinations (T_D) is also a function of the number of households and number of jobs.
\(T_O=1.0H+0.1J;R^2=0.9\)
Suburbia: 30000 H, 5000 J
Urbia: 6000 H, 29000 J
1) Determine the number of trips originating in and destined for Suburbia and for Urbia according to the model.
2) Does this result make sense? Normalize the result to improve its accuracy and sensibility?
- \(T_{O,i}\) - Person trips originating in Zone i
- \(T_{D,j}\) - Person Trips destined for Zone j
- \(T_{O,i'}\) - Normalized Person trips originating in Zone i
- \(T_{D,j'}\) - Normalized Person Trips destined for Zone j
- \(T_h\) - Person trips generated at home end (typically morning origins, afternoon destinations)
- \(T_w\) - Person trips generated at work end (typically afternoon origins, morning destinations)
- \(T_s\) - Person trips generated at shop end
- \(H_i\) - Number of Households in Zone i
- \(E_{off,k}\) - office employment in Zone k
- \(E_{ret,k}\) - retail employment in Zone k
- \(E_{oth,k}\) - other employment in Zone k
- \(B_n\) - model coefficients
Abbreviations
- H2W - Home to work
- W2H - Work to home
- W2O - Work to other
- O2W - Other to work
- H2O - Home to other
- O2H - Other to home
- O2O - Other to other
- HBO - Home based other (includes H2O, O2H)
- HBW - Home based work (H2W, W2H)
- NHB - Non-home based (O2W, W2O, O2O)
External Exercises
Use the ADAM software at the STREET website and try Assignment #1 to learn how changes in analysis zone characteristics generate additional trips on the network.
Additional Problems
- the start and end time (to the nearest minute)
- start and end location of each trip,
- primary mode you took (drive alone, car driver with passenger, car passenger, bus, LRT, walk, bike, motorcycle, taxi, Zipcar, other). (use the codes provided)
- purpose (to work, return home, work related business, shopping, family/personal business, school, church, medical/dental, vacation, visit friends or relatives, other social recreational, other) (use the codes provided)
- if you traveled with anyone else, and if so whether they lived in your household or not.
Bonus: Email your professor at the end of everyday with a detailed log of your travel diary. (+5 points on the first exam)
- Are number of destinations always less than origins?
- Pose 5 hypotheses about factors that affect work, non-work trips? How do these factors affect accuracy, and thus normalization?
- What is the acceptable level of error?
- Describe one variable used in trip generation and how it affects the model.
- What is the basic equation for normalization?
- Which of these models (home-end, work-end) are assumed to be more accurate? Why is it important to normalize trip generation models
- What are the different trip purposes/types trip generation?
- Why is it difficult to know who is traveling when?
- What share of trips during peak afternoon peak periods are work to home (>50%, <50%?), why?
- What does ORIO abbreviate?
- What types of employees (ORIO) are more likely to travel from work to home in the evening peak
- What does the trip rate tell us about various parts of the population?
- What does the “T-statistic” value tell us about the trip rate estimation?
- Why might afternoon work to home trips be more or less than morning home to work trips? Why might the percent of trips be different?
- Define frequency.
- Why do individuals > 65 years of age make fewer work to home trips?
- Solve the following problem. You have the following trip generation model:
\[Trips=B_1Off+B_2Ind+B_3Ret\]
And you are given the following coefficients derived from a regression model.
If there are 600 office employees, 300 industrial employees, and 200 retail employees, how many trips are going from work to home?
Fundamentals of Transportation/Trip Generation
Trip Generation is the first step in the conventional four-step transportation forecasting process (followed by Destination Choice , Mode Choice , and Route Choice ), widely used for forecasting travel demands. It predicts the number of trips originating in or destined for a particular traffic analysis zone.
Every trip has two ends, and we need to know where both of them are. The first part is determining how many trips originate in a zone and the second part is how many trips are destined for a zone. Because land use can be divided into two broad category (residential and non-residential) we have models that are household based and non-household based (e.g. a function of number of jobs or retail activity).
For the residential side of things, trip generation is thought of as a function of the social and economic attributes of households (households and housing units are very similar measures, but sometimes housing units have no households, and sometimes they contain multiple households, clearly housing units are easier to measure, and those are often used instead for models, it is important to be clear which assumption you are using).
At the level of the traffic analysis zone, the language is that of land uses "producing" or attracting trips, where by assumption trips are "produced" by households and "attracted" to non-households. Production and attractions differ from origins and destinations. Trips are produced by households even when they are returning home (that is, when the household is a destination). Again it is important to be clear what assumptions you are using.
- 1 Activities
- 2.1 Home-end
- 2.2 Work-end
- 2.3 Shop-end
- 3 Input Data
- 4.1 Home-end
- 4.2 Non-home-end
- 5 Normalization
- 6 Sample Problems
- 7 Variables
- 8 Abbreviations
- 9 External Exercises
- 10 Additional Problems
- 11 End Notes
- 12 Further reading
- 14 References
Activities [ edit | edit source ]
People engage in activities, these activities are the "purpose" of the trip. Major activities are home, work, shop, school, eating out, socializing, recreating, and serving passengers (picking up and dropping off). There are numerous other activities that people engage on a less than daily or even weekly basis, such as going to the doctor, banking, etc. Often less frequent categories are dropped and lumped into the catchall "Other".
Every trip has two ends, an origin and a destination. Trips are categorized by purposes , the activity undertaken at a destination location.
Some observations:
- Men and women behave differently on average, splitting responsibilities within households, and engaging in different activities,
- Most trips are not work trips, though work trips are important because of their peaked nature (and because they tend to be longer in both distance and travel time),
- The vast majority of trips are not people going to (or from) work.
People engage in activities in sequence, and may chain their trips. In the Figure below, the trip-maker is traveling from home to work to shop to eating out and then returning home.

Specifying Models [ edit | edit source ]
How do we predict how many trips will be generated by a zone? The number of trips originating from or destined to a purpose in a zone are described by trip rates (a cross-classification by age or demographics is often used) or equations. First, we need to identify what we think the relevant variables are.
Home-end [ edit | edit source ]
The total number of trips leaving or returning to homes in a zone may be described as a function of:
Home-End Trips are sometimes functions of:
- Housing Units
- Household Size
- Accessibility
- Vehicle Ownership
- Other Home-Based Elements
Work-end [ edit | edit source ]
At the work-end of work trips, the number of trips generated might be a function as below:
Work-End Trips are sometimes functions of:
- Area of Workspace
- Occupancy Rate
- Other Job-Related Elements
Shop-end [ edit | edit source ]
Similarly shopping trips depend on a number of factors:
Shop-End Trips are sometimes functions of:
- Number of Retail Workers
- Type of Retail Available
- Area of Retail Available
- Competition
- Other Retail-Related Elements
Input Data [ edit | edit source ]
A forecasting activity conducted by planners or economists, such as one based on the concept of economic base analysis, provides aggregate measures of population and activity growth. Land use forecasting distributes forecast changes in activities across traffic zones.
Estimating Models [ edit | edit source ]
Which is more accurate: the data or the average? The problem with averages (or aggregates) is that every individual’s trip-making pattern is different.
To estimate trip generation at the home end, a cross-classification model can be used. This is basically constructing a table where the rows and columns have different attributes, and each cell in the table shows a predicted number of trips, this is generally derived directly from data.
In the example cross-classification model: The dependent variable is trips per person. The independent variables are dwelling type (single or multiple family), household size (1, 2, 3, 4, or 5+ persons per household), and person age.
The figure below shows a typical example of how trips vary by age in both single-family and multi-family residence types.

The figure below shows a moving average.

Non-home-end [ edit | edit source ]
The trip generation rates for both “work” and “other” trip ends can be developed using Ordinary Least Squares (OLS) regression (a statistical technique for fitting curves to minimize the sum of squared errors (the difference between predicted and actual value) relating trips to employment by type and population characteristics.
A typical form of the equation can be expressed as:
Normalization [ edit | edit source ]
For each trip purpose (e.g. home to work trips), the number of trips originating at home must equal the number of trips destined for work. Two distinct models may give two results. There are several techniques for dealing with this problem. One can either assume one model is correct and adjust the other, or split the difference.
It is necessary to ensure that the total number of trip origins equals the total number of trip destinations, since each trip interchange by definition must have two trip ends.
The rates developed for the home end are assumed to be most accurate,
The basic equation for normalization:
Sample Problems [ edit | edit source ]
- Problem ( Solution )
Variables [ edit | edit source ]
Abbreviations [ edit | edit source ]
- H2W - Home to work
- W2H - Work to home
- W2O - Work to other
- O2W - Other to work
- H2O - Home to other
- O2H - Other to home
- O2O - Other to other
- HBO - Home based other (includes H2O, O2H)
- HBW - Home based work (H2W, W2H)
- NHB - Non-home based (O2W, W2O, O2O)
External Exercises [ edit | edit source ]
Use the ADAM software at the STREET website and try Assignment #1 to learn how changes in analysis zone characteristics generate additional trips on the network.
Additional Problems [ edit | edit source ]
- Additional Problems
End Notes [ edit | edit source ]
Further reading [ edit | edit source ].
- Trip Generation article on wikipedia
Videos [ edit | edit source ]
- Trip Generation
- Normalization
References [ edit | edit source ]
- Book:Fundamentals of Transportation
Navigation menu
- Forecasting
7 comments
Traffic Impact Study Improvements: Part 5 – When is a Trip Not a Trip?
By Mike Spack
October 27, 2015
Guest Post by Bryant Ficek, PE, PTOE, Vice President at Spack Consulting
Earlier this year, I detailed how our standard process for a Traffic Impact Study has several points of assumptions at best or guesses at worst. This post continues that discussion. Check out the “ Top 6 Ways to Pick Apart a Traffic Study ” for more on the general topic and expect more posts to follow on this subject.
Trip generation is the process of estimating the amount of traffic a proposed development will have once it is built and operating. Trip distribution is the process by which we take the raw projected traffic for a development (trip generation) and add it to the existing volumes on the transportation network. The step in-between is determining whether all the trip generation will be new to the roadway.
To start with, there are several types of trips as follows (with definition summarized from the Institute of Transportation Engineers or ITE). The figure below illustrates the different types of trips.
- Primary or New. Traffic with the specific purpose of visiting the site being studied.
- Pass-By. Traffic already on the way from an origin to a primary trip destination that will make an intermediate stop at the site being studied without a route diversion.
- Diverted. Traffic attracted to the site being studied from adjacent facilities without direct access to the site. A diverted trip example is a through trip on a freeway that diverts to an exit and a development, adding traffic to the local road but removing traffic from the freeway.
- Internal. Traffic associated with multi-use developments where trips among various land uses can be made on the site being studied without using the major street system. These trips can be made either by walking or by vehicles using internal roadways.
These different trip generation options, combined with so many different types of land uses, leads to virtually limitless possibilities for the amount and type of traffic a particular site could generate on the roadway system. As with our trip distribution column, we initially thought about testing multiple scenarios, which would be relatively easy with today’s software. At least, theoretically. To restate our collective conclusion – While interesting on a pure research level, a thicker actual traffic impact study report covering multiple results leads us down a path no one wants to go.
Furthermore, sub-dividing the raw trip generation into parts is not something that can be quantified into a “one-size fits all” equation. Given the possibilities and the limits of our collective traffic research to date, ITE provides the best procedure to follow. So this article is dedicated to reviewing that procedure, which is spelled out in ITE’s Trip Generation Handbook and Trip Generation Manual, Volume 1 . That step-by-step process is as follows:
- Raw Trip Generation. Using ITE or other land use information (try tripgeneration.org !), calculate the raw trip generation for the site.
- Pass-By and Diverted Number of Trips. Use either local data or ITE data to determine a percentage of the reduced trip generation that is pass-by or diverted. Similar to the ITE Trip Generation data, both pass-by and diverted trip percentages are available by average rate or an equation for many land uses. Use this percentage to calculate the total pass-by and diverted trips for the site.
- Pass-By and Diverted Trip Patterns. Use the existing traffic to determine how the pass-by and diverted trips will access the site.
- Pass-By and Diverted Trip Volume Adjustment. Apply the existing traffic patterns to the pass-by and diverted trips to establish the impact on the roadway system for these trips.
- Remaining Primary/New trips. Determine the remaining trip generation after reducing for internal trips and then removing the pass-by and diverted trips.
- Primary/New Trip Pattern. We discussed factors to consider for the primary/new trip distribution in Part 4 of this series.
- Primary/New Trip Volume Adjustment . Apply the trip distribution to the primary/new trips to determine the impact on the roadway system for these trips.
- Final Volumes. Combine the pass-by, diverted, and primary/new trips at each study intersection to determine the final impact of the site being studied.
We can demonstrate this process on a theoretical study site with the following characteristics:
- 17,000 square feet of office, 3,000 square feet of fast food with a drive-thru, and 10 vehicle fueling positions at a gas station with convenience market
- One driveway accesses the site off a busy road (1,000 vehicles in the p.m. peak hour)
- A highway interchange with the busy road is located just east of the site
- Trip Generation (PM Peak)
- General Office, Land Use 710 – 98 raw trips
- Fast Food with Drive Thru, Land Use 934 – 98 raw trips
- Gas Station with Convenience Market, Land Use 945 – 136 raw trips
- Internal Trips
4. Pass-By and Diverted Patterns (per the theoretical roadway data)
6. Remaining Primary/New trips:
- Office – (98 raw – 5 internal – 0 pass-by – 0 diverted) = 93 primary/new trips
- Fast Food – (98 raw – 21 internal – 43 pass-by – 23 diverted) = 11 primary/new trips
- Gas Station – (136 raw – 22 internal – 57 pass-by – 26 diverted) = 31 primary/new trips
7. Primary/New Trip Pattern (per knowledge of theoretical area)
8. Primary/New Trip Volume Adjustment
9. Final Volumes (add the pass-by, diverted, and primary/new trips together)
We don’t include all of the above example graphs in our reports. Instead, our short-hand method is a trip generation table that looks like this:
As a final note, the internal, pass-by, diverted, and new percentages are often adjusted from the base ITE information. ITE itself notes the limited amount of data available and the inherent variability in surveyed sites. The best approach, if possible, is to discuss the percentages with the governing agency to achieve agreement and buy-in before you get too far down the path in your analysis.
Did you miss the other installments of the Traffic Impact Study Improvements series? Here are the links to the other articles:
- Part 1 – Traffic Counts
- Part 2 – Would Multiple Results Help Us?
- Part 3 – All Trips are Equal, But Some Trips are More Equal Than Others
At what point would you apply a reduction for non-SOV trips such as transit? It looks like ITE would have you apply internal capture, transit and then pass-by.
very precious and informative post…thanks so much..I had found the answer of one of my question in another post of yours..thanks for sharing your experiences
Sometimes I drive right by a coffee shop and then think, hmm, I could really use a good cup of black coffee and then I turn around and go back. Is this a pass-by trip or a diverted trip (or both?). What about a cappuccino?
If the need for java hit as you approached the site, and you turned right in, this would be a standard diversion. But since you passed the driveway and turned around, ….you need to take public transit and stop burning up our resources.
Hey Mike, After you do trip gen. calcs (let’s say for a built-up year 2029) but you want to consider design year (typical 20 years) and you want to design/improve nearby roads for the design year 2049 (2029 + 20 years); do you apply same growth rate on trip generated as you would do for existing traffic? or you apply the growth rate on existing traffic, and use trip gen numbers without applying a growth rate?
No – the growth rate is not applied to the trip generation. For instance, the trips generated by a single family home isn’t going to keep growing over time. They’re assumed to be static.
My mission is to help traffic engineers, transportation planners, and other transportation professionals improve our world.
Get these blog posts sent to your email! Sign up below.
Session expired
Please log in again. The login page will open in a new tab. After logging in you can close it and return to this page.
Get our information in your Inbox!
Sign up below to stay informed

Trip generation
What is trip generation .
A trip is usually defined in transport modeling as a single journey made by an individual between two points by a specified mode of travel and for a defined purpose. Trips are often considered as productions of a particular land-use and attracted to other specified land-uses. The number of trips arises in unit time, usually for a specified zonal land use , is called the trip generation rate.
How to estimate trip generation ?
Trip generation is estimated in three ways:
(i) traditionally by linear and multiple regression
(ii) by aggregating the trip generating capability of a household or car and aggregating the total according to the distribution of each selected category in the zones, and
(iii) by household classification method through a catalogue of the characteristic mean trip rates for specific types of household.
The attraction points are identified as trip generated by work, and other purpose visits. By assigning suitable values to the independent variables of the regression equations forecasts can be made of the future trip ends for zones by either method.

Trip distribution :Trip generation estimates the number and types of trips originating and terminating in zones. Trip distribution is the process of computing the number of trips between one zone and all other. A trip matrix is drawn up with the sums of rows indicating the total number of trips originating in zone i and the sums of columns the total number of destinations attracted to zone j.
Each cell in the matrix indicates the number of trips that go from each origin zone to each destination zone. The trips on the diagonal are intra-zonal trips, trips that originate and end in the same zone. The balancing equation is implemented in a series of steps that include modeling the number of trips originating in each cases, adding in trips originating from outside the study area(external trips), and statistically balancing the origins and destinations.
This is done in the trip generation stage. But, it is essential that the step should have been completed for the trip distribution to be implemented. Two trip distribution matrices need to be distinguished. The first is the observed distribution. This is the actual number of trips that are observed traveling between each origin zone and each destination zone. It is calculated by simply enumerating the number of trips by each origin-destination combination. It is also called trip-link. The second distribution is a model of the trip distribution matrix, called the predicted distribution.
Generally trips should be distributed over the area proportionally to the attractiveness of activities and inversely proportional to the travel resistances between areas. It is assumed that the trips between zones will be by the most direct or cheapest routes and, taking each zone in turn, a minimum path is traced out to all other zones to form a minimum path tree. The trip distribution is a model of travel between zones-trips or links. The modeled trip distribution can then be compared to the actual distribution to see whether the model produces a reasonable approximation.
Read about: Zoning of Land for OD Survey , Traffic Volume Count , Origin Destination Survey Methods
About The Author
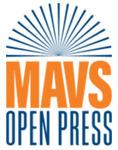
Want to create or adapt books like this? Learn more about how Pressbooks supports open publishing practices.
Part III: Travel Demand Modeling
10 First Step of Four Step Modeling (Trip Generation)
Chapter overview.
The previous chapter introduces the four-step travel demand model (FSM), provides a real-world application, and outlines the data required to carry out each of the model steps. Chapter 10 focuses on the first step of the FSM, which is trip generation. This step involves predicting the total number of trips generated by each zone in a study area and the trips attracted to each zone based on their specific purpose. The chapter delves deeper into this process, providing detailed insights into the factors influencing trip generation and how they can inform transportation planning decisions. Trip generation is a function of land use, accessibility, and socioeconomic factors, such as income, race, and vehicle ownership. This chapter also illustrates how to incorporate these inputs to estimate trips generated from and attracted to each zone using regression methods, cross-classification models (tables), and rates based on activity units as specified by the Institute of Transportation Engineers (ITE). It also provides examples to demonstrate the model applications.
The essential concepts and techniques for this step, such as growth factors and calibration methods, are also discussed in this chapter.
Learning Objectives
- Explain what trip generation is and summarize what factors contribute to trip generation.
- Recognize the data components needed for trip generation estimation and ways to prepare them for estimation.
- Summarize and compare different methods for conducting trip generation estimation and ways to interpret their results
Introduction
The Four-Step Model (FSM) is comprised of four consecutive steps, each addressing a specific question, ultimately contributing to an enhanced comprehension of travel demand. The questions are:
- Trip generation (Chapter 10) – How many total trips are estimated? What is the demand (total trips)?
- Trip distribution (Chapter 11) – Where are the trip destinations? What are the destinations of the trips?
- Modal split (Chapter 12) – What modes are used to complete those trips?
- Trip assignment (Chapter 13): What routes will be selected to complete the trips? (Meyer, 2016).
Figure 10.1 shows how the model is structured. It shows what kinds of data we provide as input for the model, and what steps we take to generate outputs.
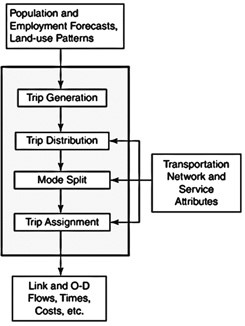
Key Concepts
Link-diverted trips: Trips produced as a result of congestion near the generator and require a diversion; new traffic will be added to the streets adjacent to the site. In other words, these are trips with multiple destinations within one area and do not require road access between destinations.
Diverted trips: Travel changes in time and route are known as diverted trips. For example, when a trip is diverted or re-routed from the original travel path due to the traffic on nearby roadways, new traffic on surrounding streets results, but the trip attraction remains the same.
Pass-by trips (see below) do not include link-diverted trips.
Pass-by trips: This type of trip is described as a trip for which the destination is not a final but a stop along the way by using the connecting roads. Passing-by traffic volume in a zone depends on the type and size of development or available activities. A gas station with higher prices near an employment center may receive many pass-by trips for gas compared to other gas stations (Where up to 50 % of all trips to a service station are travelers passing by rather than people who made a special trip to the gas station)
A gas station located in close proximity to an employment center and charging higher prices might experience a higher number of pass-by trips for gas, in contrast to other gas stations. It is observed that up to 50% of all trips to a service station are by travelers passing by, rather than individuals specifically making a deliberate trip to that gas station. (Meyer, 2016).
Traditional FSM Zonal Analysis : After inputting the required data for the model, FSM calculates the number of trips generated by or attracted to each zone using the primary input using data from travel surveys from census data. While one limitation of the trip generation model is reduced accuracy due to aggregated data, the model offers a straightforward and easily accessible set of data requirements. Typically, by utilizing basic socio-economic information like population, job figures, vehicle availability, income, and similar metrics, one can calculate trip generation and distribution.
Activity-based Analysis: There are also other (newer) methods for travel demand modeling in which individual trips are modeled based on individuals’ behaviors and activities in a disaggregated manner. The methods that use activity-based models can estimate travel demand based on a basic premise—the demand to accomplish personal activities during the day (for example, work, school, personal business, and so forth) produces a demand for travel that is often connected (Glickman et al., 2015). However, activity-based models have extensive data requirements as individuals, rather than traffic analysis zones, are the unit of analysis. Detailed information on each individual’s daily activity and socioeconomic information is needed.
Travel diaries (tours) are one source of such information (Ettema et al., 1996; Malayath & Verma, 2013). Because of travel demand modeling, additional information can be learned about the study area. For example, the detailed data may reveal information about areas with or without minimum accessibility, underserved populations, transportation inequity, or congested corridors (Park et al., 2020).
Several scholars have compared the two models – traditional zonal models and activity-based models – to assess factors such as forecasting ability, accuracy, and policy sensitivity. Despite initial expectations, the findings from some studies show no improvement in the accuracy of activity-based models over traditional models (Ferdous et al., 2011). However, considering the complexity of decision-making, activity-based models can be used to minimize the unrealistic assumptions and aggregation bias inherent in FSM models. Still, the applicability and accuracy of activity-based models should be independently assessed for each context analysis to determine which is the most effective approach.
In transportation analysis, trips are typically classified based on the origin (O)and destination (D) location. As mentioned in previous sections, for a more accurate and better estimation of trip generation results, it would be better to identify a wide range of trip categories and have disaggregate results by trip purposes. The following lists typical trip classifications:
- Home-based work (HBW) : If one of the trip origins is home and the destination is the workplace, then we can define the trip purpose as home-based work (HBW). These trips usually happen in the morning (to work) and in the evening (from work to home).
- Home-based non-work (HBNW) : If from the two ends of the trips, one is home and the other one is not workplace, the trip purpose is home-based-non-work (HBNW). Sometimes this trip purpose is called home-se is called home-based other ( HBO ). Examples of these are going to services like a restaurant or hospital.
- Non-home-based (NHB) : If neither the origin nor the destination is home, we can classify the trip as a non-home-based (NHB) purpose. One typical example is a lunch break trip from the workplace to a shopping mall.
While the above categories include only one origin and one destination, most individual trips are more complex due to chaining different trips into one tour. For instance, a person may stop for coffee or drop their child at daycare on the way to work, leave on lunch break for shopping, and then pick up their child from daycare on the way home. A tour is a continuous chain of trips an individual takes daily to complete their chores, which activity-based models can simulate (Ben-Akiva & Bowman, 1998). Figure 10.2 illustrates the different trip purposes and differences between FSM and activity-based models in trip classification.
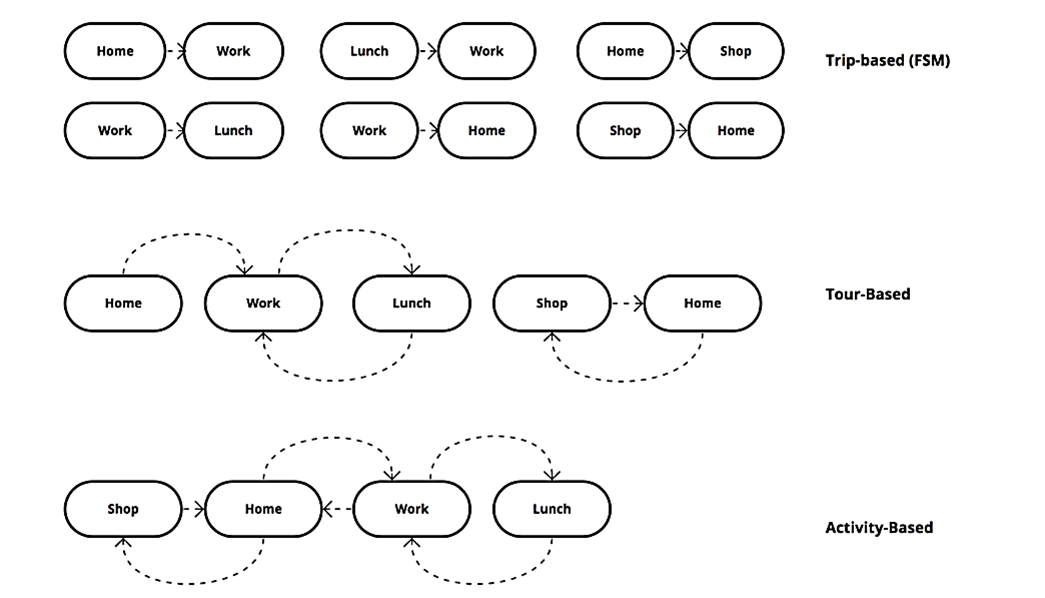
It is important to note that home-based trips can be work, school, shopping, recreational, and others. While the first two are usually mandatory and made daily, the rest are less regular or discretionary.
Trips can also be classified based on the time of day that they are generated or attracted, as traffic volumes on various corridors vary throughout the day. Essentially, the proportion of different trip purposes in the total trips is more pronounced during specific times of the day, usually categorized as peak and off-peak hours (Alkaissi, 2021).
Lastly, another factor to consider is the socio-economic characteristics and behaviors of the trip makers. An understanding of these factors is crucial for classifying trips, as some possess significant influence on travel behavior (Giuliano, 2003; Jahanshahi et al., 2009; Mauch & Taylor, 1997), such as, income level, car ownership, and household size.
Trip generation
Recall from the previous chapter, a comprehensive analysis of travel demand should include trip generation and attractions for different zones. These values should be balanced to produce an equal number of trips. In general, trip generation helps predict the number of trips for different purposes generated by and attracted to every zone in a study area.
Additionally, the number of trip ends – the total number of trips entering and leaving a specific land use or site over a designated period – can be calculated in the trip generation step (New Jersey Transit, 1994). Despite recent trends for remote work, most people do not live and work in the same area. Daily round trips to work or shopping centers originate from different locations. In this regard, the distribution of activities, like job centers, can help us to understand daily travel patterns (Wang & Hofe, 2020).
After generating an overview of the distribution of activities and land uses, we must identify the factors or conditions affectingtripgeneration. Over the years, studieshaveexaminedfactorsthatarenow accepted as standard:income,autoownership,familysize,ordensity(Ewingetal.,1996;Sharpeetal.,1958).Using a zonal level analysis, population, number of jobs, and availability of modes can affect trip generation (Wang&Hofe,2020).Similarly,thetypeandsizeofretailstores canalsoaffectthenumberoftrips.
Additionally, the predominant travel mode chosen by the population for their daily trips is a vital factor to consider. Because of the interconnectedness of land use and transportation, the primary mode influences the distribution of services, employment centers, and the overall structure and boundaries of the city. In summary, the type and intensity of land use in combination with transportation mode play crucial roles in trip generation.
The table below shows 5 hypothetical cities where the predominant mode of transportation is different for each case. According to the speed of each mode, the extent to which activities are dispersed, determines the size of the city. For instance, a city where rail is the frequent mode of transportation, the speed (21 mph) and travel time (43 mins), the catchment (distance) would be 12 miles. Using this distance as a radius, we can estimate the size of the city.
Table 10.1 Hypothetical cities with different transportation modes
According to the discussion here, the following categories can be identified as contributors to trip generation (McNally, 2007).
- Land-use types
- Land-use Intensity
- Location/accessibility
- Travel time
- Travel mode (transit, auto, walking …)
- Households’ income level
- Auto ownership rate
- Workers per household
Trip Generation Calibration
Traffic Analysis Zones (TAZs) connected by transportation networks and facilities are used to model the study area. TAZs are the smallest units of analysis in FSM. They are typically bounded by transportation networks or natural boundaries such as rivers.
Prior to estimating trip generators and attractions, calibrate the model as follows:
- Determine the regional population and the employment rate for the forecasting year to estimate the total number of interactions and possible future patterns.
- Allocate population and economic activities to each TAZ to prepare the study area for the modeling framework.
- Specify the significant variables and a proper method for creating the travel demand model (trip generation step). This step can be called model specification.
Calibration is an essential process in travel demand modeling. It involves collecting actual traffic flow data and calculating model parameters to verify the accuracy of the model for a specific region. The purpose of calibration is to match predicted outcomes with observed data, ensuring that model results are reliable and trustworthy (Wang & Hofe, 2020).
FSM MODELING UNITS
As discussed previously, the unit of analysis used for the model varies by model type. The unit of analysis is important as it guides data collection. Traditional zonal analysis, like FSM, typically uses TAZs. Activity-based models typically use data at the level of the individual person or household. There are three general methods for trip generation estimations:
1. Growth factor model,
2. regression methods,
3. cross-classification models (tables),
4. and rates based on activity units (ITE).
Generally, the trip generation step requires two types of data – household-based and zonal-based. Household-based data is more suitable for cross-classification analysis , and zonal-based data is more applicable for regression method analysis (the following sections will discuss these methods).
The third method is based on rates by which each land use type generates trips. The very general process for this method is identifying land use types, estimating trip generation according to ITE manuals, calculate total generation, and finally modifying based on specific characteristics such as proximity or location of land use. In this chapter, we do not wish to illustrate the third model, instead we focus on regression and cross-classification models since they are more data-oriented methods, more realistic and more frequently used in real-world.
The zonal analysis consists of areas divided into smaller units (zones), from which an estimate of trips generated in each zone is obtained (aggregate model). Household-based analysis decomposes zones into smaller units based on households with similar characteristics. In transportation travel demand modeling, we estimate zonal trips for various purposes, such as work, school, shopping, and social or recreational trips. As said, a zone is an area with homogeneous characteristics of land use, population, income, vehicle ownership, and the same access path outside of the zone.
In many cases, however, sufficient data at this resolution is unavailable (available at Census Tracts, Blocks, and Block Groups). In these conditions, the modeler should assess if the lower-resolution data is sufficient for their purpose. If not, using appropriate GIS-based data conversion methods, the data from a higher level (such as Census Tract) can be migrated to lower-level units (such as TAZ).
GROWTH FACTOR MODELING
A straightforward approach for estimating future trip generation volumes is to translate trends from the past into the future based on a linear growth trend of effective factors such as population or income. This method projects past data into the future by assuming a constant growth rate between two historical points. We can use this method when trip production and attraction in the base year are available, but the cost function (like travel time) is not. While this method is commonly used, it is important to note that it is insensitive to the distance between zones, which affects the estimated future data (Meyer, 2016).
In this model, the future number of trips equals the number of current trips times the growth factor.
Equation below is the method’s mathematical format:

T i is the number of trips in the zone in the forecasting year
t i is the current number of trips in that zone
f i is a growth factor
The growth factor itself consists of a number of explanatory variables that we acknowledge have impact on trip generation such as population, income (I), and ownership (V). To calculate a single growth factor with all these variables, the below equation is useful:

P i d is the population in the design year
P i c is the population in the current year
I i d is the income level in the design year
I i c is the income level in the current year
V i d is the vehicle ownership rate in the design year
V i c is the vehicle ownership rate in the current year
In a small neighborhood, 630 households reside, out of which 300 households have cars and 330 are without cars. Assuming population and income remain constant, and all households have one car in the forecasting year, calculate the total trips generated in the forecasting year and the growth factor (trip generation rate for 1-car: 2.8; 0-car:1.1). Assume that a zone has 275 households with cars and 275 without cars, and the average trip generation rates for the two groups are 5.0 and 2.5 trips per day.
Assuming all households will have a car in the future, find the growth factor and the future generated trips from that zone, keeping population and income constant.
- Current trip rate ti=300 × 2.8 + 330 × 1.1 = ? (Trips/day)
- Growth factor Fi=Vdi/Vc =630/300= ?
- Number of future trips Ti = Fiti = 2.1 × 1203 = ? (Trips / day)
Regression Analysis
Regression analysis begins with the classification of populations or zones using the socio-economic data of different groups (like low-income, middle-income, and high-income households). Trip generation can be calculated for each category and the total generated trips by each socio-economic group such as income groups and auto ownership groups using linear regression modeling. The reason for disaggregating different trip making groups is that as we discussed, travel behavior can significantly vary based on income, vehicle availability and other capabilities. Thus, in order to generate accurate trip generations using linear models such as OLS (Ordinary Least Squares) regression, we have to develop different models with different trip making rates and multipliers for different groups. This classification is also employed in cross-classification models, which is discussed next. While the initial process for regression analysis is similar to cross-classification models, one should not confuse the two methods, as the regression models attempts to fit the data to a linear model to estimate trip generation, while cross-classification disaggregates the study area based on characteristics using curves and then attributes trips to each group without building predictive models.
Alternatively, the number of total trips attracted to each zone would be determined using regression analysis on employment data and land-use attraction rates. The coefficients for the prediction model in linear regression analysis can be derived. The prediction model has a zone’s trip production or attraction as a dependent variable, and independent variables are socio-economic data aggregated by zone. Below, we illustrate a general formula for the regression type analysis:
Trip Production= f (median family income, residential density, mean number of automobiles per household)
The estimation method in this regression analysis is OLS (Ordinary Least Squares). After zonal variable data for the entire study area are collected, linear regression analysis is applied to derive the coefficients for the prediction model. A major shortcoming associated with this model is that aggregate data may not reflect the precise effect of data on trip production. For instance, individuals in two zones with an identical vehicle ownership rate may have very different access levels to private cars, thus having different trip productions. The cross-classification model described in the next section helps address this limitation (McNally, 2007).
Equation below shows the typical mathematical format of the trip generation regression model:

where X i is the independent variable and a i is the associated coefficient.
In a residential zone, trip production is assumed to be explained by the vehicle ownership rate of households. For each household type, the trip-making rates are shown in Table 10.2). Using this information, derive a fitted line. Table 10.2 documents 12 data points. Each corresponds to one family and the number of trips per day. For instance, for a 1-vehicle family, we have (1,1) (1,3), and (1,4).
Table 10.2 Sample vehicle ownership data for trip generation
The linear equation will have the form: y = bx + a. Where: y is the trip rate, and x is the household vehicle ownership, and a and b are the coefficients. For a best fit, b is given by the equation:

Based on the input table, we have:
Σx = 3 × 1 + 3 × 1 + 3 × 3 + 3 × 3 = 24 Σx2 = 3 × (1 2 ) + 3 × (2 2 ) + 3 × (3 2 ) + 3 × (4 2 ) = 90 Σy = 8 + 14 + 21 + 28 = 71 Σxy = 1 × 1 + 1 × 1 + 1 × 3 + 1 × 3 + 2 × 2 + 2 × 3 + 2 × 4 + 2 × 5 + 3 × 5 + 3 × 4 + 3 × 5 + 3 × 7 + 4 × 7 + 4 × 5 + 4 × 8 + 4 × 8 = 211
y‾ = 71/12 = 5.91 x‾ = 30/12 = 2.5 b = (nΣxy − ΣxΣy)/[(nΣx 2 − (Σx) 2 ] =((16 × 211) − (24 × 71))/((16 × 90) − (24) 2 ) = 1.93 a = y‾ − b x‾ = 17.75 – 1.93 × 2.5 = 12.925 y= 1.93X + 12.925
Cross Classification Models
This type of model estimates trip generation by classifying households into zones based on similarities in socio-economic attributes such as income level or auto ownership rate. Since the estimated values are separate for each group or category of households, this model aligns with our presumption that households with similar characteristics are likely to have similar travel patterns (Mathew & Rao, 2006). The first step in this approach is to disaggregate the data based on household characteristics and then calculate trip generations for each class. Aggregate all calculated rates together in the final step to generate total zonal trip generations. Typically, there are three to four variables for household classification, and each variable includes a few discrete categories. This model’s standard variables or attributes are income categories, auto ownership, trip rate/auto, and trip purpose.
The cross-classification method involves grouping households based on different characteristics such as income and family size. For each group, the trip generation rate can be calculated by dividing the total number of trips made by families in that group by the total number of households in that group within each zone (Aloc & Amar, 2013).
The following are some of the advantages of the cross-classification model:
- Groupings are independent of the TAZ system of the study area.
- No need to assume linearity as it disaggregates the data.
- It can be used for modal split.
- It is simple to run and understand. Furthermore, some of the model’s disadvantages are:
- It does not permit extrapolation beyond its calibration strata.
- No measure of goodness of fit is identifiable.
- It requires large sample sizes (25 households per cell); otherwise, cell values will vary.
After exploring the general definitions and features of the cross-classification model for trip generation estimations, we present a specific example and show how to perform each model step in detail.
Suppose there is a TAZ that contains 500 households, and the average income for this TAZ is
$35000. We are to develop the family of cross-classification curves and determine the number of trips produced by purpose. The low, medium, and high income are $15,000, $25,000, and $55,000, respectively (Note: this data is extracted from 1990 and is therefore out of date. Current rates for income categories may be higher.) (Adapted from: NHI, 2005). For the first step, we should develop the family of cross-class curves for the income levels and find the number of households in each income category.
If we divide the households by six income ranges, we have the table below, derived from the survey.
Based on this table, we can plot the curves in the following format:
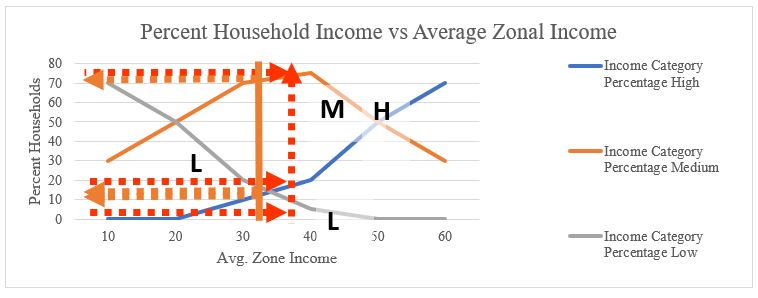
If you look at the vertical line on the $40,000 income level, you can find that the intersection of this line with three income range categories shows the percentage of households in that range. Thus, to find the number of total households in each group we have to find the intersection of the curves with average income level ($35,000). In the above plot, the orange line shows these three values, and the table below can be generated according to that:
2. In the second step, after deriving the number of households in each income category, we follow the same procedure for other variables: vehicle ownership. In other words, now we find trips per household in each auto ownership/income group “class.” Again, from the survey, we have the following table, and we can generate the plot of the curves according to that:
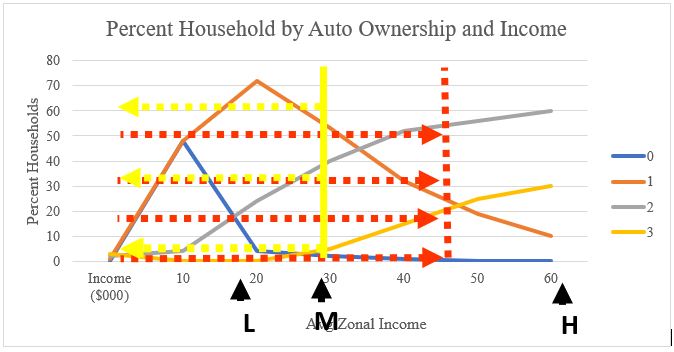
Like the previous step, the intersection of four auto ownership curves with low, medium, and high-income level lines determine the share of each auto ownership rate in each income level group:
3. After calculating the number of households in each income level category and auto ownership rate, the next step in the trip generation estimation procedure is to find the number of trips per household based on income level and auto ownership rate. The table below shows the trip generation rate for different income levels:
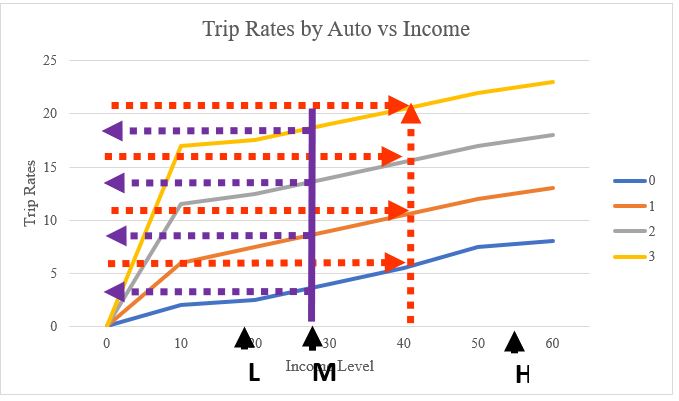
In Figure 10.3, the meeting point of three income levels and auto ownership status with trip rates yields us the following table:
4. In the fourth step, we must incorporate the trip purpose into the model. To that end, we have trip purposes ratios based on income level from the survey. Like the previous steps, we plot the table on a graph to visualize the curves and find the intersection points of the curves with our three low, medium, and high-income levels:
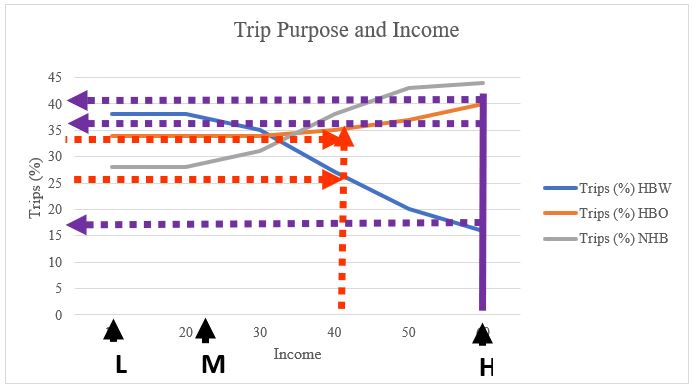
Based on the findings of this plot, we can now generate the table below, in which the percentage of trips by purpose and income level is illustrated:
Now, we have all the information we need for calculating the total number of trips by household income level and trip purpose.5.
5. In the next step, we calculate the total number of households in each income group based on the number of cars they own. Multiplying the number of households in each income group (00) to the percent of families with a certain number of cars (A) will give us the mentioned results.
6. Once we have the total number of households in each group of income based on auto ownership, we multiply the results to the trips rate (B) so that we have the total number of trips for each group.
7. In the next step, we sum the results of the number of trips by the auto ownership number to have the total number of trips for each income group (∑(00xAxB)).
8. Finally, the results from the above table (416, 3474, 1395) will be multiplied by the percentage of trip purposes for each income group in order to estimate the number of trips by trip purposes for each income group. The table below shows these results as the final trip generation results (example adapted from: NHI, 2005).
Cx∑(00xAxB):
Trip Attraction in the Cross-Classification Model
In the previous section, we modeled trips generated from different households and zones, and calculated their total number by purpose. However, in trip generation, trip attractions play a crucial role, along with trip production. To measure the attractiveness of zones, we can use an easy and straightforward method, which is to determine the size of each zone and the land use types within it, such as square feet of floor space or the number of employees. We can then derive trip generation rates for different attractions from surveys. Trip attractions refer to the number of trips that end in one zone. Typically, we express trip generation rates for different attractions in terms of the number of vehicle trips per household or unit area of non-residential land use. For instance, Table 10.13 provides trip attraction rates for residential and some non-residential land uses. The number 0.074 for HBW trips means that each household can attract 0.074 HBW vehicle trips per day. For non-residential land uses, the numbers are also dependent on the type and size of land uses. As shown in Table 10.13, the retail sector is more attractive than the basic sector.
Table 10.13 shows that the retail sector is more attractive than the basic sector.
After collecting the necessary data from surveys or other appropriate sources, a regression analysis can be used to determine the attraction rates for each land-use category. Then, the HBW vehicle trips attracted to a zone are then calculated as:

TA HBW_H = home-based work vehicle trip attractiveness of the zone by households
N hh = number of household in the zone
TAR _R = trip attraction rate by households
In a similar way, the HBW trips attracted by retail are calculated from the size of retail land use and the retail trip attraction rates.

TA HBW_NR = home-based work vehicle trip attractiveness of the zone
A _NR = non-residential land use size in the zone
TAR _NR = trip attraction rate of the non-residential land use
Assume that Table 10-14 is derived from survey data in a hypothetical city and attractiveness of each land use by trip purpose is generated.
Additionally, a new retail center in a part of the city accommodates 370 retail workers and 550 non-retail workers. According to this information, the number of trips attracted to this area can be calculated as:
First, using the information in table 10.14:
HBW: (370 * 1.7) + (550 * 1.8) = 1619
HBO: (370 * 5.4) + (550 * 2.2) = 3208
NHB: (370 * 3.0) + (550 * 1.1) = 1715
Total = 6542trips/day (example adopted from: Alkaissi, 2021)
Balancing Attractions and Productions
After generating trips, the final step is to balance trip production and attraction. Since trip generation is more accurate, and its validity is more reliable compared to trip attraction models, attraction results are usually brought to the scale of trip generation. Balance factors are used to balance Home-Based Work (HBW) trip attraction and production, which is illustrated in the example below.
According to Table 10.15, the total number of trips generated by all three zones is 600. However, the total number of trips attracted to all the zones is 800, which is an unreasonable value. To fix this issue, we use a balancing factor to multiply each cell in the attraction column by (600/800).
When planning NHB (non-home-based) trips, it is important to take an extra step to ensure that the production and attraction outputs are balanced. This means that for all zones and each zone, the total number of trips attracted and generated should be the same. The reason for this is that NHB trips have unknown origins, meaning that the origin information is not available through surveys or census data. Therefore, the most accurate estimate possible is to set the total NHB productions and attractions to be equal.
In this chapter, we introduced and reviewed the first step of travel demand modeling that is developed for estimating trip generation from each neighborhood or zone. We specifically focused on different methods (growth factor, regression, and cross-classification) and provided examples for each method along with an overview of key concepts and factors contributing to trip generation. Today, the ongoing advancements in computational capacity as well as capabilities for real-time data collection appear to be promising in equipping us with more accurate predictions of trip generation. For instance, GPS mobile data can be used to empirically estimate the rate of trip generation, build advanced models (such as machine learning models) to develop highly calibrated and optimized models.
In the next chapter, we learn about trip distribution. It is worth noting here that the trip distribution is completely based on a foundation of attractiveness of various location determined in trip generation step. As we will see, we used gravity-based models to allocate demand to pair of zones in space. In other words, four-step model is a sequential model, in which the accuracy and reliability of the each step depends on model performance in previous steps.
- activity-based model is travel forecasting framework which is based on the principle that travel is derived from demand reflected in activity patterns of individuals.
- Travel diaries (tours) refers to a chain of trips between multiple locations and for different purposes such as home to work to shopping to home.
Land-use Intensity is a measure of the amount of development on a piece of land usually quantified as dwelling per acre.
- Pass-by trips refers to the trips for which the destination is not a final destination but rather an stop along the way by using the connecting roads.
- Diverted link trips are produced from the traffic flow in the adjacent area of the trip generator that needs diversion. This new traffic will be accumulated in the roadways close to the site.
Key Takeaways
In this chapter, we covered:
- What trip generation is and what factors influence trip generation.
- Different approaches for estimating trip generation rates and the data components needed for each.
- The advantages and disadvantages of different methods and assumptions in trip generation.
- How to perform a trip generation estimation manually using input data.
Prep/quiz/assessments
- List all the factors that affect trip generation. What approaches can help incorporate these factors?
- What are the different categories of trip purposes? How do newer (activity-based models) models differ from traditional models (FSM) based on trip purposes?
- What are the data requirements for the growth factor model, and what shortcomings does this method have?
- Why should trip productions’ and attractions’ total be equal, and how do we address a mismatch?
Alkaissi, Z. (2021). Trip generation model. In Advanced Transportation Planning, Lecture, 4. Mustansiriya University https://uomustansiriyah.edu.iq/media/lectures/5/5_2021_05_17!10_34_51_PM.pdf
Aloc, D. S., & Amar, J. A. C. (2013). Trip generation modelling of Lipa City . Seminar and research methods in civil engineering research program, University of Philippines Diliman. doi: 10.13140/2.1.2171.7126.
Ben-Akiva, M.E., Bowman, J.L. (1998). Activity based travel demand model systems. In: P. Marcotte, S. Nguyen, S. (eds) Equilibrium and advanced transportation modelling. Centre for Research on Transportation . Springer, Boston, MA. Kluwer Academic Publishers, pp. 27–46. https://doi.org/10.1007/978-1-4615-5757-9_2
Ettema, D., Borgers, A., & Timmermans, H. (1996). SMASH (Simulation model of activity scheduling heuristics): Some simulations. Transportation Research Record , 1551 (1), 88–94. https://doi.org/10.1177/0361198196155100112
Ewing, R., DeAnna, M., & Li, S.-C. (1996). Land use impacts on trip generation rates. Transportation Research Record , 1518 (1), 1–6. https://doi.org/10.1177/0361198196151800101
Giuliano, G. (2003). Travel, location and race/ethnicity. Transportation Research Part A: Policy and Practice , 37 (4), 351–372. https://doi.org/10.1016/S0965-8564(02)00020-4
Glickman, I., Ishaq, R., Katoshevski-Cavari, R., & Shiftan, Y. (2015). Integrating activity-based travel-demand models with land-use and other long-term lifestyle decisions. Journal of Transport and Land Use , 8 (3), 71–93. https://doi.org/10.5198/jtlu.2015.658
ITE, I. of T. E. (2017). Trip generation manual . ITE Journal. ISSN 0162-8178. 91(10)
Jahanshahi, K., Williams, I., & Hao, X. (2009). Understanding travel behaviour and factors affecting trip rates. In European Transport Conference, Netherlands (Vol. 2009). https://www.researchgate.net/profile/Kaveh Jahanshahi/publication/281464452_Understanding_Travel_Behaviour_and_Factors_Affecting_Trip_Rates/links/57286bc808ae262228b5e362/Understanding-Travel-Behaviour-and-Factors-Affecting-Trip-Rates.pdf
Malayath, M., & Verma, A. (2013). Activity based travel demand models as a tool for evaluating sustainable transportation policies. Research in Transportation Economics , 38 (1), 45–66. https://doi.org/10.1016/j.retrec.2012.05.010
Mathew, T. V., & Rao, K. K. (2006). Introduction to transportation engineering. Civil Engineering–Transportation Engineering. IIT Bombay, NPTEL ONLINE, Http://Www. Cdeep. Iitb. Ac. in/Nptel/Civil% 20Engineering .
Mauch, M., & Taylor, B. D. (1997). Gender, race, and travel behavior: Analysis of household-serving travel and commuting in San Francisco bay area. Transportation Research Record , 1607 (1), 147–153.
McNally, M. G. (2007). The four step model. In D. A. Hensher, & K. J. Button (Eds.), Handbook of transport modelling , Volume1 (pp.35–53). Bingley, UK: Emerald Publishing. http://worldcat.org/isbn/0080435947
Meyer, M. D., (2016). Transportation planning handbook . John Wiley & Sons: Hoboken, NJ, USA, 2016.
New Jersey Transit, N. (1994). Planning for transit-friendly land use: A handbook for New Jersey communities . NJ Transit, Trenton, NJ.
NHI. (2005). Introduction to Urban Travel Demand Forecasting . In National Highway Administration (Ed.), Introduction to Urban Travel Demand Forecasting. American University. . National Highway Institute : Search for Courses (dot.gov)
Park, K., Sabouri, S., Lyons, T., Tian, G., & Ewing, R. (2020). Intrazonal or interzonal? Improving intrazonal travel forecast in a four-step travel demand model. Transportation , 47 (5), 2087–2108. https://doi.org/10.3141/1607-20
Sharpe, G. B., Hansen, W. G., & Hamner, L. B. (1958). Factors affecting trip generation of residential land-use areas . Highway Research Board Bulletin, 203 . http://onlinepubs.trb.org/Onlinepubs/hrbbulletin/203/203-002.pdf
Wang, X., & Vom Hofe, R. (2020). Selected methods of planning analysis (2nd ed. 2020 edition). Springer. Springer Nature. https://doi.org/10.1007/978-981-15-2826-2
Whitney, V. (2019, September, 29). Activity & Trip Based Travel Models. Medium . https://medium.com/data-mining-the-city/activity-trip-based-travel-models-e4833571570
Cross-classification is a method for trip production estimation that disaggregates trip rates in an extended format for different categories of trips like home-based trips or non-home-based trips and different attributes of households such as car ownership or income.
Transportation Land-Use Modeling & Policy Copyright © by Mavs Open Press. All Rights Reserved.
Share This Book
- Dictionaries home
- American English
- Collocations
- German-English
- Grammar home
- Practical English Usage
- Learn & Practise Grammar (Beta)
- Word Lists home
- My Word Lists
- Recent additions
- Resources home
- Text Checker
Definition of trip noun from the Oxford Advanced Learner's Dictionary
- Did you have a good trip?
- We went on a trip to the mountains.
- a boat/coach/bus trip
- a business/school/shopping trip
- a fishing/camping trip
- They took a trip down the river.
- We had to make several trips to bring all the equipment over.
- Jack made a return trip (= another visit to the same place) later that year.
- The return trip (= back to the place where you started) on the bike was much easier and quicker than the outbound trip.
- He went with her on her overseas trips.
- She's away on a short trip.
- He is planning a trip to Vienna.
- They organize short bike trips.
- He has just returned from a three-day trip to Australia.
- a business trip
- a five-minute trip by taxi
- a long and difficult journey across the mountains
- a tour of Bavaria
- the first expedition to the South Pole
- We went on an all-day excursion to the island.
- The children were on a day’s outing from school.
- We had a day out at the beach.
- a(n) foreign/overseas trip/journey/tour/expedition
- a bus/coach/train/rail trip/journey/tour
- to go on a(n) trip/journey/tour/expedition/excursion/outing/day out
- to set out/off on a(n) trip/journey/tour/expedition/excursion
- to make a(n) trip/journey/tour/expedition/excursion
- have/take (British English) a holiday/ (North American English) a vacation/a break/a day off/ (British English) a gap year
- go on/be on holiday/vacation/leave/honeymoon/safari/a trip/a tour/a cruise/a pilgrimage
- go backpacking/camping/hitchhiking/sightseeing
- plan a trip/a holiday/a vacation/your itinerary
- book accommodation/a hotel room/a flight/tickets
- have/make/cancel a reservation/ (especially British English) booking
- rent a villa/ (both British English) a holiday home/a holiday cottage
- (especially British English) hire/ (especially North American English) rent a car/bicycle/moped
- stay in a hotel/a bed and breakfast/a youth hostel/a villa/ (both British English) a holiday home/a caravan
- cost/charge $100 a/per night for a single/double/twin/standard/ (British English) en suite room
- check into/out of a hotel/a motel/your room
- pack/unpack your suitcase/bags
- call/order room service
- cancel/cut short a trip/holiday/vacation
- apply for/get/renew a/your passport
- take out/buy/get travel insurance
- catch/miss your plane/train/ferry/connecting flight
- fly (in)/travel in business/economy class
- make/have a brief/two-day/twelve-hour stopover/ (North American English also) layover in Hong Kong
- experience/cause/lead to delays
- check (in)/collect/get/lose (your) (especially British English) luggage/ (especially North American English) baggage
- be charged for/pay excess baggage
- board/get on/leave/get off the aircraft/plane/ship/ferry
- taxi down/leave/approach/hit/overshoot the runway
- experience/hit/encounter severe turbulence
- suffer from/recover from/get over your jet lag/travel sickness
- attract/draw/bring tourists/visitors
- encourage/promote/hurt tourism
- promote/develop ecotourism
- build/develop/visit a tourist/holiday/ (especially British English) seaside/beach/ski resort
- work for/be operated by a major hotel chain
- be served by/compete with low-cost/ (especially North American English) low-fare/budget airlines
- book something through/make a booking through/use a travel agent
- contact/check with your travel agent/tour operator
- book/be on/go on a package deal/holiday/tour
- buy/bring back (tacky/overpriced) souvenirs
- Enjoy your trip!
- He makes frequent trips to Poland.
- He's just back from a trip to Alaska.
- I had to cut short my trip when my wallet was stolen.
- My last trip abroad was two years ago.
- Don't make a special trip just to get my newspaper.
- In their last two away trips, Everton were defeated by Spurs.
- The first prize is a free trip to New York.
- The food alone made the trip worthwhile.
- The rest of our trip was uneventful.
- The trip home took us five hours!
- They are hoping to complete the trip in four days.
- They saved for years for their trip of a lifetime to Hawaii.
- Well, have a safe trip back!
- her dream trip to New Zealand
- Because of bad weather conditions, the trip was cancelled.
- Tomorrow there will be a boat trip to the island.
- We used to go on school trips to France when we were kids.
- be (away) on
- a trip abroad
- the trip home
- the trip of a lifetime
Join our community to access the latest language learning and assessment tips from Oxford University Press!
- an acid (= LSD) trip
- an act of falling or nearly falling down, because you hit your foot against something
- Don't lay a guilt trip on your child about schoolwork.
- She was trying to lay a guilt trip on me.
- Visiting my old school was a real trip down memory lane.
Other results
- round-trip ticket
- trip somebody up
- trip up | trip somebody up
- a guilt trip
- a trip/walk down memory lane
- roll/slip/trip off the tongue
- slip/roll/trip off the tongue
- a walk/trip down memory lane
Nearby words
The Definitive Glossary of Transit Terms

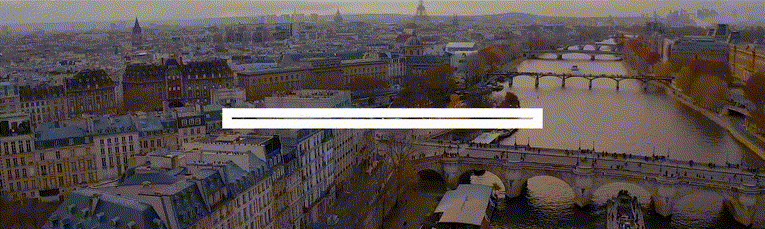
Share on Social
- Share on Facebook
- Share on Twitter
- Share on LinkedIn
- Copy URL to clipboard
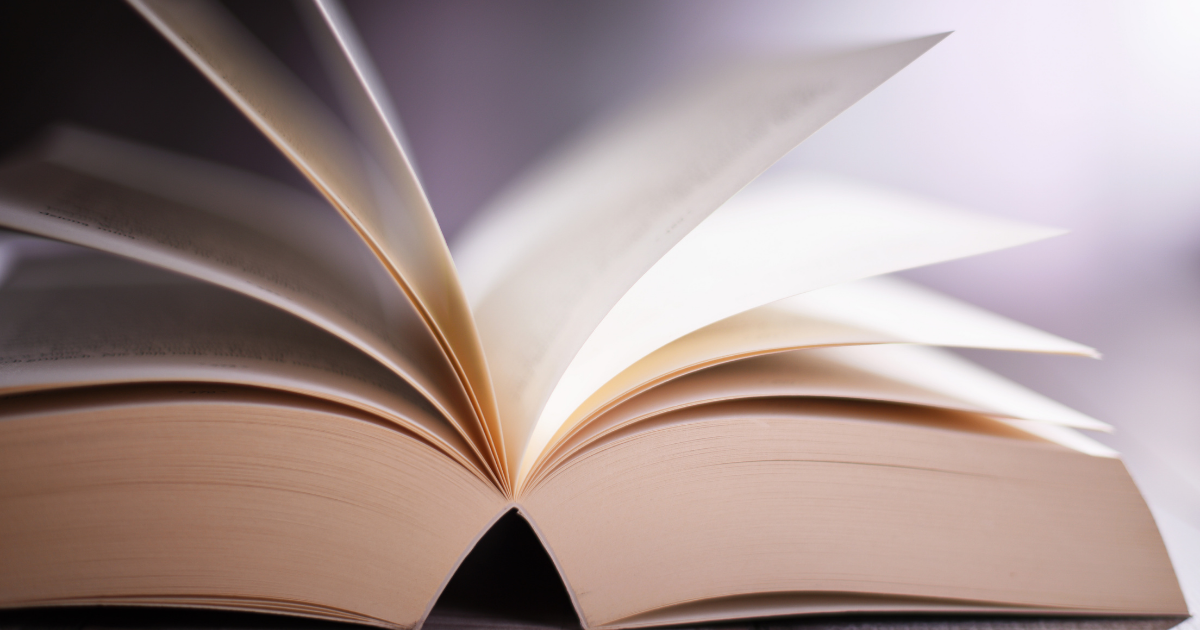
As with most industries these days, transit is evolving. Technologies are emerging, new mobility options are becoming available, and people are moving more freely than ever before. From rails to buses, to scooters and autonomous vehicles, it can be hard to keep up with the new and exciting developments happening in the world of transportation.
For those of us who work in the industry, however, knowing these terms comes with the work we love and the passion we have to keep our communities moving!
In order to make life a little easier, we’ve created a modern glossary of transit terms. In this glossary, we’ll define everything from transportation policies to new technologies, along with common acronyms and measurements that are used in transportation management every day. Click on a letter below to jump to the word you’re looking for, and let us know if we’re missing anything! We’ll try and keep this list updated as mobility continues to grow. Who knows, we might even invent a new word or two along the way.
Click on a letter below to navigate to the corresponding section.
A B C D E F G H I J K L M N O P Q R S T U V W XYZ
Accessibility
The ability and level of ease with which all riders — including those with disabilities, special requirements, or other needs — can access transportation.
ADA paratransit
The Americans with Disabilities Act (ADA) mandates that comparable transportation service be offered to individuals with disabilities who are unable to use fixed-route transportation systems. Paratransit service must be available to individuals who start and end their transportation within three-quarters of a mile of a normal fixed route.
An agency is an operator of a transit system, including both university and municipal public transportation agencies. See also: transit agency.
agency-owned on-demand microtransit
On-demand microtransit services that are either completely delivered by or through the local transit agency on behalf of their city. The agency has unfettered access to all transit service data and where the agency and the city are prescriptive on where and how services are delivered.
American Public Transportation Association (APTA)
APTA is a nonprofit international association of more than 1,500 public and private sector member organizations. Programs include advocacy for federal funding and policies, research, technical expertise and consulting services, workforce development programs, educational conferences and seminars, and 135 subject-matter working committees.
Americans with Disabilities Act (ADA)
Federal legislation passed in 1990 that defines the responsibilities of and requirements for transportation providers to make transportation accessible to individuals with disabilities. This means that public transportation providers cannot refuse to provide transportation because of a person’s disability. This also requires that a public transit agency’s fixed-route service include a complementary paratransit service for those who may be unable to access fixed-route bus or rail.
A TransLoc product that enables transit agencies to build and manage their General Transit Feed Specification (GTFS) feeds: the standard format for public transportation data. Architect allows users to simply view GTFS data in tabular and graphical formats in one place. Users can customize details for routes and stops on maps. It also has built-in checks that warn users about errors before exporting their GTFS feeds to Google, Apple, etc, so the public receives accurate GTFS files to know where their stops are located, etc.
automatic fare collection (AFC)
A system of devices and technology that automates ticketing and fare collection for a public transit system.
automatic passenger counting (APC)
Method of using electronic devices, typically situated near the doors of a bus or vehicle, that count the number of passengers that enter and exit at every stop. APC systems can sync with other operations hubs to provide real-time monitoring and inform route optimizations based on passenger information.
automatic vehicle location (AVL)
The use of computers and Global Positioning Systems (GPS) to dispatch and track transit vehicles in real-time.
automatic voice annunciation (AVA)
Equipment installed on a public transit vehicle to play audible information about upcoming stops. Used by agencies to comply with ADA requirements and provide an enhanced experience to their riders.
autonomous vehicle (AV)
A self-driving vehicle that does not require manual assistance to operate.
auto-restricted zone
Areas in which certain types of vehicles are regulated, sometimes by time of day or day of week. Public transit vehicles are usually permitted unrestricted access.
The interval between morning and evening weekday peak service periods, also known as the off-peak period.
base period
When transit services are scheduled at a normal, constant level. Also known as “off-peak periods,” when base fares are charged.
bus run number
A unique number assigned to a bus route that enables bus dispatchers to schedule buses and track their progress as they travel along their routes. Passengers can also use the run number to confirm their bus. Some transit agencies use the run number to collect data about their ridership and route patterns.
Roads, or sections of roads, that are dedicated to public buses. Busways may contain tracks or grooves for guiding buses and restricting other traffic.
An arrangement among commuters or travelers to make a regular trip in a single, shared vehicle. Some high-population areas may offer reserved carpool traffic lanes for vehicles carrying a driver and one or more passengers.
car sharing
A model of car rental where people rent cars for short periods of time, often by the hour. Attractive to people who make only occasional use of a vehicle, as well as those who would like occasional access to a vehicle of a different type than the one they use day-to-day.
central business district (CBD)
The commercial and business centers in cities. CBDs often contain the highest density of commercial space and offices and service as common destination points for public transit.
choice riders
Riders who have a transportation choice, such as commuters who could use their private vehicle but choose to take public transportation.
A fixed-route transportation option that typically operates within a closed loop, usually three miles or shorter in length. Effective in high-density areas with common shared travel patterns such as city centers or college campuses.
complete streets
An approach to transportation design that requires streets to be planned, designed, and maintained to enable safe and comfortable access for users of all ages and abilities regardless of their mode of transportation. Complete streets allow for safe travel by those walking, cycling, driving automobiles, and riding public transportation.
computer-aided dispatch (CAD)
Software helping agencies deploy their drivers and vehicles according to the complex agency rules and plans. Typical CAD systems include services for dispatching, customer support, and administration. Also referred to as a joint system in conjunction with automatic vehicle location (AVL) as CAD/AVL.
congestion mitigation and air quality (CMAQ)
A federal program that provides funding for transportation projects that reduce emissions and contributes to the reduction of pollutants. Examples of eligible projects include vehicle replacement, facility development, non-recreational trails, and bike-share programs.
contraflow lane
Reserved traffic lanes for buses where the direction of bus traffic is opposite to the flow of traffic on other lanes on the same street.
A broad area of land that follows a general directional flow, containing a number of streets, highways, and transit routes, and that connects major sources of trips.
A bus or rail route that travels across a city or town. They typically operate along a major street or avenue. Their direct route through a central business district makes crosstown buses and rails a popular transit option for riders.
curb management
Also referred to as curbside management, curb management is the collection of operations, guidelines, and practices that enable the effective management of curbs and other high-demand areas for applications such as accessibility, transportation, and safety.
curb-to-curb
Curb-to-curb service is a type of transit service where, at both the beginning and end of the trip, the driver will assist the rider between the vehicle and a sidewalk or other location no more than 15 feet from the vehicle.
When a transit vehicle is operating without passengers on board, often to and from a garage or from one route to another.
demand response
Demand response, or demand-response transit, is a broad category of public transit in which shuttles or other shared vehicles will alter their routes during each journey based on rider locations and drop offs. Rides can be summoned through an app or phone call.
demand-response transit
Demand-response transit may include shuttle services to connect riders to employment and transit centers, paratransit, and private sector transit solutions such as Uber and Lyft. Benefits of demand-responsive transit services include greater flexibility in routing and scheduling compared to traditional fixed-route services.
dependent rider
Someone who must use public transportation to meet their mobility needs.
deviated fixed route
Service routes that are characterized by deviated times, rather than deviated routes. Service routes allow riders to hail a vehicle and request a drop-off anywhere along the route.
dial-a-ride
Dial-a-ride is an origin-to-destination advanced reservation transportation service provided by public transit for seniors and persons with disabilities. Passengers must call one to three days ahead of time to request a reservation.
DIKW triangle
The hierarchy of value that data goes through while it is being transformed into wisdom. Data > Information > Knowledge > Wisdom. This is a concept that TransLoc data and product teams use as a guiding principle in product development.
Data is just numbers and labels. Giving those numbers and labels context makes it Information. Applying academic or professional experience to better understand the available information transforms Information into Knowledge. Knowing what to do with the knowledge to lead to actionable steps is Wisdom. At TransLoc, our role is to provide information in a way that enables our customers to derive knowledge, and then wisdom, from it.
downtime
A period when a bus or vehicle is not being operated due to repairs or general maintenance.
Operator of a vehicle.
electronic fare payment (EFP)
The automated calculation, validation, collection, recording, and reporting of passenger fare payments using some form of electronic media for rides on a mass transit system. Such acceptance is generally referred to as open payments within the mass transit industry.
exception date
Within GTFS, specific dates that are included or excluded from a particular trip.
fare elasticity
Fare elasticity measures how sensitive passengers are to fare price, and can be used to predict changes in service demand as fare price increases or decreases.
farebox recovery ratio
The fraction of operating expenses which are met by the fares paid by passengers. It is computed by dividing the system’s total fare revenue by its total operating expenses. Across the U.S. public transit system, the average farebox recovery ratio is approximately 20%.
farebox revenue
The value of cash, pass receipts, and tokens given by passengers as payment for transit service.
Federal Highway Administration (FHWA)
The U.S. Department of Transportation agency responsible for administering the federal highway aid program to individual states, and helping to plan, develop, and coordinate construction of federally-funded highway projects.
Federal Transit Administration (FTA)
Government agency providing financial and technical assistance to local public transit systems while also overseeing safety measures and helping develop next-generation technology research.
A GTFS “feed” is composed of a group of text files collected within a .zip file. Each file contains a particular set of transit information, such as stops, trips, routes, and other data.
first mile/last mile (FM/LM)
The beginning or end of an individual trip made primarily by public transportation. In many cases, people will walk to transit if it is close enough. However, on either end of a public transit trip, the origin or destination may be difficult or impossible to access by a short walk. This gap from public transit to destination is termed a last-mile connection.
fixed route
Transit services that are provided on a repetitive, fixed schedule along a specified route. Unlike demand responsive transit, fixed-route vehicles only stop to pick up and drop off passengers at specific locations or stops. In order to maximize efficiency, each route in a fixed-route service is usually designed to provide coverage in areas of high ridership.
fixed-route services
Services provided on a repetitive, fixed schedule along a specific route with vehicles stopping to pick up and deliver passengers to specific locations. Each fixed-route trip serves the same origins and destinations, such as rail and bus; unlike demand responsive and vanpool services.
Headway, or the time between trips, for a given route.
fringe parking
Parking areas, usually located outside of the central business district (CBD), and most often used by residents of suburban areas who commute downtown.
General Transit Feed Specification (GTFS)
Common format for public transportation schedules and geographic information. GTFS feeds allow public transit agencies to publish their transit data and developers to write applications that consume that data in an interoperable way. A GTFS feed is a collection of at least six, and up to 13 CSV files (with extension .txt) contained within a .zip file.
General Transit Feed Specification Realtime, GTFS-RT (GTFS Realtime)
A format for expressing the real-time information about a fixed-route transit system. Similar to GTFS, GTFS-RT adds a layer of dynamic information about vehicle positions and arrival times.
GTFS Validator
Provided by Google, the GTFS Validator allows you to validate a GTFS feed upon submitting it to Google.
headway
The time interval between vehicles moving in the same direction or along a particular fixed route.
headway signage
sign located in the front or rear of a bus indicating the route the bus is on.
in-advance scheduling
A paratransit feature of TransLoc’s OnDemand required for persons with disabilities to be able to schedule their rides in advance.
instant replay viewer (IRV)
A feature within many transit operations software products allowing dispatchers, administrators, and other agency personnel to view historical system operations as an “instant replay.” This feature gives agencies the opportunity to diagnose prior issues, improve driver training, and audit service performance after-the-fact.
intelligent transport systems (ITS)
An advanced application which aims to provide innovative services relating to different modes of transport and traffic management, enabling users to be better informed and make safer and smarter use of transport networks. ITS is made up of 16 types of technology-based systems, divided into intelligent infrastructure systems and intelligent vehicle systems.
intermodal
Intermodal, or multimodal, services include more than one mode of transportation and often require connections, choices, and coordination between various modes.
intermodal passenger transport
See multimodal transportation .
internal destination signage
Displays and plays stop arrivals and departures. Also known as interior signage.
International Parking & Mobility Institute (IPMI)
I PMI is the world’s largest association of professionals in parking, transportation, and mobility. Members include everyone from garage owners and operators to architects to city managers to government agencies, health care centers, universities, airports, and convention centers.
jitney
A small bus or other vehicle that carries passengers for a low fare. In the early 1900s, jitney was slang for nickel due to the popularity of services that charged five-cent fares.
kiosk signage
Any sign at a terminal or stop that has human interaction.
layover time
Time that is built into a transit schedule between the end of a route and departure for the return trip. Layover time is typically used to recover any delays accumulated during the initial trip and to prepare for the return trip.
load factor
A ratio calculated by the number of passengers carried divided by the total passenger capacity of a transit vehicle.
lobby signage
Digital display sign located at an internal stop or lobby.
mean distance between failures (MDBF)
A measurement of the average distance in miles that a transit vehicle travels before a breakdown or other failure causes the vehicle to be removed from service.
micromobility
The use of small, lightweight personal vehicles (e.g., electric scooters, e-bikes, bicycles, etc.) to travel short distances, typically within an urban environment or college campus.
microtransit
A flexible, demand-driven mode of service that helps agencies optimize vehicles and improve the rider experience while reducing cost. See also: on-demand microtransit.
mixed-mode commuting
mobile ticketing
Providing fares for riders through mobile devices. Companies such as Masabi and Token Transit.
Mobility-as-a-Service (MaaS)
A shift away from personally-owned modes of transportation and towards mobility solutions that are consumed as a service. This is enabled by combining transportation services from public and private transportation providers through a unified gateway that creates and manages the trip, which users can pay for with a single account. Users can pay per trip or a monthly fee for a limited distance. The key concept behind MaaS is to offer travelers mobility solutions based on their travel needs.
mobility integrator (MI)
What transit agencies are aiming to become by integrating not only different modes of transportation, but also regional, city, and county partners to provide real-time solutions for riders.
mobility management
The application of strategies and policies to reduce travel demand, or to redistribute this demand. Managing demand can be a cost-effective alternative to increasing capacity.
mobility on demand (MOD)
An innovative, user-focused approach which leverages emerging mobility services, integrated transit networks and operations, real-time data, connected travelers, and cooperative ITS to allow for a more traveler-centric approach, providing improved mobility options to all travelers and users of the system in an efficient and safe manner.
mobility service provider (MSP)
An organization that pairs passengers via websites and mobile apps with drivers who provide transportation services. Transportation network companies are examples of the sharing economy and shared mobility. See also: transportation network companies (TNCs)
modal split
The number of people who use alternative forms of transportation in lieu of public transit. It is typically used to determine the percentage of people in an area who use private vehicles as an alternative to public transit.
multimodal transportation
Involves using two or more modes of transportation in a journey. Mixed-mode commuting is often used to combine the strengths and offset the weaknesses of various transportation options. A major goal of modern intermodal passenger transport is to reduce dependence on the automobile as the major mode of ground transportation and increase use of public transport.
National Transportation Database (NTD)
The NTD was established by Congress to be the nation’s primary source for information and statistics on the transit systems of the United States. Recipients or beneficiaries of grants from the FTA are required by statute to submit data to the NTD.
non-emergency medical transport/transportation (NEMT)
A transportation service provided to individuals who are not in an emergency situation but need more assistance than a taxi service is able to provide. Oftentimes, these services are specially equipped to transport riders in wheelchairs, stretchers, or with other ability needs.
on-board communication (OBC)
On-board communication systems can consist of audio and/or visual communication devices such as loudspeakers, LED signs, and video monitors that allow for messages to be relayed to passengers in order to communicate route and stop information or other pertinent messages while onboard a bus or vehicle.
on-board survey
Surveys conducted by transit agencies every three to ten years. On-board surveys generally involve hiring an outside company to create a survey and collect responses from passengers on agency vehicles. Surveys try to assess passenger satisfaction and gather information for planning purposes as well as to satisfy funding sources such as state or federal grants.
Software provided by TransLoc that helps transit providers manage their demand-response operations, including microtransit, campus/university safe ride, and dial-a-ride services.
on-demand transit
A method of passenger transportation that allows for vehicles to alter their routes during each journey based on particular transport demand without using a fixed route or timetable. Vehicles typically pick-up and drop-off passengers in locations according to passengers’ needs and can include taxis, buses, or other vehicles. See also: demand response
operating budget
Detailed projection of all estimated income and expenses based on forecasted sales revenue during a given period. A majority of municipal transit agencies operate on a fiscal year operating budget that runs from July 1st to June 30th.
paddle
The schedule of work for each driver showing all routes they will operate in a day, including arrival and departure times and specific directions.
paratransit
Paratransit is recognized in North America as special transportation services for people with disabilities, often provided as a supplement to fixed-route bus and rail systems by public transit agencies. TransLoc OnDemand is a demand-response system that can power paratransit systems.
An individual on board, boarding, or alighting from a revenue transit vehicle. Excludes operators, transit employees, and contractors.
passenger fares
Revenue earned from carrying passengers in regularly scheduled services.
passenger information system (PIS)
An automated information system providing real-time passenger information. It may include predictions about arrival and departure times, as well as information about the nature and causes of disruptions. It may be used both physically within a transportation hub and remotely using a web browser or mobile device.
passenger miles
The total number of miles traveled by passengers on transit vehicles. Passenger miles are determined by multiplying the number of unlinked passenger trips times the average length of their trips.
Not defined within the actual GTFS specification, a pattern is an abstraction that exists only within TransLoc Architect. A pattern defines a template for a trip, but does not include stop times or calendar information. Within Architect, it is possible to update a pattern and have the change propagate to the associated trips.
peak/base ratio
Calculated by taking the number of vehicles operated in passenger service during the peak period divided by the number of vehicles operated during the base period.
peak period
Time periods when transit riding is busiest. Typically peak periods are in the morning and afternoon, coinciding with business commutes and standard highway rush hour.
A router that brings in a cell network data plan and creates WIFI and GPS from the device.
Planning & Design Services
A solution that uses the expertise of TransLoc’s certified transportation planners to design the optimal transit system for customers that flexes between fixed route and on demand for their unique system’s needs. This is done through simulation algorithms, service cost analysis, route/system redesign, comprehensive service analysis, and data and visualization analysis.
privately-owned
Ride-sharing, shuttle, or other transportation services that are owned and operated by private companies, not affiliated with local or regional governments. Privately-owned transportation services are often reliant on smaller, private vehicles and are typically predominant in high-traffic areas only, with no obligation to serve under-represented populations.
rapid transit
A rail or bus transit service that operates completely separate from all other modes of transportation on an exclusive right-of-way route.
TransLoc dispatching and fleet management software, which uses integrations with on-vehicle hardware to enable transit agencies to access map-based vehicle tracking and route and vehicle performance.
real-time tracking
The ability to track an agency’s current vehicle location as it navigates a fixed or ad hoc route.
recovery time
A planned time allowance between the arrival time of a just completed trip and the departure time of the next trip in order to allow the route to return to schedule if traffic, loading, or other conditions have made the trip arrive late. Recovery time is considered as reserve running time. Typically, the operator will remain on duty during the recovery period.
refresh rate
The update rate of a GPS module is how often it calculates and reports its position. The standard for most devices is 1hz (once per second).
revenue service
The time when a vehicle is available to the general public and there is an expectation of carrying passengers. These passengers either directly pay fares, are subsidized by public programs, or provide payment through some contractual arrangement. Vehicles operated in fare free service are considered in revenue service. Revenue service includes layover and recovery time. Revenue service excludes deadhead, vehicle maintenance testing, school bus service, and charter service.
reverse commuting
Travel that occurs opposite from the main flow of traffic during the morning peak period. Reverse commuting typically occurs from a CBD to a suburb or non-central location.
ride-hailing service
Booking rides and paying for a car service with a transportation network company (TNC) through an app. Ridesharing is also used, but this term has been considered inaccurate, so ride-hailing has been considered a more accurate descriptor.
A transit user. See also: passenger.
ridership
The total number of passenger trips taken on a particular service or system during a given time period.
ridesharing
A form of transportation, other than public transit, in which more than one person shares in the use of the vehicle, such as a van or car, to make a trip. Uber, Lyft, Via and others are examples of ridesharing services.
rolling stock
The vehicles used in a transit system, including buses, rail cars, vans, and shuttles.
The literal transit route, often referred to as a public transit “line.” Within GTFS, a route is made up of one or more “trips,” which occur at a specific time, making a route time independent.
route miles
The total number of miles along a fixed-route transit system.
routing waypoint
Within the pattern editor for Architect, a routing waypoint allows you to draw a shape that is automatically routed along roads on the map.
A university-funded service designed as a safe means of transportation on university campuses after dark.
seamless mobility
Providing riders a way to complete their journeys across all mobility modes easily while giving agencies the ability to offer demand-driven modes to increase access to public transportation and make transit the first choice for all.
service alert
An announcement by a transit agency to a rider regarding an unexpected service interruption (e.g., delays, cancellations). A service alert can be sent to a rider’s mobile device, shared on a transit agency’s website, and/or announced over a vehicle intercom system.
Describes the physical path that a vehicle takes in the real world. A shape consists of a sequence of points, with the points traced in the appropriate order, providing the path of the vehicle.
simulation
Virtual simulations are commonly used by transit providers to predict the performance and viability of a particular transit service given a set of circumstances. Simulations are commonly used when implementing demand responsive or microtransit services alongside fixed route or other service models in order to create optimal coverage within multimodal service areas.
single-occupancy vehicle (SOV)
A single-occupancy, privately-operated vehicle with the driver as the only occupant. The drivers of SOVs use their vehicles primarily for personal travel, daily commuting, and for running errands.
A smart city is an urban area that uses different types of electronic data collection sensors to supply information which is used to manage assets and resources efficiently.
sprawl
T he expansion of human populations away from central urban areas into low-density, monofunctional, and car-dependent communities.
A location where a vehicle allows passengers to board or alight.
Times that a vehicle arrives and departs from an individual stop for a given trip.
third-party operator
Contract organizations that operate part or all of a university or municipal transit system on behalf of the university or municipality. Companies like FirstTransit can deploy an entire transit operation for their customers including vehicles, drivers, administrators, planners, etc.
Refers to Title VI of the Federal Civil Rights Act of 1964, and requires that transportation planning and programming be nondiscriminatory on the basis of race, color, and national origin.
traffic signal priority (TSP)
The operational process that prioritizes transit vehicles at intersections that contain traffic signals. There are a variety of implementation options for signal priority, but all work by holding green lights longer, and red lights shorter, for transit vehicles to reduce travel times and maintain schedule adherence.
transit agency/provider
An organization that has specific authority to operate public transit within a specified geographic area.
transit authority/transit district
A transit authority or transit district has the power of the government for solving problems related to transit issues. This includes eminent domain, the ability to impose taxes, and the ability to operate independently of the cities and counties that the transit district operates within.
transit desert
Areas containing transit-dependent populations who lack access to adequate public transit service.
transit-oriented development (TOD)
A type of development that links land use and transit facilities to support the transit system and help reduce sprawl, traffic congestion, and air pollution. It includes housing and complementary public uses (e.g., jobs, retail, and services) located at a strategic point along a regional transit system, such as a rail hub or major transit stop.
TransLoc app
The only transit app with both fixed-route and on-demand data, giving riders more choice and more service reach for transit providers. TransLoc app is available in Spanish and simplified Chinese, with more languages on the way. With a focus on accessibility, the app is fully aligned with Web Content Accessibility Guidelines (WCAG) 2.0 AA standards.
transportation management area (TMA)
Designated by the United States Secretary of Transportation as urbanized areas with a population of at least 200,000 people. These designations require additional oversight and gain access to planning benefits in an effort to continually improve planning processes in areas with large populations.
transportation network company (TNC)
An organization that pairs passengers with drivers who provide transportation services. Transportation network companies are examples of the sharing economy and shared mobility. Uber and Lyft are TNCs.
travel demand model
Used by transportation planners for simulating current travel conditions and for forecasting future travel patterns and conditions. Travel demand models help planners and policymakers analyze the effectiveness and efficiency of alternative transportation investments in terms of mobility, accessibility, and environmental and equity impacts.
travel time
The movement of people between relatively distant geographical locations and can involve travel by foot, bicycle, automobile, train, bus, or other means. It can be one way or round trip.
A time-specific journey taken by a vehicle through a series of stops as expressed within a GTFS feed.
United States Department of Transportation (USDOT)
The federal cabinet-level agency with responsibility for highways, mass transit, aviation, and ports. It is headed by the Secretary of Transportation. The USDOT includes the Federal Highway Administration and the Federal Transit Administration, among others.
unlinked passenger trips
The frequency in which passengers board a public transportation vehicle.
urbanized area
The fully developed area of a central city and its suburbs. A rather complicated, but consistent formula measures for contiguous urban development. According to the 2010 census, urban areas — classified as either larger urbanized areas or smaller urban clusters — must encompass at least 2,500 people with 1,500 residing outside institutional group quarters.
Agency personnel that use our software, not passengers that use the app.
vehicle miles traveled (VMT)
One vehicle traveling one mile constitutes a vehicle mile. VMT is one measure of the use of state highways and roads, and is aggregated by calculating the total annual miles of vehicle travel divided by a geographical location’s total population.
vehicle operators
Bus drivers, shuttle drivers, train conductors or any operator of a vehicle in a transportation system.
voice announcements
The Americans with Disabilities Act (ADA) requires transit agencies to announce stops at transfer points, major intersections, destination points, requested stops, and at intervals along a route sufficient to permit individuals who are blind or have vision impairments to be oriented to their location.
wait time
Wait time refers to the time spent by passengers while waiting for a transit vehicle.
wayside signage
Also called outdoor displays. Signage located at a stop or terminal for riders to know how much longer until the bus arrives.
Web Content Accessibility Guidelines (WCAG)
A range of recommendations to make Web content accessible to people with cognitive disabilities, visual, auditory, and speech impairments, or limited mobility. WCAG 2.0 , published on December 11, 2008, consists of 12 guidelines categorized by four principles. All TransLoc software is designed with these guidelines and principles in mind.
zone fares
A system of fares where a transit system’s service area is divided into zones with different specified rates or fares.

Page categories
Needs Review
Trip distribution
The trip distribution step (within trip-based models) determines where trips go, spatially. Trip distribution allocates trips to pairs of traffic analysis zones (TAZs), from the production (P) end to the attraction (A) end. The result is a PA table, which describes the numbers of person trips between zones (usually over a 24-hour period of time) but does not describe the actual direction of travel. Inputs to the trip distribution step come principally from the trip generation step. There is also a requirement for calibrated parameters. The number of these parameters depends upon the model formulation.
There are a variety of trip distribution formulations. Among recent travel models two formulations dominate: the gravity “model” and the destination choice “model”. There are variations to each formulation.
The gravity “model” allocates trips roughly in proportion to the number of productions at the production end, roughly in proportion to the number of attractions at the attraction end, and roughly in proportion to a measure of proximity (often called a “friction factor”) of the two zones. A gravity “model” may be “singly-constrained” or “doubly-constrained”. A singly-constrained gravity “model” assures that the PA table is consistent with trip productions from trip generation. A doubly-constrained gravity “model” also assures that the PA table is consistent with trip attractions from trip generation.
A friction factor is a function of trip impedance (time, cost, distance, etc.) between zones, with impedance usually expressed in units of travel time. There is an inverse relation between the value of a friction factor and the value of impedance. This inverse relation is largely empirical in practice, although theories such as “entropy maximization” have been proposed to derive this relation. A functional form dubbed the “gamma” function has been recommended by NCHRP Report 365 and NCHRP Report 716; however, many travel models use a negative exponential function or a power function with a negative power. Some travel models use a table lookup to obtain friction factors.
A technical discussion of the gravity model, along with example friction factors, may be found in NCHRP Report 716. An alternative to a gravity “model” is a destination choice “model”. The preferred destination choice “model” takes the form of a multinomial logit equation. The utility function for the destination choice “model” may contain measures of impedance (time, cost, distance, etc.) as well as a measure of attraction zone size and perhaps socioeconomic variables. A singly-constraint gravity "model" containing a negative exponential friction factor function is very similar to a destination choice "model".
Trip distribution for multimodal forecasts needs a combined impedance across all modes that reflect the various mode shares. A feedback from mode split to trip distribution is not usually accomplished. Rather, multimodal trip distribution uses a “combined impedance” for all modes which is calculated as a “log sum”, similar to what is used in a nested logit formulation. See NCHRP Report 716 for a discussion about “log sum”.
← Trip Generation Destination choice models →
This site uses cookies to learn which topics interest our readers.
A traffic analysis zone definition: a new methodology and algorithm
- Published: 07 May 2009
- Volume 36 , pages 581–599, ( 2009 )
Cite this article
- Luis M. Martínez 1 ,
- José Manuel Viegas 1 &
- Elisabete A. Silva 2
2301 Accesses
70 Citations
Explore all metrics
This paper develops a comprehensive approach to the definition of transportation analysis zones (TAZ), and therein, presents a new methodology and algorithm for the definition of TAZ embedded in geographic information systems software, improves the base algorithm with several local algorithms, and comprehensively analyses the obtained results. The results obtained are then compared to these presently used in the transportation analysis process of the Lisbon Metropolitan Area. The proposed algorithm presents a new methodology for TAZ design based on a smoothed density surface of geocoded travel demand data. The algorithm aims to minimise the loss of information when moving from a continuous representation of the origin and destination of each trip to their discrete representations through zones, and focuses on the trade-off between the statistical precision, geographical error, and the percentage of intra-zonal trips of the resulting OD matrix. The results for the Lisbon Metropolitan Area case study suggest a significant improvement in OD matrix estimates compared to current transportation analysis practises based on administrative units.
This is a preview of subscription content, log in via an institution to check access.
Access this article
Price includes VAT (Russian Federation)
Instant access to the full article PDF.
Rent this article via DeepDyve
Institutional subscriptions
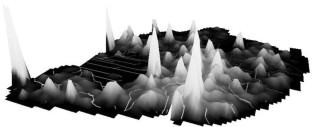
Similar content being viewed by others
Adaptive Zoning for Efficient Transport Modelling in Urban Models
The approaches to measuring the potential spatial access to urban health services revisited: distance types and aggregation-error issues.
The Impact of Data Aggregation on Potential Accessibility Values
Developed in Geomedia Professional 5.1 (INTERGRAPH).
The thin plate spline is the two-dimensional analogue of the cubic spline in one dimension. It is the fundamental solution to the biharmonic equation. The name "thin plate spline" refers to a physical analogy involving the bending of a thin sheet of metal.
This value was obtained for the case study after calibration using different size grid cells.
The relative statistical error is presented in Eq. 2.
The surrounding area size is a parameter of the algorithm that is defined by the user.
The concept of influence area of local “highest peaks” is defined as the minimum size that an analyst establishes for the specific modelling problem.
The core problem area parameter allows the analyst to establish different levels of relevance inside the modelling area, which does not need to be used. The default is the entire modelling area.
Subset containing zones with a statistical average equal to or less than 50%.
Amrhein, C.G.: Searching for the elusive aggregation effect—evidence from statistical simulations. Environ. Plan. A 27 (1), 105–119 (1995)
Article Google Scholar
Baass, K.G.: Design of zonal systems for aggregate transportation planning models. Transp. Res. Rec.—Travel. Demand. Forecast. Data. Consid. 807 , 1–6 (1981)
Google Scholar
Batty, M.: Spatial entropy. Geogr. Anal. 6 (1), 1–31 (1974)
Batty, M.: Entropy in spatial aggregation. Geogr. Anal. 8 (1), 1–21 (1976)
Batty, M., Sikdar, P.K.: Spatial aggregation in gravity models.1. An information-theoretic framework. Environ. Plan. A 14 (3), 377–405 (1982a)
Batty, M., Sikdar, P.K.: Spatial aggregation in gravity models.2 One-dimensional population-density models. Environ. Plan. A 14 (4), 525–553 (1982b)
Batty, M., Sikdar, P.K.: Spatial aggregation in gravity models.3. Two-dimensional trip distribution and location models. Environ. Plan. A 14 (5), 629–658 (1982c)
Batty, M., Sikdar, P.K.: Spatial aggregation in gravity models.4. Generalizations and large-scale applications. Environ. Plan. A 14 (6), 795–822 (1982d)
Bennion, M.W., O’Neill, W.A.: Building transportation analysis zones using geographical information systems. Trasp. Res. Rec.—Multimodal. Prior. Setting. Appl. Geogr. Inf. Syst. 1429 , 49–56 (1994)
Binetti, M., Ciani, E.: Effects of traffic analysis zones design on transportation models. In: Proceedings of the 9th Meeting of the Euro Working Group on Transportation, Bari, Italy (2002)
Chang, K.T., Khatib, Z., Ou, Y.M.: Effects of zoning structure and network detail on traffic demand modeling. Environ. Plan. B-Plan. Des. 29 (1), 37–52 (2002)
Chapleau, R.: Conducting telephone origin—destination household surveys with an integrated informational approach. In: Proceedings of the International Conference on Transport Survey Quality and Innovation, Grainau, Transportation Research Board (1997)
Chesbon, G., Cheryl, P.: Using GIS to define and develop TAZ data. In: Proceedings of the 4th International Conference on Microcomputers in Transportation, Baltimore, Maryland (1992)
Clark, W.A.V., Avery, K.L.: Effects of data aggregation in statistical-analysis. Geogr. Anal. 8 (4), 428–438 (1976)
Crevo, C.C.: Impacts of zonal reconfigurations on travel demand forecasts. Transp. Res. Rec. 1477 , 72–80 (1991)
Ding, C.: Impact analysis of spatial data aggregation on transportation forecasted demand. In: Proceedings of the Urban and Regional Information System Association (URISA) Conference, Washington DC, URISA (1994)
Ding, C.: The GIS-based human-interactive TAZ design algorithm: examining the impacts of data aggregation on transportation-planning analysis. Environ. Plan. B-Plan. Des. 25 (4), 601–616 (1998)
Ding, C., Choi, K., Kim, T.J.: GIS-based traffic analysis zone design. In: Proceedings of the 3rd International Conference on Computers in Urban Planning and Urban Management, Atlanta (1993)
Eagleson, S., Escobar, F., Williamson, I.: Hierarchical spatial reasoning theory and GIS technology applied to the automated delineation of administrative boundaries. Comput. Environ. Urban. Syst. 26 (2–3), 185 (2002)
Edwards, J.D.: Transportation planning handbook, 2nd edn. Institute of Transportation Engineers, Washington DC (1992)
Fotheringham, A.S., Rogerson, P.A.: GIS and spatial analytical problems. Int. J. Geogr. Inf. Syst. 7 (1), 3–19 (1993)
Fotheringham, A.S., Wong, D.W.S.: The modifiable areal unit problem in multivariate statistical-analysis. Environ. Plan. A 23 (7), 1025–1044 (1991)
Gan, C.-T.: A GIS-aided procedure for converting census data for transportation planning. ITE J.-Inst. Transp. Eng. 64 (11), 34–40 (1994)
Goodchild, M.F.: Aggregation problem in location-allocation. Geogr. Anal. 11 (3), 240–255 (1979)
Keane, M.: Size of region-building problem. Environ. Plan. A 7 (5), 575–577 (1975)
Martínez, L.: “TAZ delineation and information loss in transportation planning studies”. MS Dissertation submitted in Department of Civil and Architecture, IST-Technical University of Lisbon, Lisbon, Portugal (2006)
Masser, I., Brown, P.J.B.: Hierarchical aggregation procedures for interaction data. Environ. Plan. A 7 (5), 509–523 (1975)
Morphet, C.S.: A statistical method for the identification of spatial clusters. Environ. Plan. A 29 (6), 1039–1055 (1997)
O’Neill, W.A.: Developing optimal transportation analysis zones using GIS. ITE J.-Inst. Transp. Eng. 61 (12), 33–36 (1991)
Openshaw, S.: Optimal zoning systems for spatial interaction models. Environ. Plan. A 9 (2), 169–184 (1977)
Openshaw, S., Rao, L.: Algorithms for reengineering 1991 census geography. Environ. Plan. A 27 (3), 425–446 (1995)
Openshaw, S., Taylor, P.J.: A million or so correlation coefficients: three experiments on the modifiable areal unit problem. In: Wringley, N. (ed.) Statistical applications in the spatial sciences, pp. 127–144. Pion, London (1979)
Ortúzar, J., Willumsen, L.: Modeling transport, 3rd edn. Wiley, New York (2001)
Robinson, W.S.: Ecological correlations and the behavior of individuals. Am. Sociol. Rev. 5 (3), 351–357 (1950)
Scott, A.J.: Combinatorial programming spatial analysis and planning. Methuen & Co., Ltd., London (1971)
Trepanier, M., Chapleau, R.: Object-oriented and totally disaggregate analysis of origin-destination household survey data. Can. J. Civ. Eng. 28 (1), 48–58 (2001)
Viegas, J., Martínez, L., Silva, E.: Modifiable areal unit problem effects on traffic analysis zones delineation. Environ. Plan. B (2008). doi: 10.1068/b34033
Ward, J.H.: Hierarchical grouping to optimize an objective function. J. Am. Stat. Assoc. 58 , 236–244 (1963)
Wong, D., Amrhein, C.: Research on the MAUP: old wine in a new bottle or real breakthrough. Geogr. Syst. 3 , 73–76 (1996)
Xie, Y.: The overlaid network algorithms for areal interpolation problem. Comput. Environ. Urban. Syst. 19 (4), 287 (1995)
You, J.S., Nedovic, Z., Kim, T.J.: A GIS-based traffic analysis zone design: implementation and evaluation. Transp. Plan. Technol. 21 (1–2), 69–91 (1997a)
You, J.S., Nedovic, Z., Kim, T.J.: A GIS-based traffic analysis zone design: technique. Transp. Plan. Technol. 21 (1–2), 45–68 (1997b)
Download references
Acknowledgments
This research has been supported by the Portuguese National Science Foundation (FCT) since 2004. We thank the consultancy TIS.pt for providing support by making the LMA Mobility Survey (TIS, 1994) available and the software company INTERGRAPH for the Geomedia Professional 5.1 license.
Author information
Authors and affiliations.
CESUR, Department of Civil Engineering, Instituto Superior Técnico, Lisbon Technical University, Av. Rovisco Pais, 1049-001, Lisbon, Portugal
Luis M. Martínez & José Manuel Viegas
Department of Land Economy, University of Cambridge, 19 Silver Street, Cambridge, CB3 9EP, UK
Elisabete A. Silva
You can also search for this author in PubMed Google Scholar
Corresponding author
Correspondence to Luis M. Martínez .
Rights and permissions
Reprints and permissions
About this article
Martínez, L.M., Viegas, J.M. & Silva, E.A. A traffic analysis zone definition: a new methodology and algorithm. Transportation 36 , 581–599 (2009). https://doi.org/10.1007/s11116-009-9214-z
Download citation
Published : 07 May 2009
Issue Date : September 2009
DOI : https://doi.org/10.1007/s11116-009-9214-z
Share this article
Anyone you share the following link with will be able to read this content:
Sorry, a shareable link is not currently available for this article.
Provided by the Springer Nature SharedIt content-sharing initiative
- TAZ (transportation analysis zones)
- Transportation planning studies
- Transportation demand models
- GIS (geographic information systems)
- Find a journal
- Publish with us
- Track your research
- More from M-W
- To save this word, you'll need to log in. Log In
Definition of trip
(Entry 1 of 2)
intransitive verb
transitive verb
Definition of trip (Entry 2 of 2)
- peregrination
Examples of trip in a Sentence
These examples are programmatically compiled from various online sources to illustrate current usage of the word 'trip.' Any opinions expressed in the examples do not represent those of Merriam-Webster or its editors. Send us feedback about these examples.
Word History
Middle English trippen , from Anglo-French treper, triper , of Germanic origin; akin to Old English treppan to tread — more at trap
14th century, in the meaning defined at intransitive sense 3a
14th century, in the meaning defined at sense 5
Phrases Containing trip
- business trip
- lay / put a guilt trip on
- on a power trip
- roll / trip off the tongue
- round - trip ticket
- trip - hammer
- trip the light fantastic
Dictionary Entries Near trip
trioxymethylene
Cite this Entry
“Trip.” Merriam-Webster.com Dictionary , Merriam-Webster, https://www.merriam-webster.com/dictionary/trip. Accessed 29 Apr. 2024.
Kids Definition
Kids definition of trip.
Kids Definition of trip (Entry 2 of 2)
More from Merriam-Webster on trip
Nglish: Translation of trip for Spanish Speakers
Britannica English: Translation of trip for Arabic Speakers
Subscribe to America's largest dictionary and get thousands more definitions and advanced search—ad free!
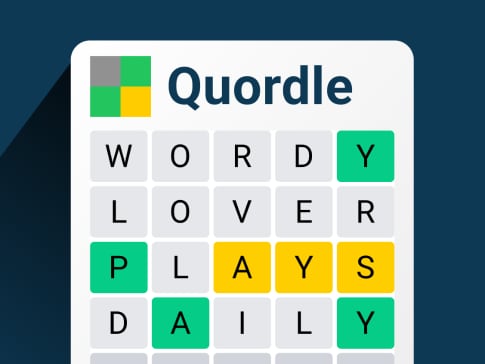
Can you solve 4 words at once?
Word of the day.
See Definitions and Examples »
Get Word of the Day daily email!
Popular in Grammar & Usage
More commonly misspelled words, commonly misspelled words, how to use em dashes (—), en dashes (–) , and hyphens (-), absent letters that are heard anyway, how to use accents and diacritical marks, popular in wordplay, the words of the week - apr. 26, 9 superb owl words, 'gaslighting,' 'woke,' 'democracy,' and other top lookups, fan favorites: your most liked words of the day 2023, 10 words for lesser-known games and sports, games & quizzes.
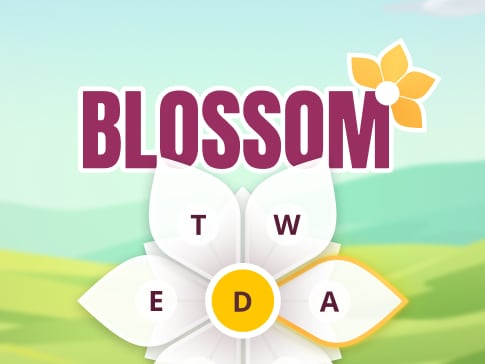
An official website of the United States government Here's how you know
Official websites use .gov A .gov website belongs to an official government organization in the United States.
Secure .gov websites use HTTPS A lock ( Lock A locked padlock ) or https:// means you’ve safely connected to the .gov website. Share sensitive information only on official, secure websites.
National Transit Database (NTD) Glossary
A b c d e f g h i j k l m n o p q r s t u v w x y z .
Section 5311(j) of the FAST Act, Public Law 114-94 (December 4, 2015), authorizes the Public Transportation on Indian Reservations Program (Tribal Transit Program (TTP)) for Fiscal Years (FY) 2016-2020. The TTP continues to be a set-aside from the Formula Grants for Rural Areas program but now consists of a $30 million formula program and a $5 million competitive grant program subject to the availability of appropriations.
A collision, derailment, fire, hazardous material spill, act of nature (Act of God), evacuation, or Other Safety Occurrence not Otherwise Classified (OSONOC) occurring on transit right-of-way, in a transit revenue facility, in a transit maintenance facility, or involving a transit revenue vehicle and meeting established NTD thresholds. Can be found in: S&S Introduction, S&S-40, S&S-50
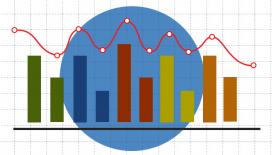
- ABC7 New York 24/7 Eyewitness News Stream Watch Now
- THE LOOP | NYC Weather and Traffic Cams Watch Now
New York City Congestion pricing plan FAQ
Congestion pricing tolls in New York City could begin as early as June, but no official date has been set

NEW YORK CITY (WABC) -- New York City could become the first U.S. city with congestion tolls on drivers as early as this summer.
Transit officials approved the congestion pricing plan that would charge a $15 fee for most drivers entering the busiest part of Manhattan, south of 60th Street during daytime hours.
When does congestion pricing start?
Congestion pricing tolls are set to begin early in the morning of June 30 .
How much will drivers be charged?
The final tolling structure is based on recommendations by the advisory panel:
Most passenger vehicles and passenger-type vehicles with commercial license plates would be charged a $15 toll during the peak period (5 a.m. to 9 p.m. on weekdays and 9 a.m. to 9 p.m. on weekends), and $3.75 overnight. Those vehicles would be charged only once a day.
Trucks and buses would be charged a $24 or $36 toll in the peak period, depending on their size and purpose. Overnight tolls would be $6 and $8
Motorcycles would be charged $7.50, no more than once per day
Passengers would be charged a toll of $1.25 per trip for taxis, green cabs, and for-hire vehicles, and $2.50 per trip for trips dispatched by high-volume for-hire services such as Uber and Lyft. There would be no nighttime discounts.
Qualifying authorized emergency vehicles, qualifying vehicles transporting a person with disabilities, specialized government vehicles, and transit and commuter buses would be exempted from the CBD toll
Low-income vehicle owners who qualify and register with MTA would receive a 50% discount on the peak auto toll beginning with the 11th trip taken in a calendar month
How do you pay the toll?
Customers can pay using E-ZPass or through Tolls by Mail, whereby an image is taken of their license plate and a bill is sent in the mail to the vehicle's registered owner
Visitors without E-ZPasses will pay more.
Taxis will charge passengers $1.25 per trip that touches the zone, while app-based rides will charge $2.50.
What are the congestion pricing hours?
Peak toll rates would apply from 5 a.m. to 9 p.m. on weekdays, and from 9 a.m. to 9 p.m. on weekends.
Toll rates would be 75% lower overnight The overnight period runs from 9 p.m. to 5 a.m. on weekdays, and from 9 p.m. to 9 a.m. on weekends.
Are there exemptions or discounts?
Some exceptions include:
Emergency vehicles, specialized city vehicles, and buses with regular public routes or city school contracts.
Vehicles carrying disabled people and certain low-income commuters.
Low-income drivers are eligible for discounts and tax credits.
The $1 billion in toll revenue mandated by the state law meant that eliminating charges for one group would increase the price for everyone else, so most requests for exceptions weren't granted.
Why congestion pricing?
Supporters of the new tolls say it will push more people to use public transport, reduce congestion to speed up public buses and emergency vehicles, reduce pollution, and raise money needed to improve the subway system.
After paying for the cost of the program, 80% of the money will be used to improve and modernize New York City Transit, which runs the subways and buses; 10% for Long Island Rail Road; and 10% for Metro-North Railroad
Who opposes congestion pricing?
A recent Siena College survey found that 64% of people in New York oppose the $15 toll to drive below 60th Street in Manhattan. Opponents say the fees are a burden on workers and will increase the prices of staple goods that are driven to the city by truck. New York's plan has drawn lawsuits from small business owners and the state of New Jersey which demand more thorough environmental assessments before the plan moves forward. In court documents, state officials argue the plan will cost its commuters millions but won't fund improvements in New Jersey transit agencies, even as it funds public transit in New York and Connecticut.
How did we get here?
The state Legislature approved the tolls in 2019, mandating that the program should raise $1 billion per year to fund public subway and bus systems for the city's 4 million daily riders. It also established the boundaries of the zone, which covers the busiest part of the city, and scaled back early proposals to include the area up to 86th Street. The pandemic and lack of federal regulation stalled the project.
If the plan survives legal challenges , New York City will become the first U.S. city to implement congestion pricing. Such schemes have been implemented in London, Stockholm, Milan and Singapore.
MORE: MTA officially approves congestion pricing tolling plan for NYC
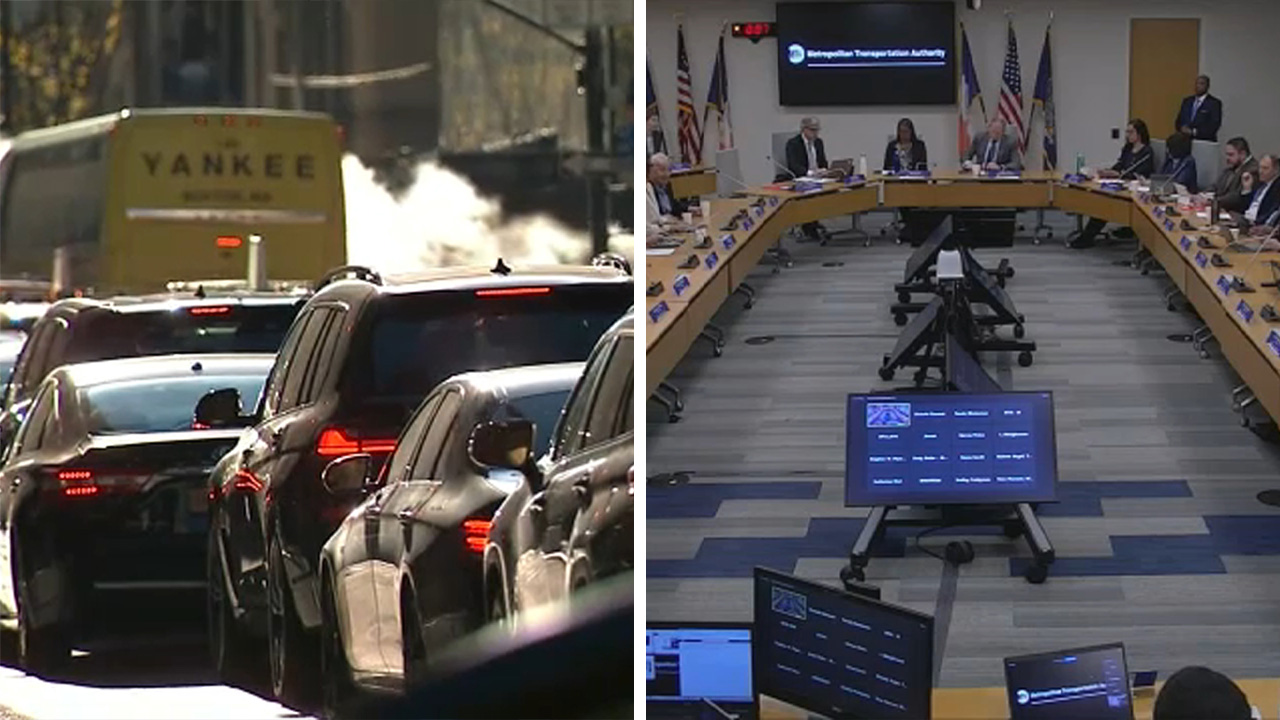
You can learn more about the plan on the MTA's congestion pricing program website .
Related Topics
- NEW YORK CITY
- CONGESTION PRICING
Top Stories
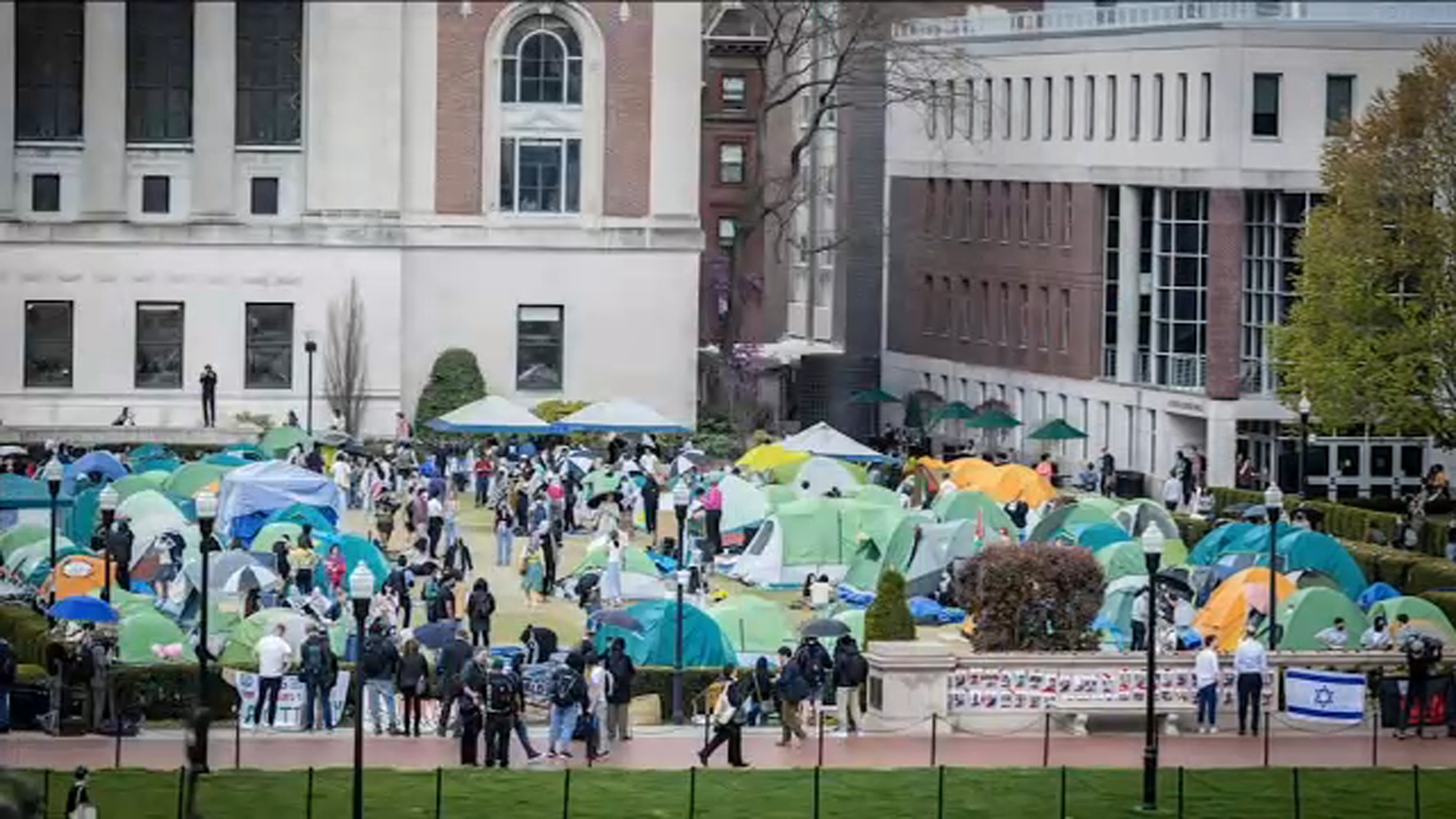
Columbia protesters told to leave encampment or face suspension
- 4 minutes ago
Queens DA clears out squatter house
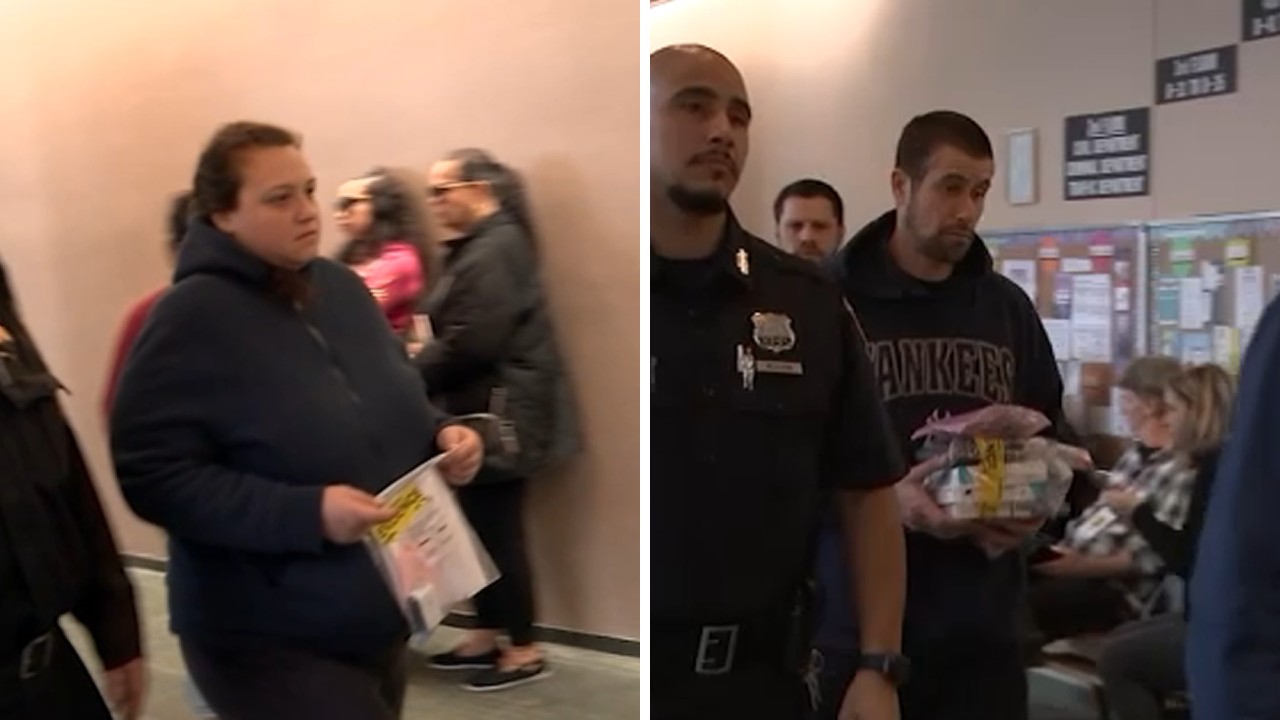
2 suspects in Long Island body parts case now charged with murder
- 3 hours ago
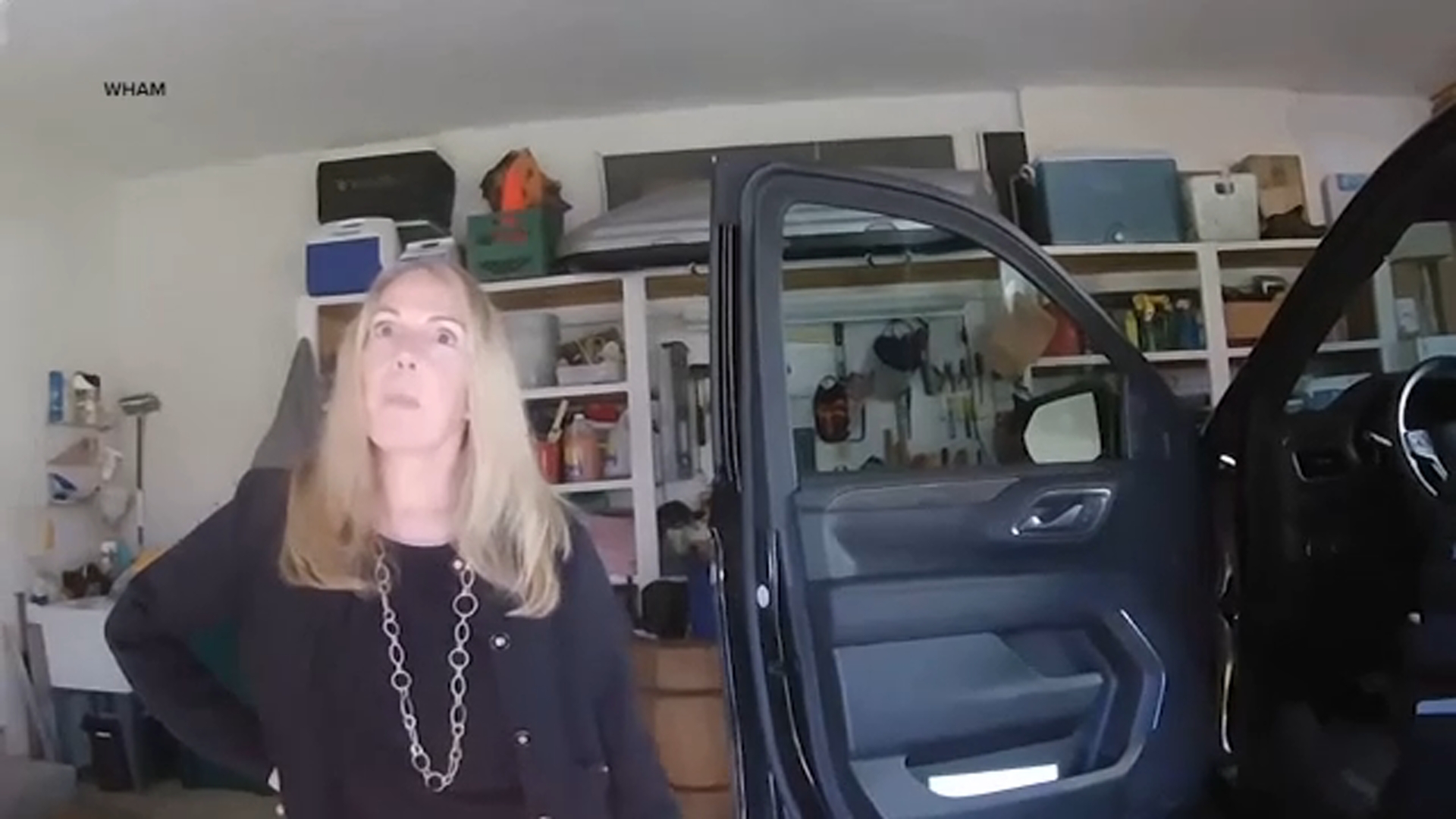
NY District Attorney accused of speeding refuses to pull over for cop
- 2 hours ago
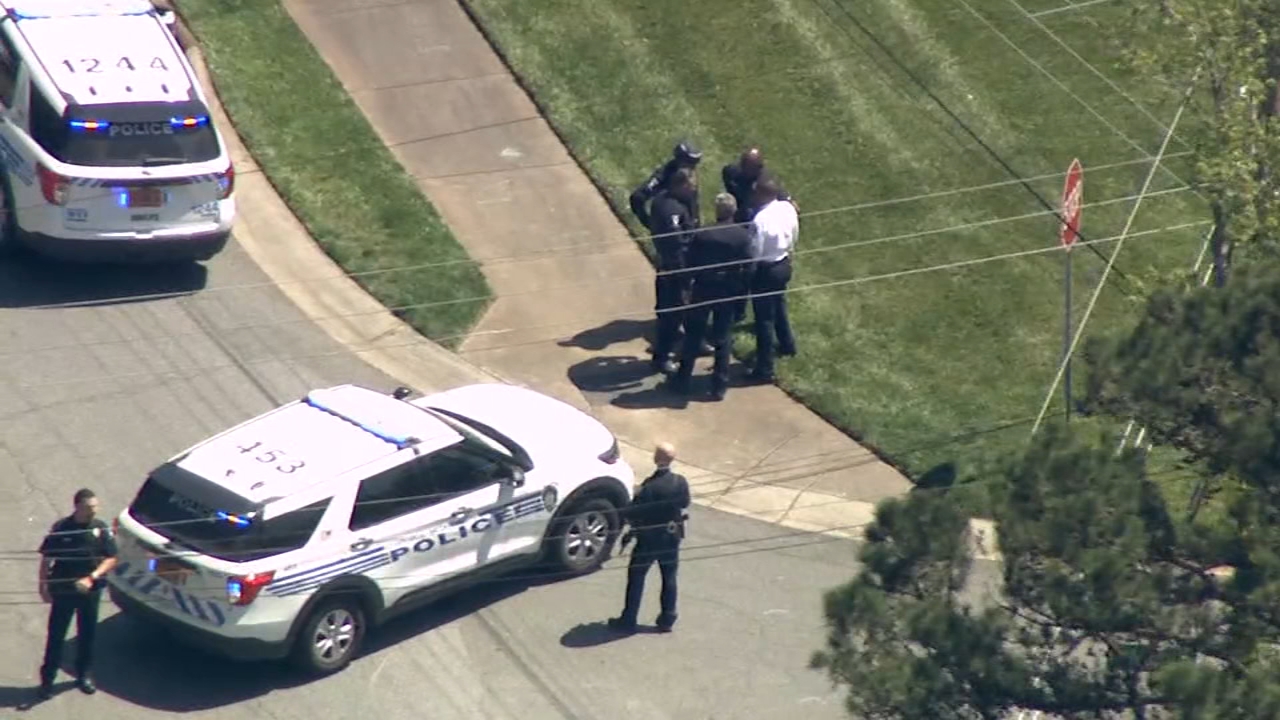
'Numerous' officers shot in Charlotte, police say
- 20 minutes ago
New proposed city law would track squatter problem
AccuWeather: Cooler on Tuesday, wet at night
- 25 minutes ago
Man killed in Hoboken's 1st homicide since 2022
Multi-source Data-driven Procedure for Traffic Analysis Zones Definition
Ieee account.
- Change Username/Password
- Update Address
Purchase Details
- Payment Options
- Order History
- View Purchased Documents
Profile Information
- Communications Preferences
- Profession and Education
- Technical Interests
- US & Canada: +1 800 678 4333
- Worldwide: +1 732 981 0060
- Contact & Support
- About IEEE Xplore
- Accessibility
- Terms of Use
- Nondiscrimination Policy
- Privacy & Opting Out of Cookies
A not-for-profit organization, IEEE is the world's largest technical professional organization dedicated to advancing technology for the benefit of humanity. © Copyright 2024 IEEE - All rights reserved. Use of this web site signifies your agreement to the terms and conditions.
- Share full article
Advertisement
Supported by
Congestion Pricing Will Start on June 30 in New York City, M.T.A. Says
Transit officials announced the start of the program, which they have said will ease some of the nation’s worst traffic.

By Ana Ley and Winnie Hu
The first comprehensive congestion-pricing program in a major U.S. city will begin in New York on June 30, the Metropolitan Transportation Authority announced on Friday.
The plan remains contentious, however, and with months to go before its scheduled rollout, legal and political clashes could still dilute or stop it altogether. A growing number of opponents — including Gov. Philip D. Murphy of New Jersey, influential unions and some elected New York officials — recently ratcheted up their effort to block it.
The program is meant to ease some of the worst traffic in the nation, improve the city’s air quality, increase mass transit ridership and raise money to modernize the city’s subway, bus and commuter rail system. Congestion-pricing tolls are expected to generate $1 billion a year, with the revenue earmarked by law for capital projects, not operating costs.
The authority, which operates the mass transit system, also said on Friday that it had opened a portal for applicants seeking the program’s coveted discounts and exemptions. A website will guide drivers on who qualifies and how to apply.
Under the program, most passenger cars will be charged $15 a day to enter a so-called congestion zone below 60th Street in Manhattan. Trucks would pay $24 or $36, depending on their size. Taxi fares would increase by $1.25, and Uber and Lyft fares would rise by $2.50.
The program is widely unpopular by many measures. A survey of about 800 New Yorkers conducted this month by Siena College found that 63 percent opposed it. Still, out of more than 25,000 public comments about congestion pricing submitted to the transportation authority from late December to mid-March, roughly 60 percent expressed support, the authority said.
M.T.A. and federal transportation officials are defending it against six lawsuits in federal courts in New York and New Jersey that seek to halt the plan. The courts, which are expected to rule before the program starts, could possibly delay or block it.
“They are proceeding at their own risk in arrogant disregard of pending court cases,” said Jack L. Lester, a lawyer for a group of city residents who filed one of the suits.
This month, a federal judge in Newark heard arguments in a suit brought by New Jersey officials who argue that the congestion-pricing plan would shift traffic and pollution to some communities in the state as drivers try to avoid the tolls.
“We are awaiting a court ruling as early as next month on whether the M.T.A.’s unprecedented congestion-pricing scheme can go forward,” said Randy Mastro, a lawyer for the state of New Jersey, adding that “the jury is still out on this hugely flawed plan.”
The tolling rates, which the authority’s board approved last month , are still being reviewed by the Federal Highway Administration, which has already signed off on an exhaustive study of the program and is expected to approve the tolls.
Congestion pricing is expected to lower the number of vehicles coming into the zone by about 17 percent, according to a November report by an authority advisory committee. The report also said the program would reduce the total number of miles driven by motorists in 28 counties in the metropolitan region.
The fees are also supposed to discourage drivers from jamming roads and idling in gridlock, which contributes to soot and other harmful air pollutants. But some of the program’s harshest critics have pointed to evidence that certain neighborhoods could end up with dirtier air as a result of the traffic that is diverted. In response, the authority has vowed to work to diminish such potential harm .
Other cities that have adopted congestion-pricing programs, such as London, Singapore and Stockholm , have reported sharp drops in traffic and congestion.
David I. Weprin, a Democratic state assemblyman from Queens and a longtime congestion-pricing opponent, said of the plan that the authority was “trying to aggressively ram it down the public’s throat.”
“I don’t think the public wants it and I think it’s really unfair for people who have to drive and have no choice,” Mr. Weprin said. He has joined a lawsuit filed by Vito Fossella, a Republican and Staten Island’s borough president, and the United Federation of Teachers that seeks to keep the tolling plan from proceeding.
Danny Pearlstein, a spokesman for the Riders Alliance, an advocacy group, said in a statement that the start of the program “cannot happen soon enough.”
Congestion pricing, he said, “will be a win-win-win for all New Yorkers, commuters and visitors and will bring better public transit, cleaner air, and freer moving traffic.”
Ana Ley is a Times reporter covering New York City’s mass transit system and the millions of passengers who use it. More about Ana Ley
Winnie Hu is a Times reporter covering the people and neighborhoods of New York City. More about Winnie Hu
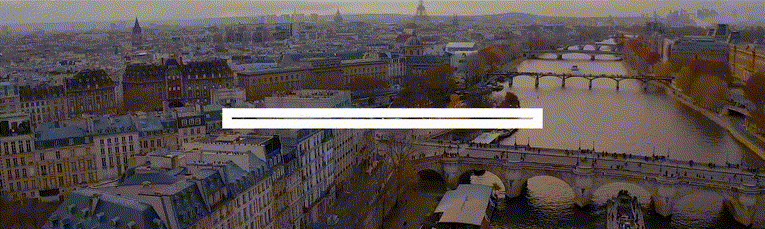
IMAGES
VIDEO
COMMENTS
Trip Generation is the first step in the conventional four-step transportation forecasting process (followed by Destination Choice, Mode Choice, and Route Choice), widely used for forecasting travel demands. It predicts the number of trips originating in or destined for a particular traffic analysis zone.
There are PWM modules, which allow generating PWM output signals. In older microcontrollers, there was a special protection mechanism, called "PDP". This one made output signals turn off if one of GPIOs read low signal. "PDP" means "Power Drive Protection" as far as I know. In new C2000 MCU PWM modules have another structure.
Trip Generation is the first step in the conventional four-step transportation forecasting process (followed by Destination Choice, Mode Choice, and Route Choice), widely used for forecasting travel demands.It predicts the number of trips originating in or destined for a particular traffic analysis zone. Every trip has two ends, and we need to know where both of them are. The first part is ...
Trip generation is the first step in the conventional four-step transportation forecasting process used for forecasting travel demands. It predicts the number of trips originating in or destined for a particular traffic analysis zone (TAZ). Trip generation analysis focuses on residences and residential trip generation is thought of as a function of the social and economic attributes of households.
Summary. Trip generation is traditionally the first step in 4-step travel models. Trip generation estimates for an individual traffic analysis zone (TAZs) the number of trip ends produced or attracted by that zone. Trip ends produced are called "trip productions", and trip ends attracted are called "trip attractions".
Pass-By and Diverted Number of Trips. Use either local data or ITE data to determine a percentage of the reduced trip generation that is pass-by or diverted. Similar to the ITE Trip Generation data, both pass-by and diverted trip percentages are available by average rate or an equation for many land uses. Use this percentage to calculate the ...
3.2.7.3 Operational Highlights for the Trip-Zone Submodule 3.2.9.2 Controlling and Monitoring the Digital Compare Submodule 3.2.9.3.1 Digital Compare Events Regarding TZCLR, If the DCEVT is active the flag will get set again. The source event has to go away for the flag to remain cleared.
Trip generation is estimated in three ways: (i) traditionally by linear and multiple regression. (ii) by aggregating the trip generating capability of a household or car and aggregating the total according to the distribution of each selected category in the zones, and. (iii) by household classification method through a catalogue of the ...
Although the definition of TAZs is one of the key stages in transportation planning studies, a proper approach for the definition is still one of the unsolved issues. In this research, a TAZ with regular geometric shapes, called regular traffic analysis zones (RTAZs), is presented as a new approach to traffic zoning.
Trip: A trip is defined as a one-way person movement by a mechanized mode of transport. A trip generally has two trip ends - an origin and a destination. Origin and Destination: Each trip starts at one location or zone and ends at another location or zone. The starting point or zone is called the "origin," and the ending point or zone is ...
Trip distribution. All trips have an origin and destination and these are considered at the trip distribution stage. Trip distribution (or destination choice or zonal interchange analysis) is the second component (after trip generation, but before mode choice and route assignment) in the traditional four-step transportation forecasting model.
Synonyms trip trip journey tour expedition excursion outing day out These are all words for an act of travelling to a place. trip an act of travelling from one place to another, and usually back again:. a business trip; a five-minute trip by taxi; journey an act of travelling from one place to another, especially when they are a long way apart:. a long and difficult journey across the mountains
An agency is an operator of a transit system, including both university and municipal public transportation agencies. See also: transit agency. agency-owned on-demand microtransit. On-demand microtransit services that are either completely delivered by or through the local transit agency on behalf of their city.
In trip-based models, TAZs are critical elements in the modeling process and are actually the spatial unit at which most calculations take place. Trip generation models predict the number of trips produced by or attracted to each zone, and trip distribution models (or destination choice models) link zonal trip productions and attractions. These ...
The trip distribution step (within trip-based models) determines where trips go, spatially. Trip distribution allocates trips to pairs of traffic analysis zones (TAZs), from the production (P) end to the attraction (A) end. The result is a PA table, which describes the numbers of person trips between zones (usually over a 24-hour period of time) but does not describe the actual direction of ...
the definition of zones with a very low number of trips or a very large area (high geographical error) is avoided; the density of trip production inside a zone should be as homogeneous as possible. Some of the constraints to the definition of TAZ presented in the preceding section were not included in this methodology.
trip: [verb] to catch the foot against something so as to stumble.
The goal of trip generation is to predict the number of trips, by purpose, that are generated by and attracted to each zone in a study area. Trip generation is performed by relating the number or frequency of trips to the characteristics of the individuals, of the zone, and of the transportation network. The zone that contains the home end of ...
A traffic analysis zone or transportation analysis zone (TAZ) is the unit of geography most commonly used in conventional transportation planning models. The size of a zone varies, but for a typical metropolitan planning software, a zone of under 3,000 people is common. The spatial extent of zones typically varies in models, ranging from very ...
The average distance ridden for an unlinked passenger trip (UPT) by time period (weekday, Saturday, Sunday) computed as passenger miles traveled (PMT) divided by unlinked passenger trips (UPT). ... (minimum average operating speed is defined as 45 mph in a 50-mh zone, or 10 mph below limit when limit is less than 50 mph). ... This definition ...
Passengers would be charged a toll of $1.25 per trip for taxis, green cabs, and for-hire vehicles, and $2.50 per trip for trips dispatched by high-volume for-hire services such as Uber and Lyft ...
The choice of an appropriate Traffic Analysis Zones (TAZ) system is a critical step of travel demand modeling which is often overlooked. Studies have approached this process as an optimization problem aiming to maximize the homogeneity of socio-economic and geographic characteristics of zones while minimizing, at the same time, the number of intrazonal trips. However, beyond the application of ...
Under the program, most passenger cars will be charged $15 a day to enter a so-called congestion zone below 60th Street in Manhattan. Trucks would pay $24 or $36, depending on their size.